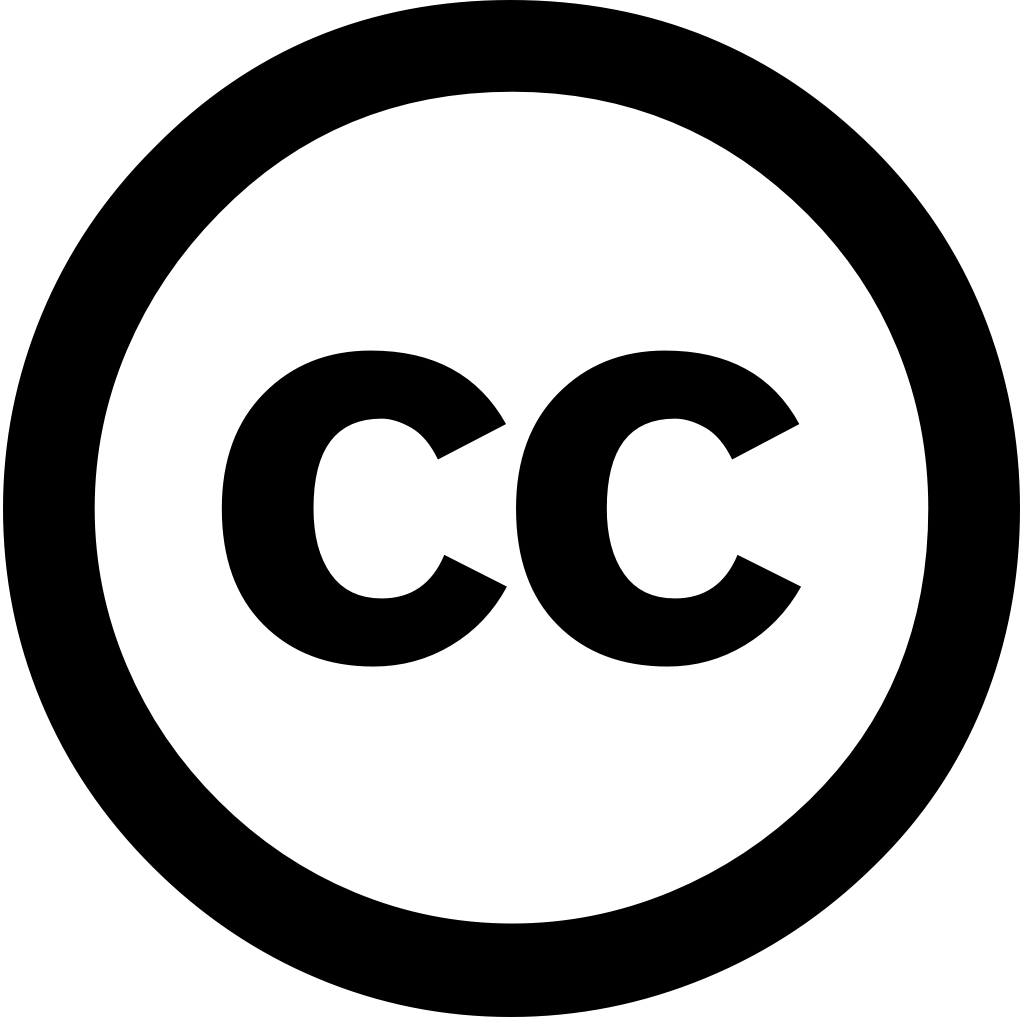
International Journal of Computational Intelligence Systems, Journal Year: 2025, Volume and Issue: 18(1)
Published: May 21, 2025
Language: Английский
International Journal of Computational Intelligence Systems, Journal Year: 2025, Volume and Issue: 18(1)
Published: May 21, 2025
Language: Английский
Scientific Reports, Journal Year: 2024, Volume and Issue: 14(1)
Published: July 19, 2024
Concrete compressive strength (CS) is a crucial performance parameter in concrete structure design. Reliable prediction reduces costs and time design prevents material waste from extensive mixture trials. Machine learning techniques solve structural engineering challenges such as CS prediction. This study used Learning (ML) models to enhance the of CS, analyzing 1030 experimental data ranging 2.33 82.60 MPa previous research databases. The ML included both non-ensemble ensemble types. were regression-based, evolutionary, neural network, fuzzy-inference-system. Meanwhile, consisted adaptive boosting, random forest, gradient boosting. There eight input parameters: cement, blast-furnace-slag, aggregates (coarse fine), fly ash, water, superplasticizer, curing days, with output. Comprehensive evaluations include visual quantitative methods k-fold cross-validation assess study's reliability accuracy. A sensitivity analysis using Shapley-Additive-exPlanations (SHAP) was conducted understand better how each variable affects CS. findings showed that Categorical-Gradient-Boosting (CatBoost) model most accurate during testing stage. It had highest determination-coefficient (R
Language: Английский
Citations
41Structures, Journal Year: 2025, Volume and Issue: 72, P. 108224 - 108224
Published: Jan. 12, 2025
Language: Английский
Citations
8Engineering Applications of Artificial Intelligence, Journal Year: 2025, Volume and Issue: 142, P. 109945 - 109945
Published: Jan. 5, 2025
Language: Английский
Citations
3Smart Construction and Sustainable Cities, Journal Year: 2025, Volume and Issue: 3(1)
Published: Jan. 26, 2025
Language: Английский
Citations
3Engineering Applications of Artificial Intelligence, Journal Year: 2024, Volume and Issue: 137, P. 109121 - 109121
Published: Aug. 27, 2024
Language: Английский
Citations
11Archives of Civil and Mechanical Engineering, Journal Year: 2024, Volume and Issue: 24(3)
Published: July 4, 2024
Language: Английский
Citations
9Engineering Structures, Journal Year: 2025, Volume and Issue: 329, P. 119752 - 119752
Published: Feb. 5, 2025
Language: Английский
Citations
1Archives of Computational Methods in Engineering, Journal Year: 2025, Volume and Issue: unknown
Published: April 10, 2025
Language: Английский
Citations
