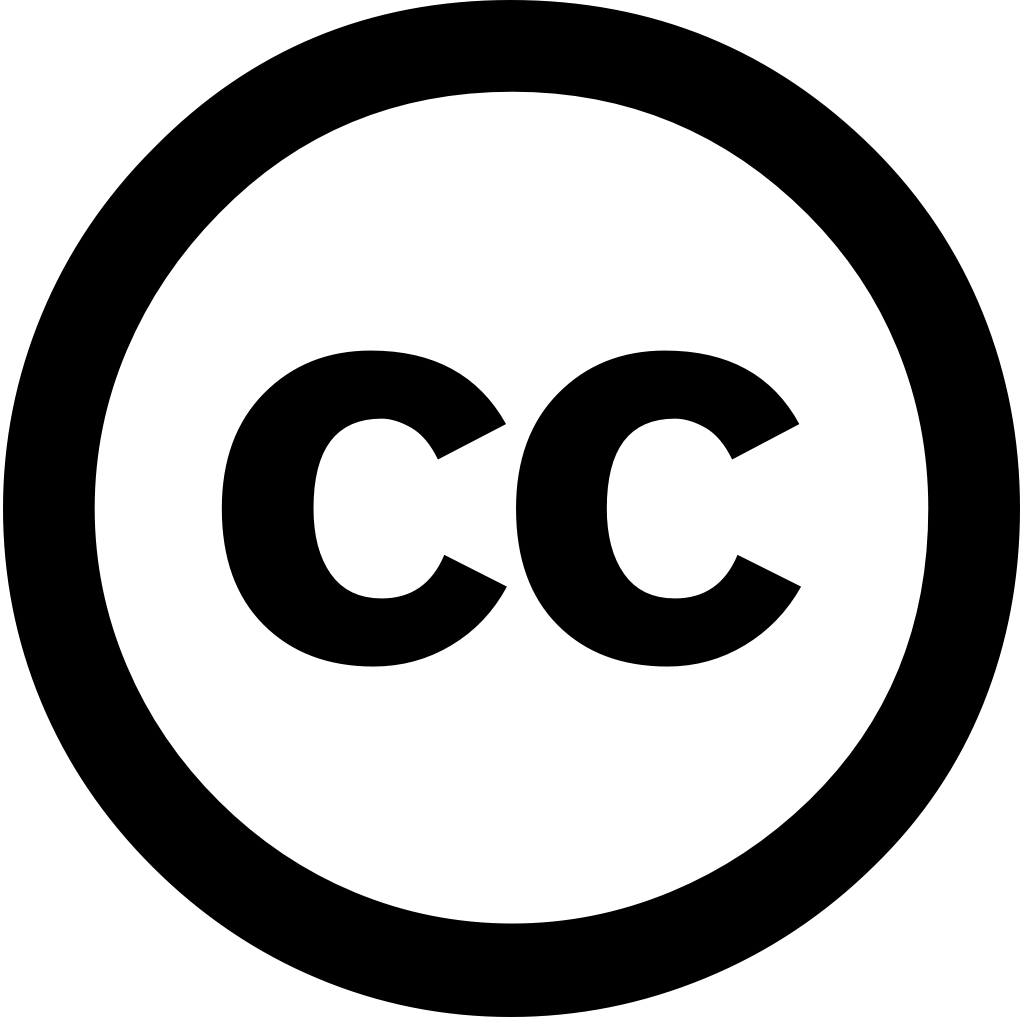
AgriEngineering, Journal Year: 2025, Volume and Issue: 7(4), P. 102 - 102
Published: April 3, 2025
Understanding the impact of robotization on workforce dynamics has become increasingly urgent. While expert assessments provide valuable insights, they are often time-consuming and resource-intensive. Large language models (LLMs) offer a scalable alternative; however, their accuracy reliability in evaluating potential remain uncertain. This study systematically compares general-purpose LLM-generated with evaluations to assess effectiveness agricultural sector by considering human judgments as ground truth. Using ChatGPT, Copilot, Gemini, LLMs followed three-step evaluation process focusing (a) task importance, (b) for robotization, (c) attribute indexing 15 occupations, mirroring methodology used assessors. The findings indicate significant tendency overestimate potential, most errors falling within range 0.229 ± 0.174. can be attributed primarily LLM reliance grey literature idealized technological scenarios, well limited capacity, account complexities work. Future research should focus integrating knowledge into training improving bias detection mitigation datasets, expanding studied enhance assessment reliability.
Language: Английский