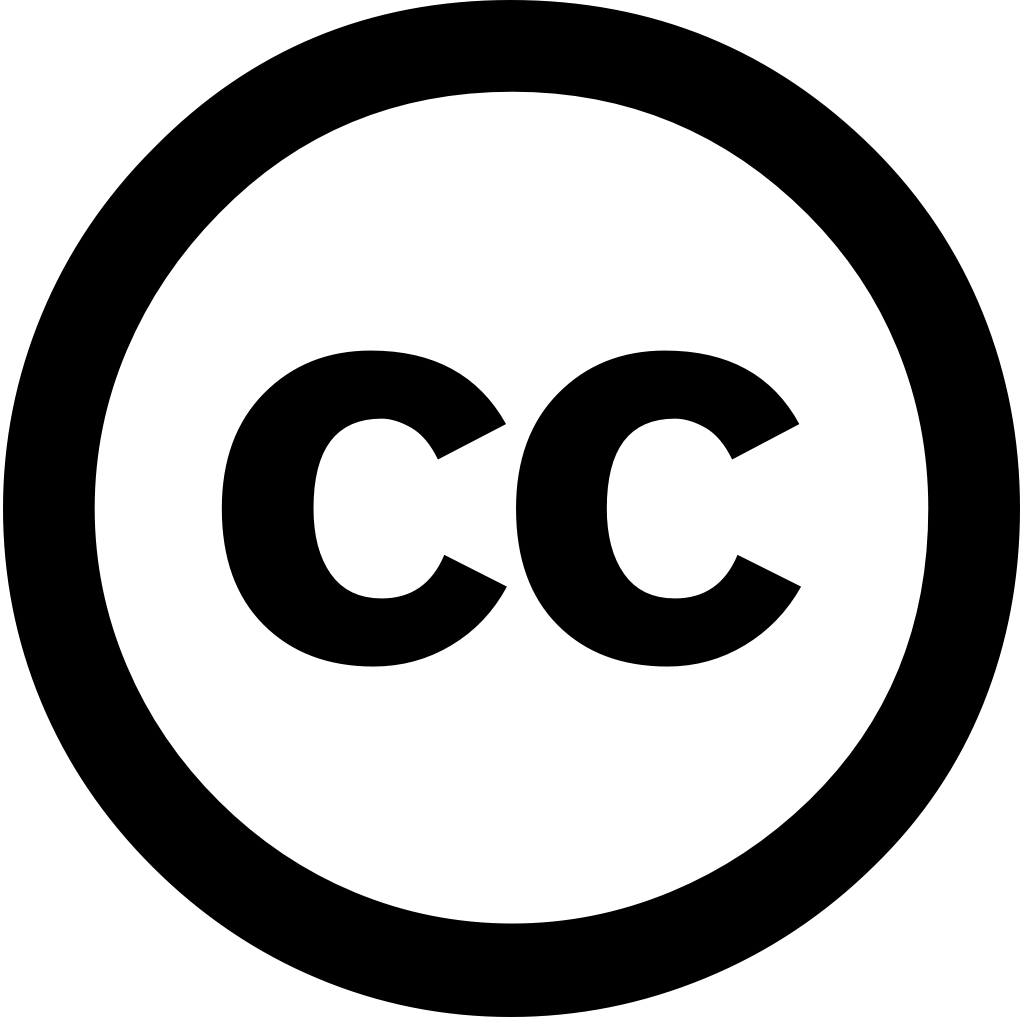
Environmental Research, Journal Year: 2024, Volume and Issue: 264, P. 120292 - 120292
Published: Nov. 7, 2024
Language: Английский
Environmental Research, Journal Year: 2024, Volume and Issue: 264, P. 120292 - 120292
Published: Nov. 7, 2024
Language: Английский
Journal of Environmental Management, Journal Year: 2025, Volume and Issue: 377, P. 124691 - 124691
Published: Feb. 27, 2025
Language: Английский
Citations
1Environmental Monitoring and Assessment, Journal Year: 2024, Volume and Issue: 196(10)
Published: Sept. 20, 2024
Language: Английский
Citations
5Journal of Environmental Management, Journal Year: 2024, Volume and Issue: 371, P. 122902 - 122902
Published: Nov. 11, 2024
Language: Английский
Citations
4Journal of Contaminant Hydrology, Journal Year: 2024, Volume and Issue: 269, P. 104480 - 104480
Published: Dec. 10, 2024
Language: Английский
Citations
4Land, Journal Year: 2025, Volume and Issue: 14(1), P. 69 - 69
Published: Jan. 2, 2025
Nitrate leaching from soil presents a significant threat to health, as it can result in nutrient loss, acidification, and structural damage. It is crucial quantify the spatial heterogeneity of nitrate its drivers. A total 509 observational data points regarding northern China were collected, capturing temporal variations across crops such winter wheat, maize, greenhouse vegetables. machine learning (ML) model for predicting was then developed, with random forest (RF) outperforming support vector (SVM), extreme gradient boosting (XGBoost), convolutional neural network (CNN) models, achieving an R2 0.75. However, performance improved significantly after integrating four models Bayesian optimization (all had > 0.56), which realized quantitative prediction capabilities loss concentrations. Moreover, XGBoost exhibited highest fitting accuracy smallest error estimating losses, value 0.79 average absolute (MAE) 3.87 kg/ha. Analyses feature importance SHAP values optimal identified organic matter, chemical nitrogen fertilizer input, water input (including rainfall irrigation) main indicators loss. The ML-based modeling method developed overcomes difficulty determination functional relationship between intensity influencing factors, providing data-driven solution nitrate–nitrogen farmlands North strengthening sustainable agricultural practices.
Language: Английский
Citations
0Environmental and Sustainability Indicators, Journal Year: 2025, Volume and Issue: unknown, P. 100582 - 100582
Published: Jan. 1, 2025
Language: Английский
Citations
0Environmental Monitoring and Assessment, Journal Year: 2025, Volume and Issue: 197(3)
Published: Feb. 19, 2025
Language: Английский
Citations
0Water, Journal Year: 2025, Volume and Issue: 17(7), P. 936 - 936
Published: March 23, 2025
