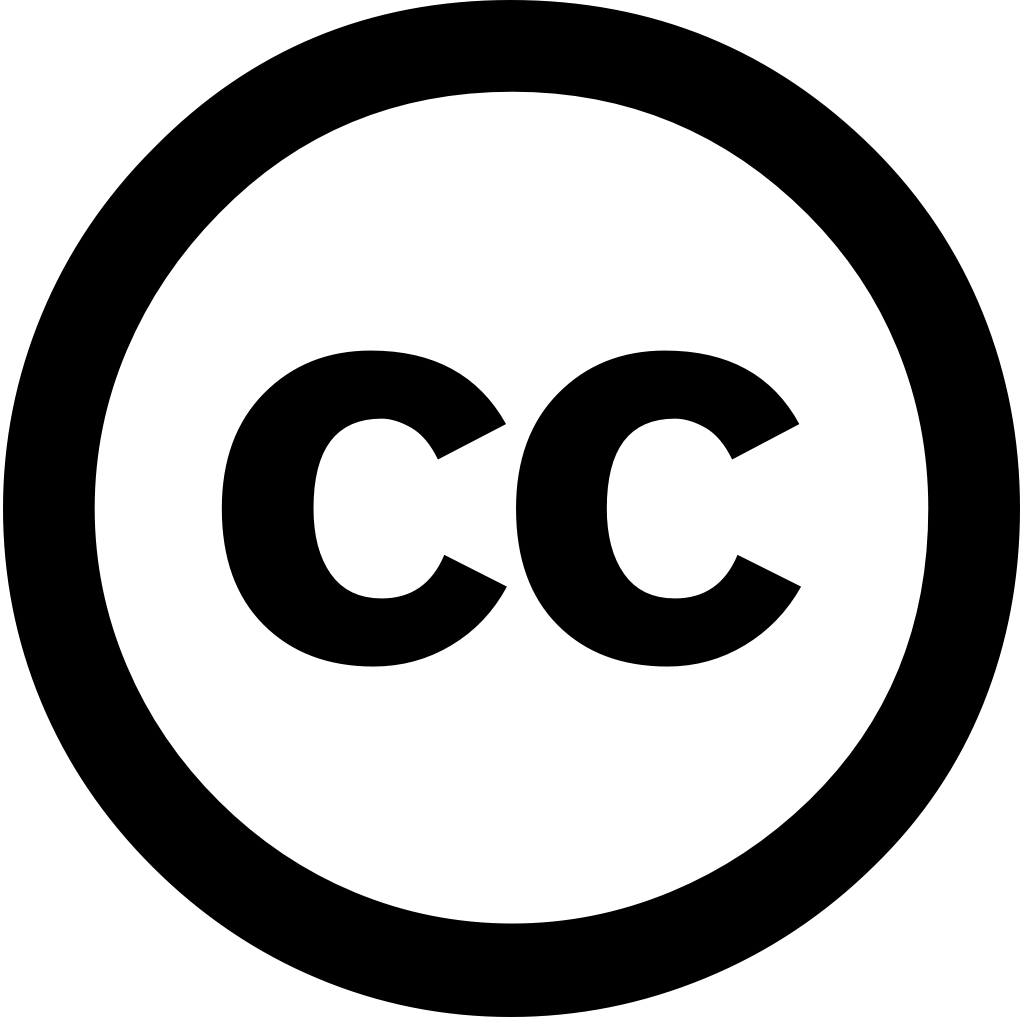
Journal of Marine Science and Engineering, Journal Year: 2025, Volume and Issue: 13(3), P. 598 - 598
Published: March 18, 2025
The suction-lifting system of cutter suction dredgers consumes a large amount energy. Optimizing their performance is great significance for enhancing the overall efficiency dredgers. This study proposes effective specific cutting energy, new indicator suitable evaluating energy consumption It reflects cooperation state between and pump-pipe has important reference value improving construction efficiency. calculation method given, which calculated by motor power, slurry concentration, flow rate. Based on machine learning framework, model framework predicting according to relevant parameters constructed. Real ship data from dredger “Changshi 12” are used experiments. First, eigenvalue screening carried out based dredging knowledge mechanism, then outliers removed, finally processing performed using Spearman correlation coefficient PCA dimensionality reduction techniques. Subsequently, five algorithms, such as RF XGBoost, in combination with grid search find optimal hyperparameters, Lasso meta-learner integrate prediction results. experimental results show that Random Forest Stacking models have high accuracy rate, verifying feasibility this method.
Language: Английский