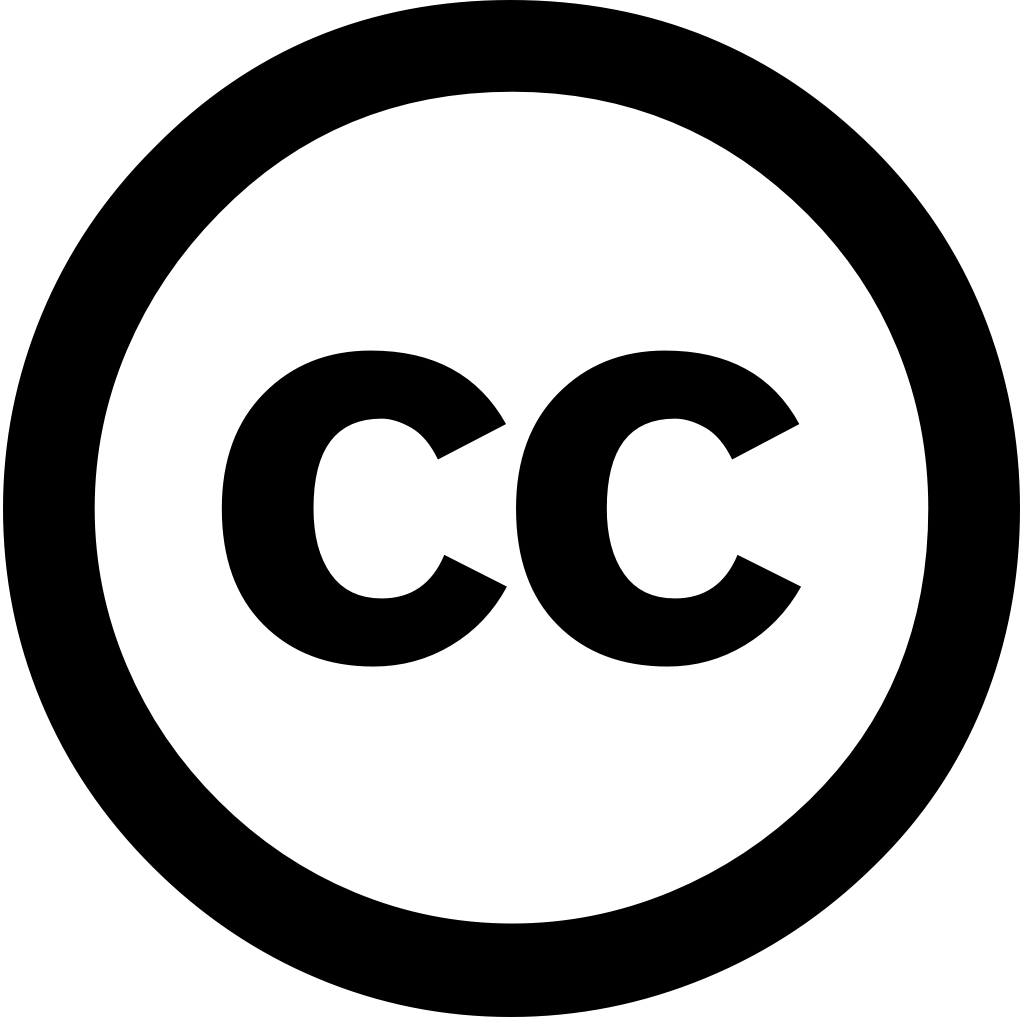
Automation in Construction, Journal Year: 2025, Volume and Issue: 175, P. 106201 - 106201
Published: April 24, 2025
Language: Английский
Automation in Construction, Journal Year: 2025, Volume and Issue: 175, P. 106201 - 106201
Published: April 24, 2025
Language: Английский
Engineering Applications of Artificial Intelligence, Journal Year: 2025, Volume and Issue: 143, P. 110068 - 110068
Published: Jan. 15, 2025
Language: Английский
Citations
2Engineering Applications of Artificial Intelligence, Journal Year: 2025, Volume and Issue: 143, P. 110045 - 110045
Published: Jan. 18, 2025
Language: Английский
Citations
0Scientific Reports, Journal Year: 2025, Volume and Issue: 15(1)
Published: March 13, 2025
Consistent detection of cracks in engineering structures is essential for maintaining structural integrity. Deep neural networks perform well this discipline, although their pixel-level labeling reliance increases costs. Thus, weakly supervised learning methods have emerged. However, labels are substantially worse quality than those manual labeling. Current deep network visual interpretation approaches issues including erroneous target localization. This study proposes an Affine Transformation and Pseudo Label Refinement (AT-CAM) method. The methodology comprises three phases: the initial phase employs a geometric enhancement strategy to produce sequence enhanced images from input images, utilizing Axiom-based Grad-CAM (XGradCAM) algorithm generate class activation maps each image, which subsequently amalgamated into unified saliency map; subsequent phase, information flow pathways subsampling convolutional layer modified by designated Hook. samples utilized invert eliminate checkerboard noise produced during integration spatially; third stage, dynamic range compression mechanism employed augment prominence cracked areas compressing highlighted regions map diminishing influence background noise. experimental results indicate that method proposed segmentation accuracy 7.2% relative original baseline, markedly improves interpretability networks, offers novel, efficient, cost-effective, interpretable approach detecting engineering.
Language: Английский
Citations
0Automation in Construction, Journal Year: 2025, Volume and Issue: 174, P. 106120 - 106120
Published: March 21, 2025
Language: Английский
Citations
0Automation in Construction, Journal Year: 2025, Volume and Issue: 175, P. 106201 - 106201
Published: April 24, 2025
Language: Английский
Citations
0