Groundwater, which constitutes 95% of the world’s freshwater resources, is widely used for drinking and domestic water supply, agricultural irrigation, energy production, bottled commercial use. In recent years, due to pressures from climate change excessive urbanization, a noticeable decline in groundwater levels has been observed, particularly arid semi-arid regions. The corresponding changes have analyzed using diverse range methodologies, including data-driven modeling techniques. Recent evidence shown notable acceleration utilization such advanced techniques, demonstrating significant attention by research community. Therefore, major aim present study conduct bibliometric analysis investigate application evolution machine learning (ML) techniques research. this sense, studies published between 2000 2023 were examined terms scientific productivity, collaboration networks, themes, methods. findings revealed that ML offer high accuracy predictive capacity, especially quality, level estimation, pollution modeling. United States, China, Iran stand out as leading countries emphasizing strategic importance management. However, outcomes demonstrated low international cooperation led deficiencies solving transboundary problems. aimed encourage more widespread effective use management environmental planning processes drew transparent interpretable algorithms, with potential yield rewarding opportunities increasing adoption technologies decision-makers.
Language: Английский
Citations
0Computers and Electronics in Agriculture, Journal Year: 2025, Volume and Issue: 236, P. 110430 - 110430
Published: April 26, 2025
Language: Английский
Citations
0Water, Journal Year: 2024, Volume and Issue: 16(15), P. 2179 - 2179
Published: Aug. 1, 2024
Heavy metal pollution seriously threatens the drinking water safety and ecological environment in karst lead–zinc mines. Fifteen groundwater surface samples were collected a mine Daxin, Chongzuo. Ten heavy (Mn, Zn, As, Pb, Cr, Cd, Ni, Co, Cu, Fe) concentrations detected. Correlation cluster analysis utilized to explore distribution characteristics sources. The health risks appraised using risk assessment model. had more types than water, of which average exceeded class III quality standard. drainage contributed most (65.10%) concentrations. Mn, Fe primarily originated from mining mine, Cr came fuel combustion wear metals, As was connected with regional geological background. higher total (5.12 × 10−4 a−1) (2.17 a−1). In comparison non-carcinogenic risk, carcinogenic increased by three five orders magnitude. Cd represented pattern. pathway posed two magnitude amount that dermal contact posed. Children suffered greater risks. Water security for children should be strictly controlled. must paid attention terms protection management.
Language: Английский
Citations
2