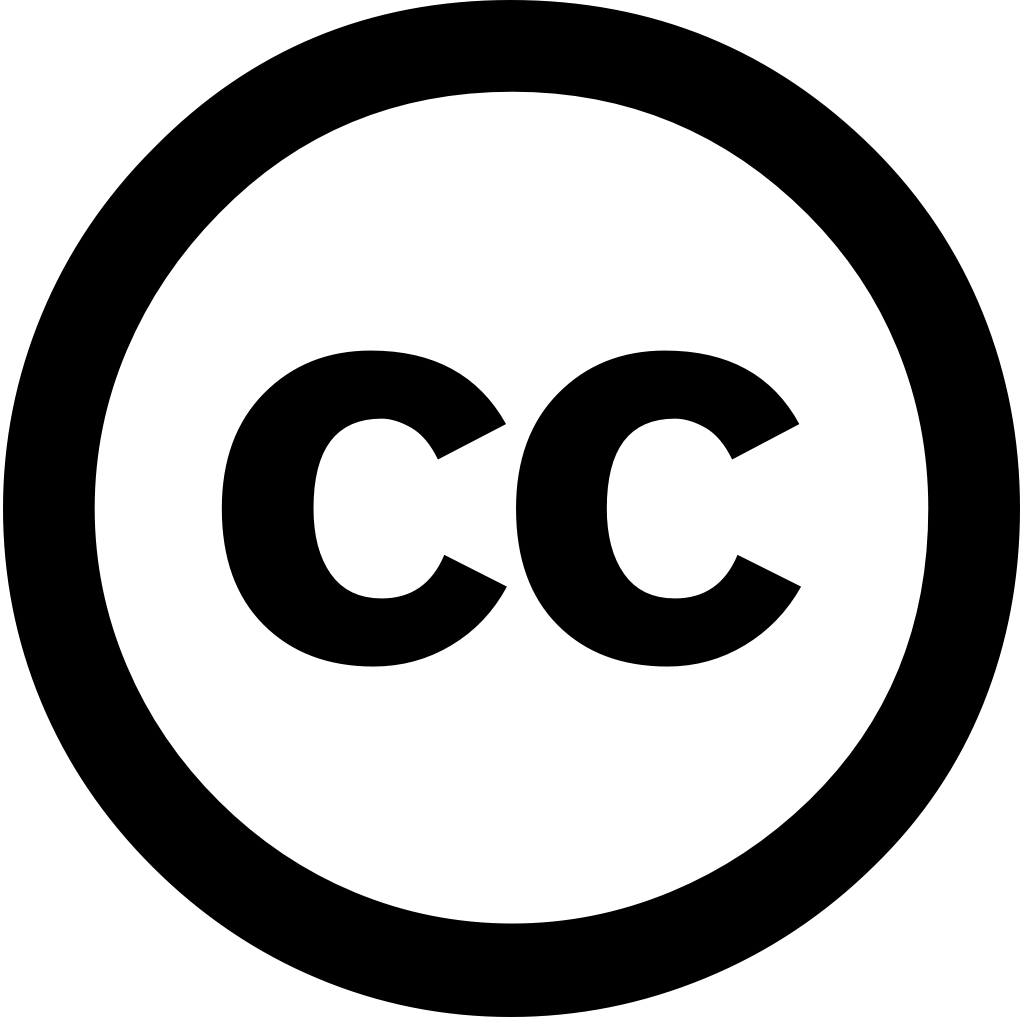
Frontiers in Energy Research, Journal Year: 2024, Volume and Issue: 12
Published: Oct. 18, 2024
The widespread adoption of nonlinear power electronic devices in residential settings has significantly increased the stochasticity and uncertainty systems. original load data, characterized by numerous irregular, random, probabilistic components, adversely impacts predictive performance deep learning techniques, particularly neural networks. To address this challenge, paper proposes a time-series prediction technique based on mature network point technique, i.e., decomposing data into deterministic stochastic components. component is predicted using technology, fitted with Gaussian mixture distribution model parameters are great expectation algorithm, after which obtained generation method. Using study evaluates six different methods to forecast power. By comparing errors these methods, optimal identified, leading substantial improvement accuracy.
Language: Английский