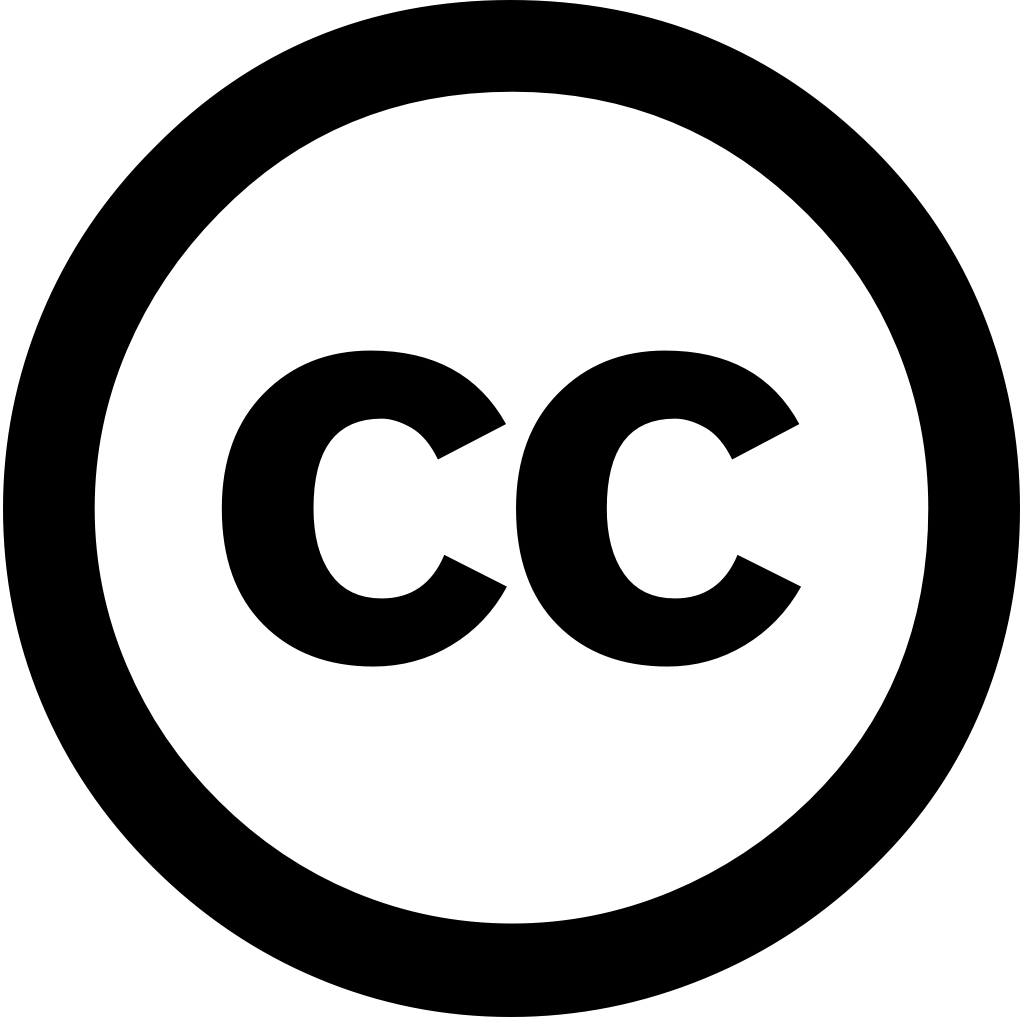
Scientific Reports, Journal Year: 2024, Volume and Issue: 14(1)
Published: Dec. 30, 2024
Accurate estimation of the soil resilient modulus (M
Language: Английский
Scientific Reports, Journal Year: 2024, Volume and Issue: 14(1)
Published: Dec. 30, 2024
Accurate estimation of the soil resilient modulus (M
Language: Английский
Materials, Journal Year: 2024, Volume and Issue: 17(7), P. 1452 - 1452
Published: March 22, 2024
This study optimized friction stir welding (FSW) parameters for 1.6 mm thick 2024T3 aluminum alloy sheets. A 3 × factorial design was employed to explore tool rotation speeds (1100 1300 rpm) and (140 180 mm/min). Static tensile tests revealed the joints' maximum strength at 87% relative base material. Hyperparameter optimization conducted machine learning (ML) models, including random forest XGBoost, multilayer perceptron artificial neural network (MLP-ANN) using grid search. Welding parameter extrapolation were then carried out, with final predictions analyzed response surface methodology (RSM). The ML models achieved over 98% accuracy in regression, demonstrating significant effectiveness FSW process enhancement. Experimentally validated, resulted an joint efficiency of 93% outcome highlights critical role advanced analytical techniques improving quality efficiency.
