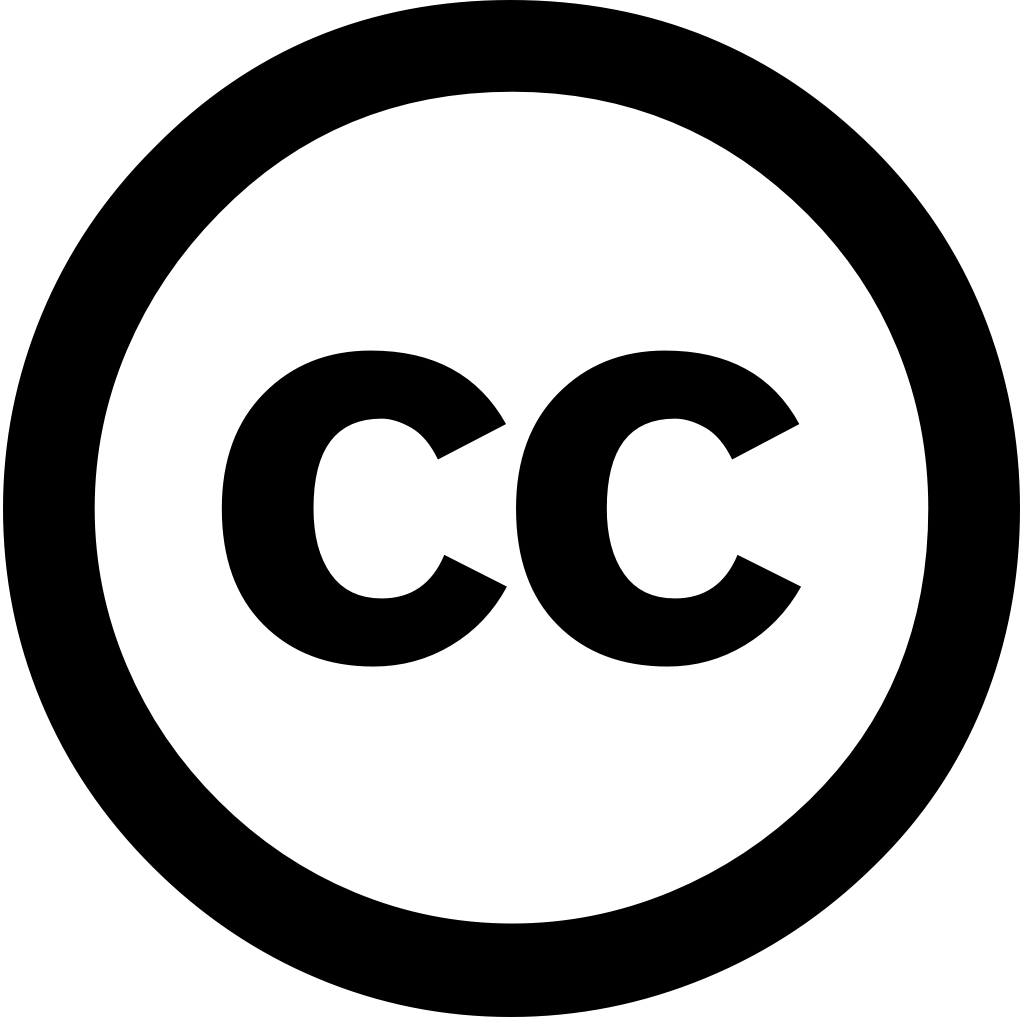
Sensors, Journal Year: 2025, Volume and Issue: 25(6), P. 1692 - 1692
Published: March 8, 2025
Maintaining effluent quality in wastewater treatment plants (WWTPs) comes with significant challenges under variable weather conditions, where sudden changes flow rate and increased pollutant loads can affect performance. Traditional physical sensors became both expensive susceptible to failure extreme conditions. In this study, we evaluate the performance of soft based on artificial intelligence (AI) predict components underlying calculation index (EQI). We thus focus our study three ML models: Long Short-Term Memory (LSTM), Gated Recurrent Unit (GRU) Transformer. Using Benchmark Simulation Model no. 2 (BSM2) as WWTP, were able obtain datasets for training models their dry scenarios, rainy episodes, storm events. To improve classification networks according type weather, developed a Random Forest (RF)-based meta-classifier. The results indicate that conditions Transformer network achieved best performance, while rain episodes scenarios GRU was capture variations highest accuracy. LSTM performed normally stable but struggled rapid fluctuations. These support decision integrate AI-based predictive WWTPs, highlighting top performances recurrent feed-forward (Transformer) obtaining predictions different
Language: Английский