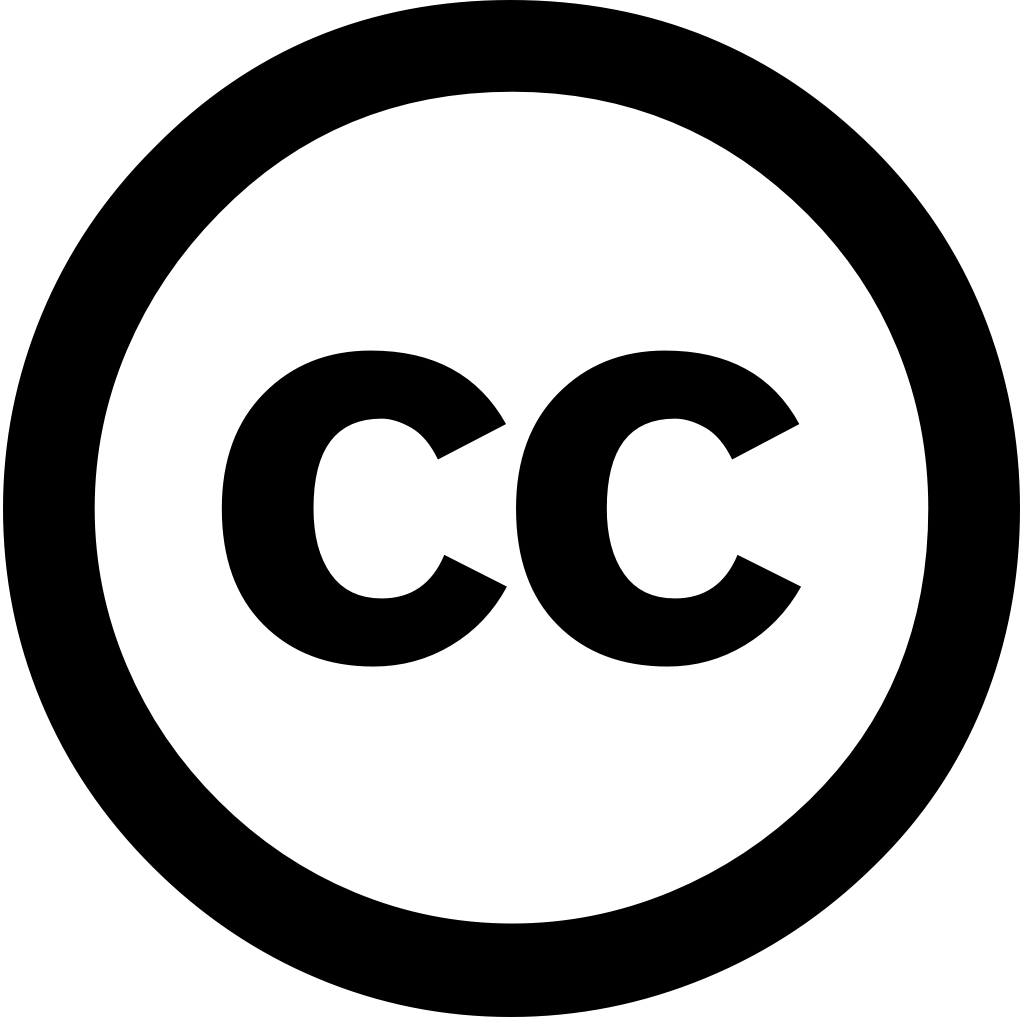
SN Computer Science, Journal Year: 2024, Volume and Issue: 5(8)
Published: Dec. 14, 2024
Language: Английский
SN Computer Science, Journal Year: 2024, Volume and Issue: 5(8)
Published: Dec. 14, 2024
Language: Английский
Engineering Applications of Artificial Intelligence, Journal Year: 2023, Volume and Issue: 126, P. 106951 - 106951
Published: Aug. 12, 2023
Language: Английский
Citations
31Cluster Computing, Journal Year: 2024, Volume and Issue: unknown
Published: June 17, 2024
Abstract
Skin
cancer
is
one
of
the
most
dangerous
types
due
to
its
immediate
appearance
and
possibility
rapid
spread.
It
arises
from
uncontrollably
growing
cells,
rapidly
dividing
cells
in
area
body,
invading
other
bodily
tissues,
spreading
throughout
body.
Early
detection
helps
prevent
progress
reaching
critical
levels,
reducing
risk
complications
need
for
more
aggressive
treatment
options.
