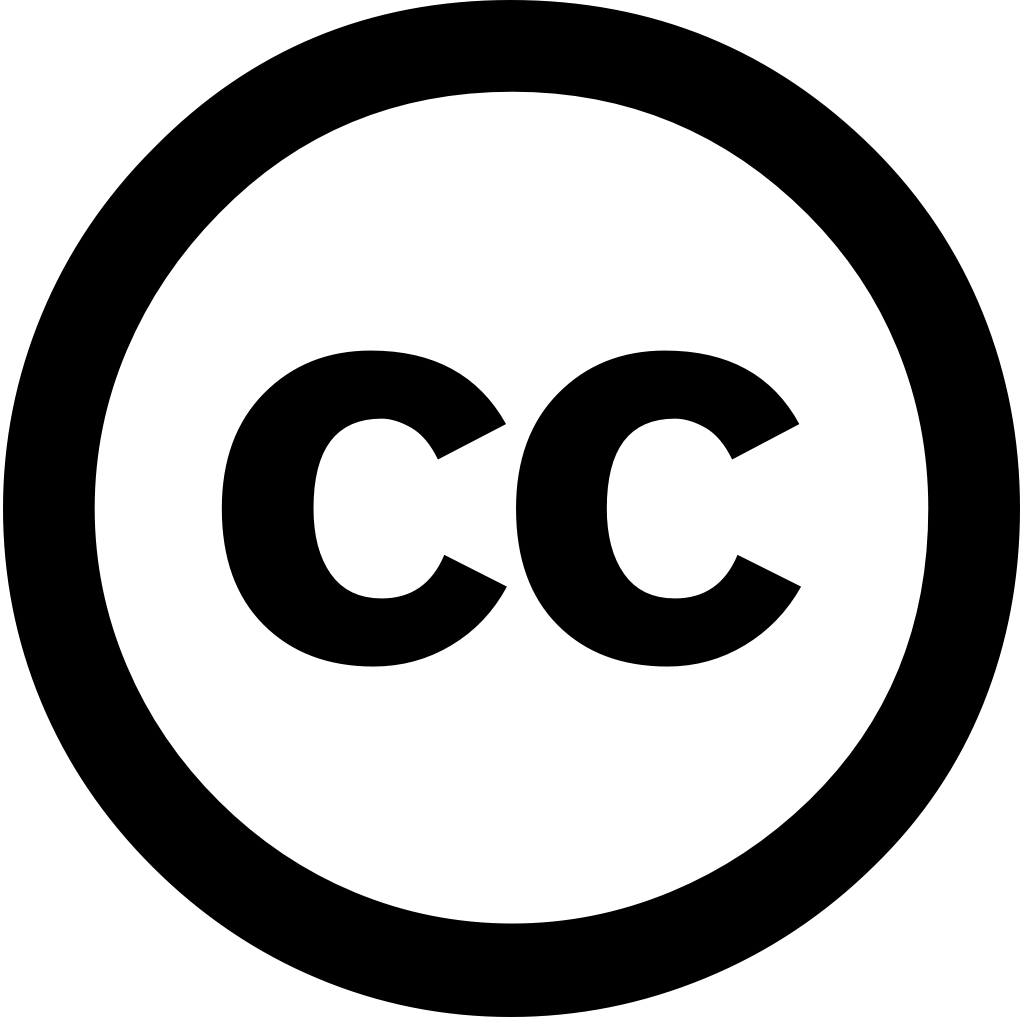
Construction and Building Materials, Journal Year: 2024, Volume and Issue: 437, P. 137079 - 137079
Published: June 14, 2024
Language: Английский
Construction and Building Materials, Journal Year: 2024, Volume and Issue: 437, P. 137079 - 137079
Published: June 14, 2024
Language: Английский
Case Studies in Construction Materials, Journal Year: 2024, Volume and Issue: 20, P. e02842 - e02842
Published: Jan. 4, 2024
This study employs soft computing techniques, including artificial neural network (ANN) models and gene expression programming (GEP), to enhance the prediction of ultimate load in timber pull-out tests under varying environmental conditions. A comprehensive dataset 202 samples normal conditions 324 harsh was gathered. Distinct were developed for each scenario, achieving commendable accuracies. The ANN performed at 0.91 0.99 conditions, while GEP 0.94, respectively. also predicted free end slip using an model with accuracy 0.97. SHapley values technique employed assess impact features on models, revealing specific influential features. In rod type most influential, bonded length demonstrated highest impact. Additionally, duration immersion feature had substantial effect predicting slip. final section compared data experimental values, showing a noteworthy correlation over 60% between outputs established models.
Language: Английский
Citations
20Engineering Applications of Artificial Intelligence, Journal Year: 2024, Volume and Issue: 136, P. 108854 - 108854
Published: July 4, 2024
Despite the widespread application of data-centric techniques in Geotechnical Engineering, there is a rising need for building trust artificial intelligence (AI)-driven safety assessment road embankments due to its so-called "black-box" nature. In addition, from lens limit equilibrium approaches, e.g., Bishop, Fellenius, Janbu and Morgenstern–Price, finite element method, it essential carefully examine interplay both topological physical/mechanical properties during factor (FoS) predictions. First, aside having conventional geotechnical inputs soil core foundation height embankments, this paper codifies geometric features innovatively. The number slope types with different ratios including 1:1, 1.5:1 2:1 as well berms introduced. Second, pool 19 machine learning (ML) effortlessly trained on dataset using an automated ML (AutoML) pipeline identify most optimized algorithm. Finally, achieve post-hoc interpretability internal mechanism input–output relationship unbiasedly, game-theory-based explainable AI (XAI) method called Shapley additive explanations (SHAP) values applied. SHAP-aided importance analysis provides human-interpretable insights indicates height, California bearing ratio, type cohesion influential parameters. Exclusively, analyzing hazardous by classifying main joint contributors exhibits complex highly variable influence FoS. This harnesses power XAI tools enhance reliability transparency rapid FoS prediction slopes. It targets researchers, practitioners, decision-makers, general public first time.
Language: Английский
Citations
18Cleaner Materials, Journal Year: 2025, Volume and Issue: unknown, P. 100300 - 100300
Published: Feb. 1, 2025
Language: Английский
Citations
2Earth Science Informatics, Journal Year: 2025, Volume and Issue: 18(3)
Published: Feb. 19, 2025
Language: Английский
Citations
2Journal of Forestry Research, Journal Year: 2024, Volume and Issue: 35(1)
Published: Sept. 27, 2024
Language: Английский
Citations
13Archives of Computational Methods in Engineering, Journal Year: 2024, Volume and Issue: unknown
Published: July 13, 2024
Language: Английский
Citations
12Deleted Journal, Journal Year: 2025, Volume and Issue: 2(1)
Published: Feb. 27, 2025
Language: Английский
Citations
1Journal of Constructional Steel Research, Journal Year: 2025, Volume and Issue: 229, P. 109509 - 109509
Published: March 11, 2025
Language: Английский
Citations
1Journal of Structural Engineering, Journal Year: 2025, Volume and Issue: 151(6)
Published: March 30, 2025
Language: Английский
Citations
1Structures, Journal Year: 2024, Volume and Issue: 65, P. 106653 - 106653
Published: June 14, 2024
Language: Английский
Citations
6