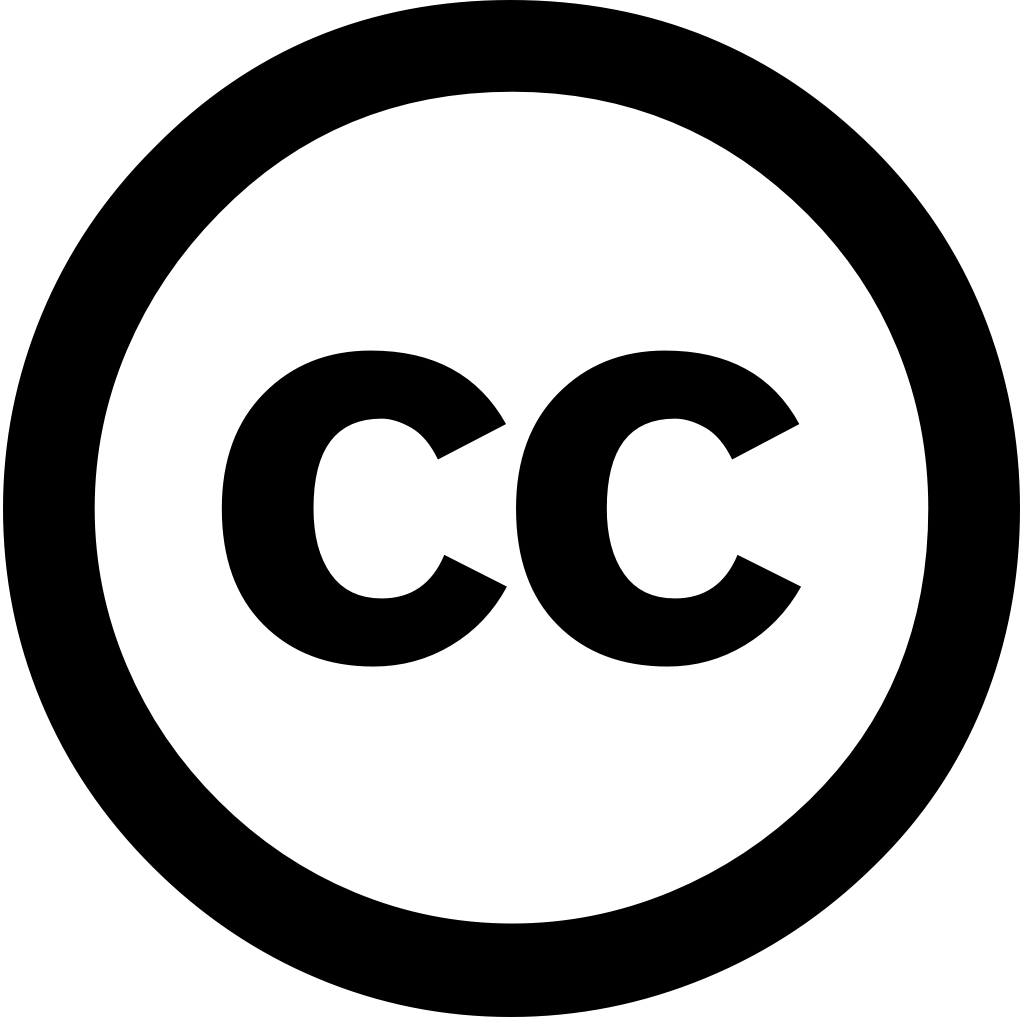
Big Data and Cognitive Computing, Journal Year: 2025, Volume and Issue: 9(5), P. 114 - 114
Published: April 27, 2025
As AI becomes indispensable in healthcare, its vulnerability to adversarial attacks demands serious attention. Even minimal changes the input data can mislead Deep Learning (DL) models, leading critical errors diagnosis and endangering patient safety. In this study, we developed an optimized Multi-layer Perceptron (MLP) model for breast cancer classification exposed cybersecurity vulnerabilities through a real-world-inspired attack. Unlike prior studies, conducted quantitative evaluation on impact of Fast Gradient Sign Method (FGSM) attack DL designed detection demonstrate how minor perturbations reduced model’s accuracy from 98% 53%, led substantial increase errors, as revealed by confusion matrix. Our findings significantly compromise performance healthcare model, underscoring importance aligning development with readiness. This research highlights demand designing resilient integrating rigorous practices at every stage lifecycle, i.e., before, during, after engineering prioritize effectiveness, accuracy, safety real-world environments.
Language: Английский