1Canadian Geotechnical Journal, Journal Year: 2025, Volume and Issue: 62, P. 1 - 17
Published: Jan. 1, 2025
Artificial ground freezing (AGF) is a widely used technique for soil stabilization and waterproofing. Numerous studies have been devoted to solving the heat transfer problems in AGF while encountering limitations handling complex geometries boundary conditions being computationally intensive. Recently, using machine learning methods predict temperature fields has gained attention, demonstrating potential achieve higher accuracy than conventional models. However, these are typically limited by need large, labeled datasets, which time-consuming difficult obtain. In this study, we address challenges applying physics-informed neural networks (PINNs) solve steady-state problem AGF, focusing on distribution around single pipe. By embedding conduction equation into loss function, PINNs reduce extensive data. To enhance efficiency, employed, results compared against finite element method. Results show that high accuracy, particularly larger domains with moderate gradients, providing competitive performance more configurations involving steeper gradients. This approach offers promising alternative modeling geotechnical applications, implications reducing computational costs design.
Language: Английский
Citations
1Smart Construction and Sustainable Cities, Journal Year: 2023, Volume and Issue: 1(1)
Published: Nov. 10, 2023
Abstract Efforts to reduce the weight of buildings and structures, counteract seismic threat human life, cut down on construction expenses are widespread. A strategy employed address these challenges involves adoption foam concrete. Unlike traditional concrete, concrete maintains standard composition but excludes coarse aggregates, substituting them with a agent. This alteration serves dual purpose: diminishing concrete’s overall weight, thereby achieving lower density than regular creating voids within material due agent, resulting in excellent thermal conductivity. article delves into presentation statistical models utilizing three different methods—linear (LR), non-linear (NLR), artificial neural network (ANN)—to predict compressive strength These formulated based dataset 97 sets experimental data sourced from prior research endeavors. comparative evaluation outcomes is subsequently conducted, leveraging benchmarks like coefficient determination ( R 2 ), root mean square error (RMSE), absolute (MAE), aim identifying most proficient model. The results underscore remarkable effectiveness ANN evident model’s value, which surpasses that LR model by 36% 22%. Furthermore, demonstrates significantly MAE RMSE values compared both NLR models.
Language: Английский
Citations
23