Language: Английский
Citations
14International Journal of Heat and Mass Transfer, Journal Year: 2024, Volume and Issue: 236, P. 126272 - 126272
Published: Oct. 15, 2024
Language: Английский
Citations
4Energies, Journal Year: 2025, Volume and Issue: 18(3), P. 511 - 511
Published: Jan. 23, 2025
Modeling of thermal energy storage (TES) tanks with computational fluid dynamics (CFD) tools exhibits limitations that hinder the time, scalability, and standardization procedure. In this study, an innovative technique is proposed to overcome challenges in CFD modeling TES tanks. This study assessed feasibility employing neural networks for tank modeling, evaluating similarities terms structure signal-to-noise ratio by comparing images generated those produced through simulations. The results regarding structural similarity index indicate around 94% obtained have a above 0.9. For ratio, mean value 25 dB, which can be considered acceptable, although indicating room improvement. Additional show our network model obtains best performance when working initial states close stable phase tank. are promising, laying groundwork future pathway could potentially replace current methods used modeling.
Language: Английский
Citations
0Micromachines, Journal Year: 2025, Volume and Issue: 16(3), P. 350 - 350
Published: March 19, 2025
Thermal analysis is an indispensable aspect of semiconductor packaging. Excessive operating temperatures in integrated circuit (IC) packages can degrade component performance and even cause failure. Therefore, thermal resistance characteristics are critical to the reliability electronic components. Machine learning modeling offers effective way predict IC packages. In this study, data from finite element (FEA) utilized by machine models during package testing. For two types, namely Quad Flat No-lead (QFN) Thin Fine-pitch Ball Grid Array (TFBGA), derived analysis, employed resistance. The values include θJA, θJB, θJC, ΨJT, ΨJB. Five models, light gradient boosting (LGBM), random forest (RF), XGBoost (XGB), support vector regression (SVR), multilayer perceptron (MLP), applied as forecasting study. Numerical results indicate that model outperforms other terms accuracy for almost all cases. Furthermore, achieved highly satisfactory. conclusion, shows significant promise a reliable tool predicting packaging design. application techniques these parameters could enhance efficiency designs.
Language: Английский
Citations
0International Communications in Heat and Mass Transfer, Journal Year: 2025, Volume and Issue: 164, P. 108921 - 108921
Published: April 3, 2025
Language: Английский
Citations
0Materials Science and Engineering A, Journal Year: 2025, Volume and Issue: unknown, P. 148338 - 148338
Published: April 1, 2025
Language: Английский
Citations
0Energy, Journal Year: 2025, Volume and Issue: unknown, P. 136185 - 136185
Published: April 1, 2025
Language: Английский
Citations
0Thermal Science and Engineering Progress, Journal Year: 2024, Volume and Issue: 50, P. 102577 - 102577
Published: April 6, 2024
Language: Английский
Citations
1Cogent Economics & Finance, Journal Year: 2024, Volume and Issue: 12(1)
Published: Nov. 8, 2024
Accurate Gross Domestic Product (GDP) prediction is essential for economic planning and policy formulation. This paper evaluates the performance of three machine learning models—Random Forest Regression (RFR), XGBoost, Prophet—in predicting Somalia's GDP. Historical data, including GDP per capita, population, inflation rate, current account balances, were used in training testing. Among models, RFR achieved best accuracy with lowest MAE (0.6621%), MSE (1.3220%), RMSE (1.1497%), R-squared 0.89. The Diebold-Mariano p-value (0.042) confirmed its higher predictive accuracy. XGBoost performed well but slightly error, yielding an 0.85 0.063. In contrast, Prophet had highest forecast errors, 0.78 0.015. For enhanced interpretability, SHapley Additive exPlanations (SHAP) applied to RFR, identifying lagged balance, population as key predictors, along total government net lending/borrowing. SHAP plots provided insights into these features' contributions predictions. study highlights RFR's effectiveness forecasting emphasizes importance indicators.
Language: Английский
Citations
1Systems, Journal Year: 2024, Volume and Issue: 12(12), P. 528 - 528
Published: Nov. 27, 2024
The reduction of greenhouse gas emissions, in order to effectively address the issue climate change, has critical importance worldwide. To achieve this aim and implement necessary strategies policies, projection emissions is essential. This paper presents a forecasting framework for based on advanced machine learning algorithms: multivariable linear regression, random forest, k-nearest neighbor, extreme gradient boosting, support vector, multilayer perceptron regression algorithms. algorithms employ several input variables associated with emission outputs. In evaluate applicability performance developed framework, nationwide statistical data from Turkey are employed as case study. dataset study includes six annual sectoral total CO2 eq. output variables. provides scenario-based approach future forecasts sector-based analysis country considering multiple present indicates that stated can be successfully applied emissions.
Language: Английский
Citations
1