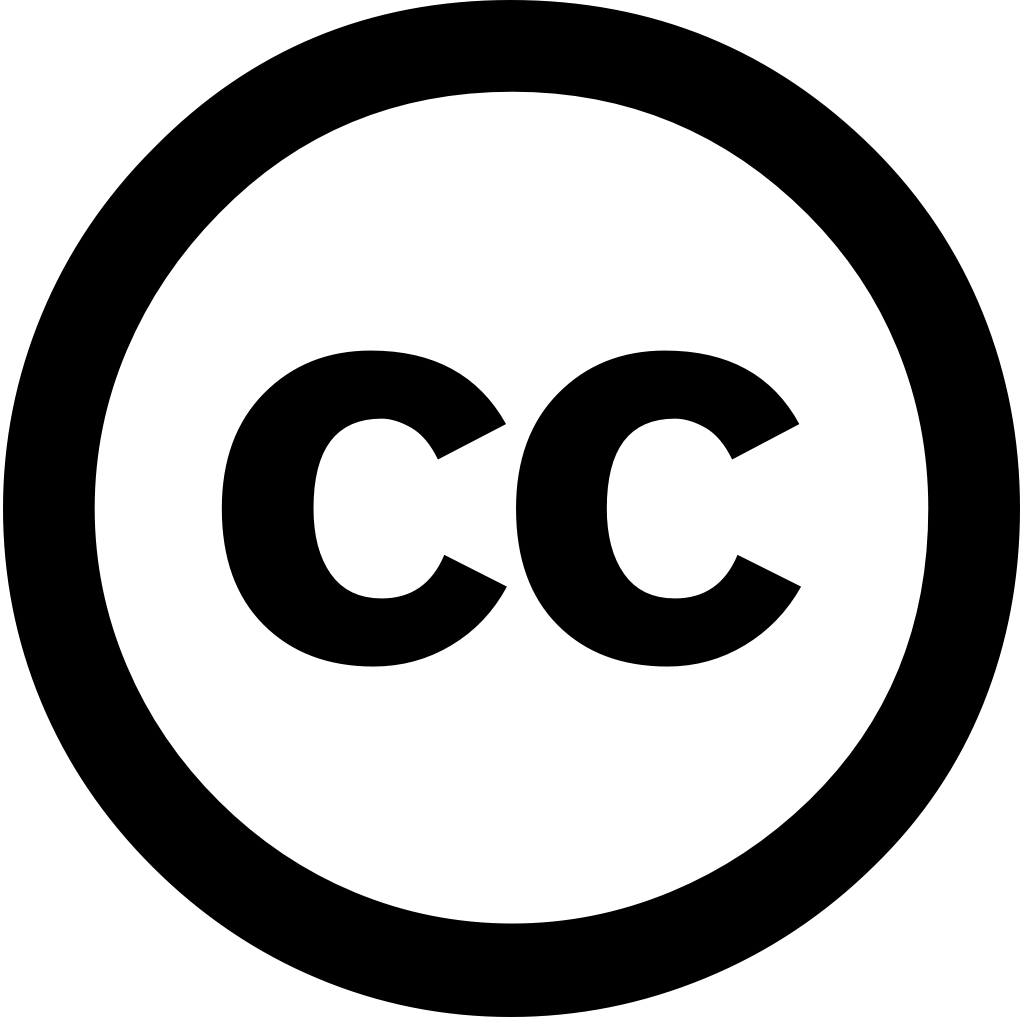
Case Studies in Thermal Engineering, Journal Year: 2024, Volume and Issue: unknown, P. 105627 - 105627
Published: Dec. 1, 2024
Language: Английский
Case Studies in Thermal Engineering, Journal Year: 2024, Volume and Issue: unknown, P. 105627 - 105627
Published: Dec. 1, 2024
Language: Английский
Results in Engineering, Journal Year: 2025, Volume and Issue: unknown, P. 103991 - 103991
Published: Jan. 1, 2025
Language: Английский
Citations
3Applied Sciences, Journal Year: 2025, Volume and Issue: 15(7), P. 3758 - 3758
Published: March 29, 2025
Wind energy represents a solution for reducing environmental impact. For this reason, research studies the elements that propose optimizing wind production through intelligent solutions. Although there are address optimization of turbine performance or other indirectly related factors in production, remains topic insufficiently explored and synthesized literature. This how machine learning (ML) techniques can be applied to optimize production. aims study systematic applications ML identify analyze key stages optimized Through research, case highlighted by which methods proposed directly target issue power process turbines. From total 1049 articles obtained from Web Science database, most studied models context artificial neural networks, with 478 papers identified. Additionally, literature identifies 224 have random forest 114 incorporated gradient boosting about power. Among these, 60 specifically addressed aspect allows identification gaps The notes previous focused on forecasting, fault detection, efficiency. existing addresses indirect component performance. Thus, paper current discusses algorithms processes, future directions increasing efficiency turbines integrated predictive methods.
Language: Английский
Citations
1Journal of Infrastructure Policy and Development, Journal Year: 2025, Volume and Issue: 9(1), P. 10072 - 10072
Published: Jan. 6, 2025
This study meticulously explores the crucial elements precipitating corporate failures in Taiwan during decade from 1999 to 2009. It proposes a new methodology, combining ANOVA and tuning parameters of classification so that its functional form describes data best. Our analysis reveals ten paramount factors, including Return on Capital ROA(C) before interest depreciation, debt ratio percentage, consistent EPS across last four seasons, Retained Earnings Total Assets, Working dependency borrowing, Current Liability Net Value Per Share (B), Equity, Liability-Assets Flag. dual approach enables more precise identification most instrumental variables leading Taiwanese firms bankruptcy based only financial rather than governance variable. By employing methodology adept at addressing class imbalance, we substantiate significant influence these factors had incidence among companies rely solely parameters. Thus, our streamlines variable selection 95 10 critical improving prediction accuracy outperforming Liang’s 2016 results.
Language: Английский
Citations
0Applied System Innovation, Journal Year: 2025, Volume and Issue: 8(1), P. 12 - 12
Published: Jan. 16, 2025
Wind turbine blade structural dynamics are crucial in the design phase. Blade deflections and loads can affect weight of rotor as well power performance a wind if extremely high. Predictions turbine’s lead to informative decisions on optimizing blade. In this work, multivariate machine learning (ML) approach is used predict blade’s based flow conditions control actions turbine. Three different datasets were generated using OpenFAST software tool for three turbulence classes. Various ML algorithms trained at tip root edgewise flapwise directions. The models tested generalization model conditions. A one dataset with classes then outputs other two datasets. random forest algorithm gave best accuracy predicting it was for, predictions found be higher direction both load deflection outputs. direction, could data an around 99% over 75%. While only prediction above 95% all
Language: Английский
Citations
0Energy Reports, Journal Year: 2025, Volume and Issue: 13, P. 4318 - 4330
Published: April 9, 2025
Language: Английский
Citations
0Case Studies in Thermal Engineering, Journal Year: 2024, Volume and Issue: unknown, P. 105627 - 105627
Published: Dec. 1, 2024
Language: Английский
Citations
1