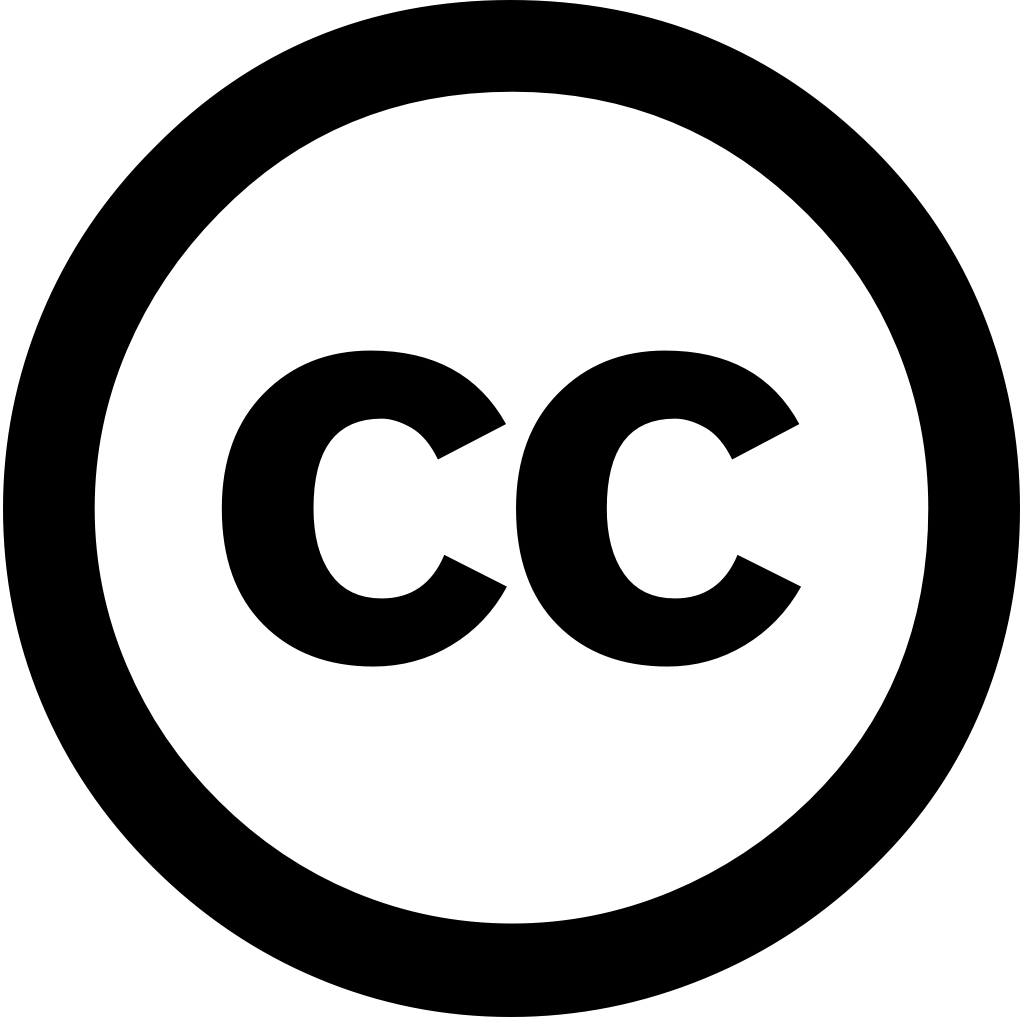
Scientific Reports, Journal Year: 2025, Volume and Issue: 15(1)
Published: April 1, 2025
This study investigates the integration of Robust Model Predictive Control (RMPC) and Deep Learning to enhance performance adaptability energy systems, focusing on Combined Heat Power (CHP), Power-to-Hydrogen, Power-to-Gas Methane applications. The proposed framework combines RMPC's robustness with Learning's ability learn adapt, improving control precision operational efficiency. Extensive simulations indicate that integrated RMPC-Deep system improves accuracy by 8.02% compared conventional methods, while also reducing consumption 12.14%. These quantitative results demonstrate effectiveness in addressing challenges such as operator saturation, showcasing its potential optimize systems under dynamic conditions. work highlights transformative role merging RMPC Learning, providing a robust adaptable solution for management complex
Language: Английский