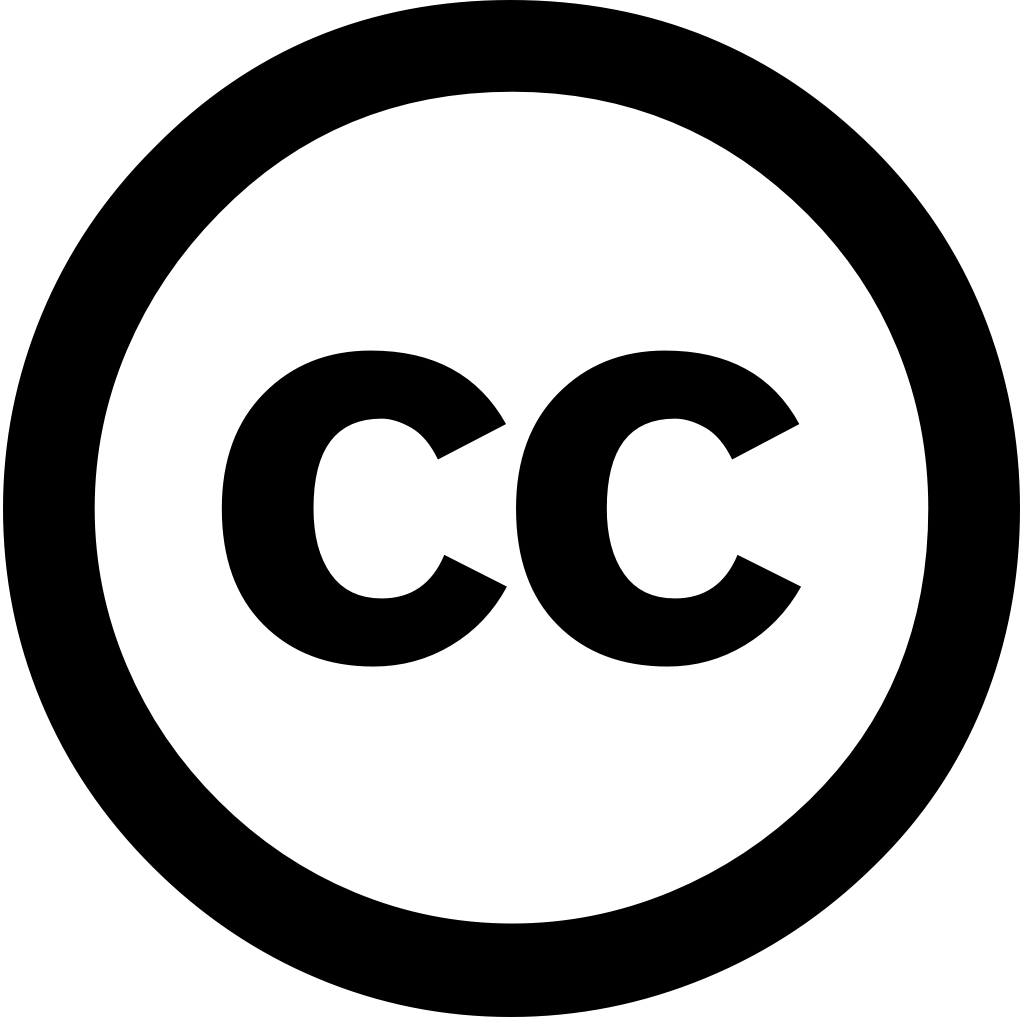
Intelligent Systems with Applications, Journal Year: 2025, Volume and Issue: unknown, P. 200529 - 200529
Published: April 1, 2025
Language: Английский
Intelligent Systems with Applications, Journal Year: 2025, Volume and Issue: unknown, P. 200529 - 200529
Published: April 1, 2025
Language: Английский
ACS Applied Electronic Materials, Journal Year: 2024, Volume and Issue: 7(1), P. 1 - 12
Published: Dec. 23, 2024
The liquid–liquid triboelectric nanogenerator (L-L TENG) is an emerging nanogeneration technology that converts weak mechanical energy, tidal and other forms of energy into electricity through the frictional interactions between liquids. This paper reviews research progress L-L TENG. First, it provides overview working principles TENG, analyzes its basic mechanisms, summarizes fundamental operation modes while organizing materials currently used for charge transfer. Additionally, this outlines applications TENG in harvesting, medicine, fields, offering insights performance enhancement expansion application scenarios. Finally, discusses challenges facing development as well future direction potential applications. Overall, conversion technology, has attracted widespread interest from scientists worldwide. review aims to provide scientists, engineers, researchers related fields with a comprehensive perspective further advance area research.
