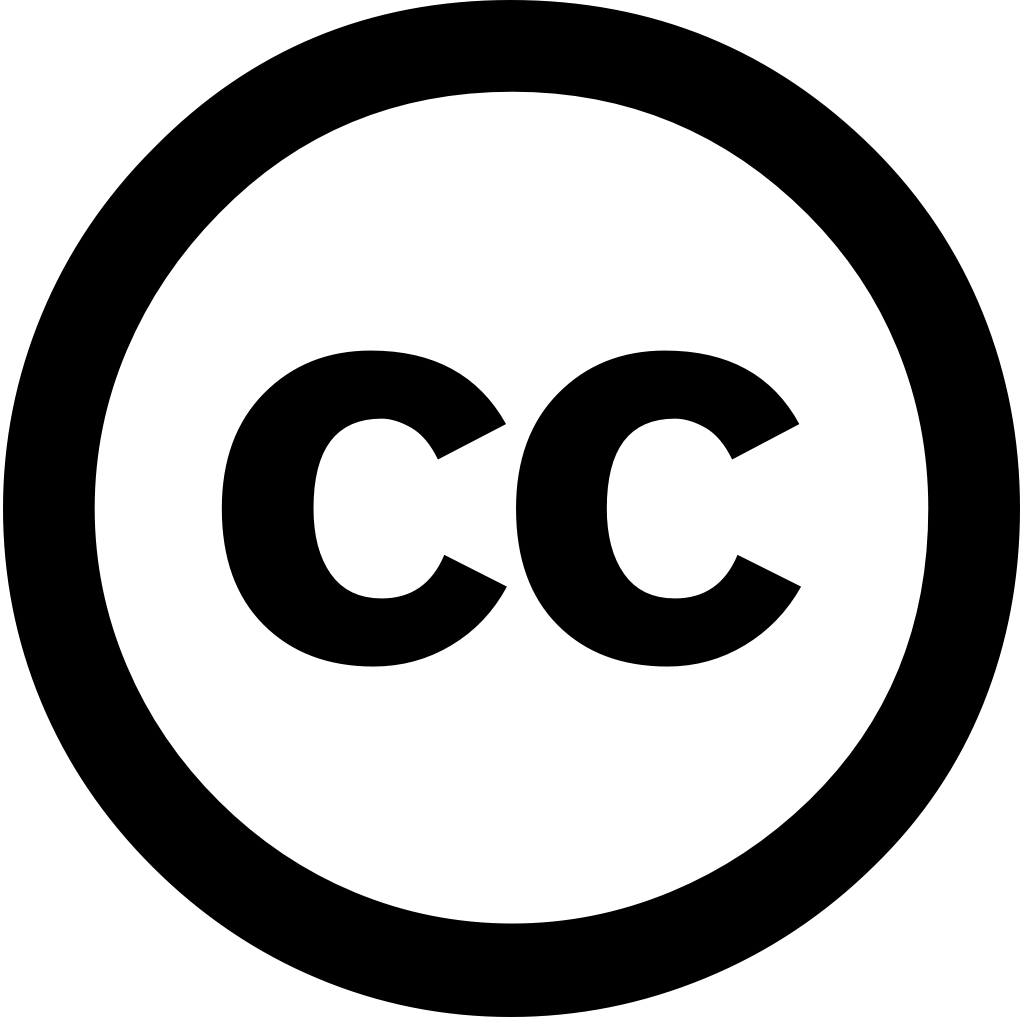
Computers in Biology and Medicine, Journal Year: 2024, Volume and Issue: 185, P. 109492 - 109492
Published: Dec. 5, 2024
Language: Английский
Computers in Biology and Medicine, Journal Year: 2024, Volume and Issue: 185, P. 109492 - 109492
Published: Dec. 5, 2024
Language: Английский
PLoS ONE, Journal Year: 2024, Volume and Issue: 19(3), P. e0297667 - e0297667
Published: March 20, 2024
Skin cancer is a common affecting millions of people annually. cells inside the body that grow in unusual patterns are sign this invasive disease. The then spread to other organs and tissues through lymph nodes destroy them. Lifestyle changes increased solar exposure contribute rise incidence skin cancer. Early identification staging essential due high mortality rate associated with In study, we presented deep learning-based method named DVFNet for detection from dermoscopy images. To detect images pre-processed using anisotropic diffusion methods remove artifacts noise which enhances quality A combination VGG19 architecture Histogram Oriented Gradients (HOG) used research discriminative feature extraction. SMOTE Tomek resolve problem imbalanced multiple classes publicly available ISIC 2019 dataset. This study utilizes segmentation pinpoint areas significantly damaged cells. vector map created by combining features HOG VGG19. Multiclassification accomplished CNN maps. achieves an accuracy 98.32% on Analysis variance (ANOVA) statistical test validate model's accuracy. Healthcare experts utilize model at early clinical stage.
