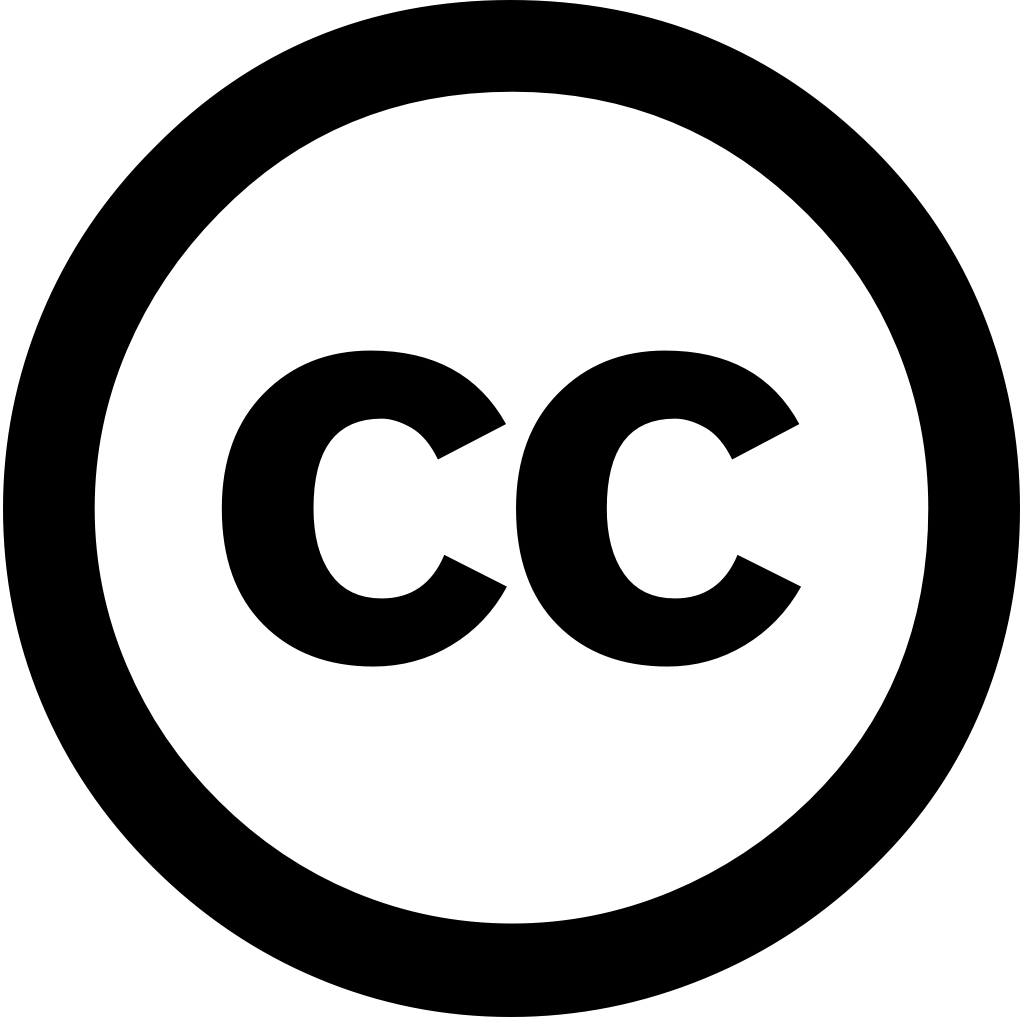
Energy and Buildings, Journal Year: 2025, Volume and Issue: unknown, P. 115665 - 115665
Published: April 1, 2025
Language: Английский
Energy and Buildings, Journal Year: 2025, Volume and Issue: unknown, P. 115665 - 115665
Published: April 1, 2025
Language: Английский
Energies, Journal Year: 2025, Volume and Issue: 18(6), P. 1408 - 1408
Published: March 12, 2025
Building HVAC systems face significant challenges in energy optimization due to changing building characteristics and the need balance multiple efficiency objectives. Current approaches are limited: physics-based models expensive inflexible, while data-driven methods require extensive data collection ongoing maintenance. This paper introduces a systematic relearning framework for supervisory control that improves adaptability reducing operational costs. Our approach features Reinforcement Learning controller with self-monitoring adaptation capabilities responds effectively changes operations environmental conditions. We simplify complex hyperparameter process through structured decomposition method implement strategy handle over time. demonstrate our framework’s effectiveness comprehensive testing on testbed, comparing performance against established methods.
Language: Английский
Citations
0Energy and Buildings, Journal Year: 2025, Volume and Issue: unknown, P. 115665 - 115665
Published: April 1, 2025
Language: Английский
Citations
0