Convolutional
neural
networks
(CNNs)
revolutionize
skin
diagnosis
by
extracting
intricate
features
images,
enabling
an
accurate
classification
lesions.
Their
role
extends
early
detection,
providing
a
powerful
tool
dermatologists
identify
abnormalities
their
nascent
stages,
ultimately
improving
patient
outcomes.
This
study
proposes
novel
deep
convolutional
network
(DCNN)
approach
classifying
The
proposed
DCNN
model
evaluated
using
two
unbalanced
datasets,
namely
HAM10000
ISIC-2019.
compared
with
transfer
learning
models,
including
VGG16,
VGG19,
DenseNet121,
DenseNet201,
MobileNetV2.
Its
performance
assessed
four
widely
used
evaluation
metrics:
accuracy,
recall,
precision,
F1-score,
specificity,
AUC.
experimental
results
demonstrate
that
outperforms
(DL)
models
utilized
these
datasets.
achieved
highest
accuracy
ISIC-2019
$$98.5\%$$
Language: Английский
Citations
12Engineering Applications of Artificial Intelligence, Journal Year: 2023, Volume and Issue: 127, P. 107385 - 107385
Published: Oct. 31, 2023
Language: Английский
Citations
14Expert Systems with Applications, Journal Year: 2023, Volume and Issue: 238, P. 122076 - 122076
Published: Oct. 16, 2023
Language: Английский
Citations
10Canadian Metallurgical Quarterly, Journal Year: 2025, Volume and Issue: unknown, P. 1 - 20
Published: May 21, 2025
Language: Английский
Citations
0Expert Systems with Applications, Journal Year: 2025, Volume and Issue: unknown, P. 128231 - 128231
Published: May 1, 2025
Language: Английский
Citations
0ACM Transactions on Asian and Low-Resource Language Information Processing, Journal Year: 2024, Volume and Issue: unknown
Published: Feb. 16, 2024
In the field of disease diagnosis, medical image classification faces an inherent challenge due to various factors involving data imbalance, quality variability, annotation and limited availability representativeness. Such challenges affect algorithm's ability on images in adverse way, which leads biased model outcomes inaccurate interpretations. this paper, a novel Discrete Levy Flight Grey Wolf Optimizer (DLFGWO) is combined with Random Forest (RF) classifier address above limitations biomedical datasets achieve better rate. The DLFGWO-RF resolves variability ultrasound limits inaccuracies using RF by handling incomplete noisy data. sheer focus majority class may lead unequal distribution classes thus imbalance. DLFGWO balances such leveraging grey wolves its exploration exploitation capabilities are improved (DLF). It further optimizes classifier's performance balanced designed perform even datasets, thereby requirement numerous expert annotations can be reduced. diabetic retinopathy grading, reduces disagreements subjective However, representativeness dataset fails capture entire population diversity, generalization proposed DLFGWO-RF. Thus, fine-tuning robustly adapt subgroups dataset, enhancing overall performance. experiments conducted two widely used test efficacy model. experimental results show that achieves accuracy between 90-95%, outperforms existing techniques for imbalanced datasets.
Language: Английский
Citations
1International Journal of Web Information Systems, Journal Year: 2024, Volume and Issue: unknown
Published: Nov. 20, 2024
Purpose This study aims to introduce a novel rank aggregation algorithm that leverages graph theory and deep-learning improve the accuracy relevance of aggregated rankings in metasearch scenarios, particularly when faced with inconsistent low-quality lists. By strategically selecting subset base rankers, enhances quality ranking while using only rankers. Design/methodology/approach The proposed graph-based model represent interrelationships between applying Spectral clustering, identifies top-performing rankers based on their retrieval effectiveness. These selected are then integrated into sequential estimate labels for query-document pairs. Findings Empirical evaluation MQ2007-agg MQ2008-agg data sets demonstrates substantial performance gains achieved by compared baseline methods, an average improvement 8.7% MAP 11.9% NDCG@1. algorithm’s effectiveness can be attributed its ability effectively integrate diverse perspectives from capture complex relationships within data. Originality/value research presents approach integrates deep-learning. author proposes select most effective applications constructing similarity innovative method addresses challenges posed lists, offering unique solution problem.
Language: Английский
Citations
0The North American Journal of Economics and Finance, Journal Year: 2024, Volume and Issue: unknown, P. 102318 - 102318
Published: Nov. 1, 2024
Language: Английский
Citations
0SN Computer Science, Journal Year: 2024, Volume and Issue: 5(8)
Published: Dec. 14, 2024
Language: Английский
Citations
0