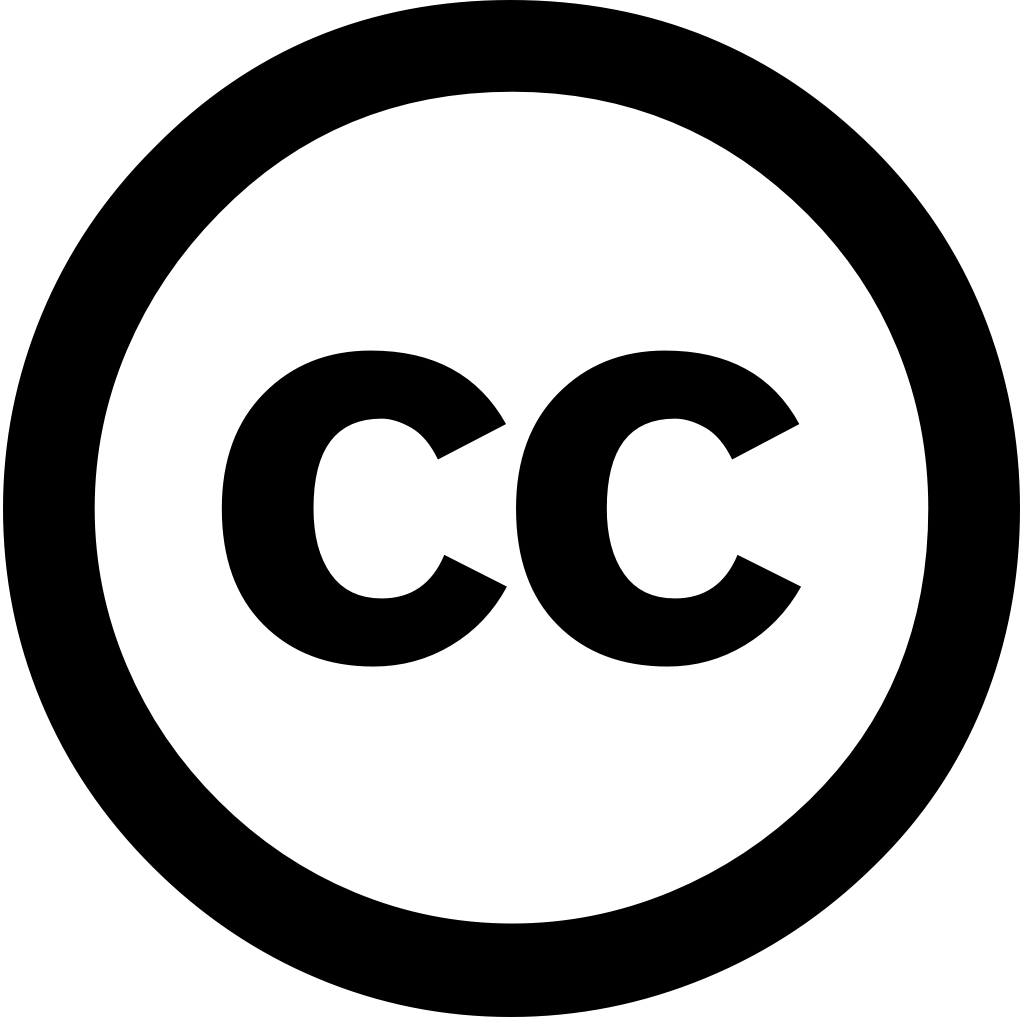
Mathematics, Journal Year: 2025, Volume and Issue: 13(8), P. 1346 - 1346
Published: April 20, 2025
The analysis of commodity markets—particularly in the energy and metals sectors—is essential for understanding economic dynamics guiding decision-making. Financial uncertainty indices provide valuable insights that help reduce price uncertainty. This study employs wavelet analyses energy-based measures to investigate relationship between these prices across multiple time scales. approach captures complex, time-varying dependencies, offering a more nuanced how influence fluctuations. By integrating this with predictability measures, we assess enhance forecasting accuracy. We further incorporate deep learning models capable capturing sequential patterns financial series into our better evaluate their predictive potential. Our findings highlight varying impact on prices, showing while some offer information, others display strong correlations without significant power. These results underscore need tailored models, as different commodities react differently same conditions. combining wavelet-based machine techniques, presents comprehensive framework evaluating role markets. gained can support investors, policymakers, market analysts making informed decisions.
Language: Английский