Language: Английский
Citations
21Computers in Biology and Medicine, Journal Year: 2024, Volume and Issue: 177, P. 108635 - 108635
Published: May 22, 2024
Multimodal medical imaging plays a pivotal role in clinical diagnosis and research, as it combines information from various modalities to provide more comprehensive understanding of the underlying pathology. Recently, deep learning-based multimodal fusion techniques have emerged powerful tools for improving image classification. This review offers thorough analysis developments classification tasks. We explore complementary relationships among prevalent outline three main schemes networks: input fusion, intermediate (encompassing single-level hierarchical attention-based fusion), output fusion. By evaluating performance these techniques, we insight into suitability different network architectures scenarios application domains. Furthermore, delve challenges related architecture selection, handling incomplete data management, potential limitations Finally, spotlight promising future Transformer-based give recommendations research this rapidly evolving field.
Language: Английский
Citations
21Diagnostics, Journal Year: 2023, Volume and Issue: 13(19), P. 3147 - 3147
Published: Oct. 7, 2023
Skin lesions are essential for the early detection and management of a number dermatological disorders. Learning-based methods skin lesion analysis have drawn much attention lately because improvements in computer vision machine learning techniques. A review most-recent classification, segmentation, is presented this survey paper. The significance healthcare difficulties physical inspection discussed state-of-the-art papers targeting classification then covered depth with goal correctly identifying type from dermoscopic, macroscopic, other image formats. contribution limitations various techniques used selected study papers, including deep architectures conventional methods, examined. looks into focused on segmentation that aimed to identify precise borders classify them accordingly. These make it easier conduct subsequent analyses allow measurements quantitative evaluations. paper discusses well-known algorithms, deep-learning-based, graph-based, region-based ones. difficulties, datasets, evaluation metrics particular also discussed. Throughout survey, notable benchmark challenges, relevant highlighted, providing comprehensive overview field. concludes summary major trends, potential future directions detection, aiming inspire further advancements critical domain research.
Language: Английский
Citations
26Bioengineering, Journal Year: 2025, Volume and Issue: 12(3), P. 282 - 282
Published: March 12, 2025
The rising prevalence of skin lesions places a heavy burden on global health resources and necessitates an early precise diagnosis for successful treatment. diagnostic potential recent multi-modal lesion detection algorithms is limited because they ignore dynamic interactions information sharing across modalities at various feature scales. To address this, we propose deep learning framework, Multi-Modal Skin-Imaging-based Information-Switching Network (MDSIS-Net), end-to-end recognition. MDSIS-Net extracts intra-modality features using transfer in multi-scale fully shared convolutional neural network introduces innovative information-switching module. A cross-attention mechanism dynamically calibrates integrates to improve inter-modality associations representation this tested clinical disfiguring dermatosis data the public Derm7pt melanoma dataset. Visually Intelligent System Image Analysis (VISIA) captures five modalities: spots, red marks, ultraviolet (UV) porphyrins, brown spots dermatosis. model performs better than existing approaches with mAP 0.967, accuracy 0.960, precision 0.935, recall f1-score 0.947. Using dermoscopic pictures from dataset, outperforms current benchmarks melanoma, 0.877, 0.907, 0.911, 0.815, 0.851. model’s interpretability proven by Grad-CAM heatmaps correlating focus areas. In conclusion, our enhances identification capturing relationship fine-grained details images, improving both interpretability. This work advances decision making lays foundation future developments
Language: Английский
Citations
1Journal of Cancer Research and Clinical Oncology, Journal Year: 2023, Volume and Issue: 149(15), P. 14365 - 14408
Published: Aug. 4, 2023
Language: Английский
Citations
21Knowledge-Based Systems, Journal Year: 2024, Volume and Issue: 296, P. 111878 - 111878
Published: May 1, 2024
Language: Английский
Citations
8Engineering Applications of Artificial Intelligence, Journal Year: 2023, Volume and Issue: 127, P. 107385 - 107385
Published: Oct. 31, 2023
Language: Английский
Citations
14Computers in Biology and Medicine, Journal Year: 2024, Volume and Issue: 176, P. 108594 - 108594
Published: May 14, 2024
Language: Английский
Citations
5Archives of Computational Methods in Engineering, Journal Year: 2025, Volume and Issue: unknown
Published: Jan. 11, 2025
Language: Английский
Citations
0Journal of Computing Theories and Applications, Journal Year: 2025, Volume and Issue: 2(3)
Published: Jan. 15, 2025
Skin cancer (SC) is a highly serious kind of that, if not addressed swiftly, might result in the patient’s demise. Early detection this condition allows for more effective therapy and prevents disease development. Deep Learning (DL) approaches may be used as an efficient tool SC (SCD). Several DL-based algorithms automated SCD have been reported. However, models are needed to improve accuracy. As result, paper introduces new strategy based on Grey Wolf optimization (GWO) methodologies CNN. The proposed methodology has four stages: preprocessing, segmentation, feature extraction, classification. method utilizes Convolutional Neural Network (CNN) extract features from Regions Interest (ROIs). CNN employed categorization, whereas GWO approach enhances accuracy by refining edge segmentation. This technique probabilistic model accelerate convergence algorithm. Employing optimize structure weight vectors CNNs can enhance diagnostic minimum 5%, evaluation outcomes. application its performance comparison with other methods indicate that predicted average 95.11% without Accuracy 92.66%, respectively, enhancing 2.5% when we train our GWO.
Language: Английский
Citations
0