Language: Английский
Citations
21Protection and Control of Modern Power Systems, Journal Year: 2024, Volume and Issue: 9(6), P. 1 - 18
Published: Nov. 1, 2024
Supercapacitors (SCs) are widely recognized as excellent clean energy storage devices. Accurate state of health (SOH) estimation and remaining useful life (RUL) prediction essential for ensuring their safe reliable operation. This paper introduces a novel method SOH RUL prediction, based on hybrid neural network optimized by an improved honey badger algorithm (HBA). The combines the advantages convolutional (CNN) bidirectional long-short-term memory (BiLSTM) network. HBA optimizes hyperparameters CNN automatically extracts deep features from time series data reduces dimensionality, which then used input BiLSTM. Additionally, recurrent dropout is introduced in layer to reduce overfitting facilitate learning process. approach not only improves accuracy estimates forecasts but also significantly processing time. SCs under different working conditions validate proposed method. results show that model effectively features, enriches local details, enhances global perception capabilities. outperforms single models, reducing root mean square error below 1%, offers higher robustness compared other methods.
Language: Английский
Citations
11Scientific Reports, Journal Year: 2024, Volume and Issue: 14(1)
Published: Oct. 9, 2024
Accurate runoff forecasting is of great significance for water resource allocation flood control and disaster reduction. However, due to the inherent strong randomness sequences, this task faces significant challenges. To address challenge, study proposes a new SMGformer forecast model. The model integrates Seasonal Trend decomposition using Loess (STL), Informer's Encoder layer, Bidirectional Gated Recurrent Unit (BiGRU), Multi-head self-attention (MHSA). Firstly, in response nonlinear non-stationary characteristics sequence, STL used extract sequence's trend, period, residual terms, multi-feature set based on 'sequence-sequence' constructed as input model, providing foundation subsequent models capture evolution runoff. key features are then captured layer. Next, BiGRU layer learn temporal information these features. further optimize output MHSA mechanism introduced emphasize impact important information. Finally, accurate achieved by transforming through Fully connected verify effectiveness proposed monthly data from two hydrological stations China selected, eight compare performance results show that compared with Informer 1th step MAE decreases 42.2% 36.6%, respectively; RMSE 37.9% 43.6% NSE increases 0.936 0.975 0.487 0.837, respectively. In addition, KGE at 3th 0.960 0.805, both which can maintain above 0.8. Therefore, accurately sequence extend effective period
Language: Английский
Citations
9Ionics, Journal Year: 2025, Volume and Issue: 31(3), P. 2337 - 2349
Published: Jan. 14, 2025
Language: Английский
Citations
1Energy, Journal Year: 2025, Volume and Issue: unknown, P. 134545 - 134545
Published: Jan. 1, 2025
Language: Английский
Citations
1Energy, Journal Year: 2025, Volume and Issue: unknown, P. 134792 - 134792
Published: Jan. 1, 2025
Language: Английский
Citations
1Journal of Power Sources, Journal Year: 2025, Volume and Issue: 633, P. 236414 - 236414
Published: Feb. 7, 2025
Language: Английский
Citations
1Applied Energy, Journal Year: 2025, Volume and Issue: 386, P. 125525 - 125525
Published: Feb. 20, 2025
Language: Английский
Citations
1Ionics, Journal Year: 2024, Volume and Issue: unknown
Published: Oct. 5, 2024
Language: Английский
Citations
8International Journal of Electrochemical Science, Journal Year: 2024, Volume and Issue: 19(10), P. 100793 - 100793
Published: Sept. 11, 2024
Language: Английский
Citations
5