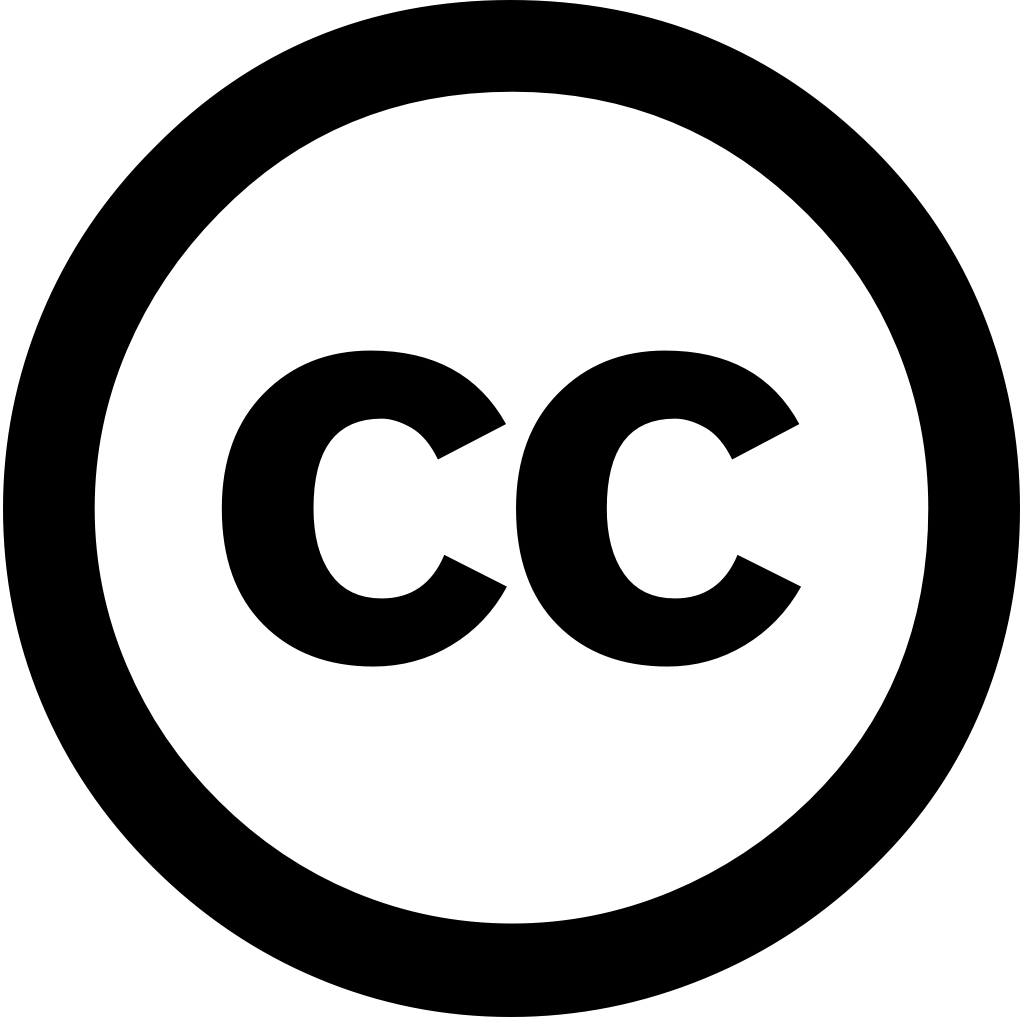
Results in Engineering, Journal Year: 2024, Volume and Issue: unknown, P. 103033 - 103033
Published: Oct. 1, 2024
Language: Английский
Results in Engineering, Journal Year: 2024, Volume and Issue: unknown, P. 103033 - 103033
Published: Oct. 1, 2024
Language: Английский
Environmental Technology & Innovation, Journal Year: 2023, Volume and Issue: 33, P. 103509 - 103509
Published: Dec. 29, 2023
Nowadays the pace of production and consumption is reaching environmentally unsustainable levels. In this regard, great technological advances developed in recent years are postulated as a source opportunities to boost circular economy sustainable development. This wide range possibilities offered by new technologies create more reality has aroused curiosity interest academic world, especially years. The main objective research reveal challenges that arise when incorporating objectives economy. Regarding methodology, study been partially supported using bibliometric techniques. results highlight transformative role technologies, blockchain artificial intelligence, advancing economy, with particular emphasis on community technology integration, ethical considerations, synergies, business models, burgeoning bioeconomy. We conclude promise enhanced resource efficiency, optimized supply chains, innovative improved product lifecycle management, offering profound economic environmental benefits while fostering collaborative innovation. However, these also represent address, such integrating advanced methods, ensuring chain transparency, overcoming skill gap, avoiding data centralization, adapting regulatory frameworks foster equitable growth. These some most important areas for further research, those related development employees' capabilities adaptation frameworks, they understudied gaps.
Language: Английский
Citations
87International Journal of Hydrogen Energy, Journal Year: 2024, Volume and Issue: 67, P. 1009 - 1025
Published: Jan. 19, 2024
Language: Английский
Citations
52Sustainable Cities and Society, Journal Year: 2024, Volume and Issue: unknown, P. 105570 - 105570
Published: June 1, 2024
Language: Английский
Citations
25Sustainable Energy Research, Journal Year: 2024, Volume and Issue: 11(1)
Published: July 20, 2024
Abstract The global energy sector is currently undergoing a transformative shift mainly driven by the ongoing and increasing demand for clean, sustainable, reliable solutions. However, integrating renewable sources (RES), such as wind, solar, hydropower, introduces major challenges due to intermittent variable nature of RES, affecting grid stability reliability. Hybrid storage systems (HESS), which combine multiple devices (ESDs), present promising solution leveraging complementary strengths each technology involved. This comprehensive review examines recent advancements in grid-connected HESS, focusing on their components, design considerations, control strategies, applications. It provides detailed analysis technological progress various ESDs critical role power conversion, control, management, cooling optimizing HESS performance. Highlighting case studies some notable successful implementations across globe, we illustrate practical applications identify benefits encountered. By addressing these challenges, can significantly enhance efficiency reliability supporting towards sustainable resilient infrastructure. paper concludes identifying future research directions, highlighting development intelligent systems, materials, efficient recycling processes ensure widespread adoption long-term viability HESS.
Language: Английский
Citations
23Neural Computing and Applications, Journal Year: 2024, Volume and Issue: 36(21), P. 12655 - 12699
Published: May 13, 2024
Abstract Artificial neural networks (ANN), machine learning (ML), deep (DL), and ensemble (EL) are four outstanding approaches that enable algorithms to extract information from data make predictions or decisions autonomously without the need for direct instructions. ANN, ML, DL, EL models have found extensive application in predicting geotechnical geoenvironmental parameters. This research aims provide a comprehensive assessment of applications addressing forecasting within field related engineering, including soil mechanics, foundation rock environmental geotechnics, transportation geotechnics. Previous studies not collectively examined all algorithms—ANN, EL—and explored their advantages disadvantages engineering. categorize address this gap existing literature systematically. An dataset relevant was gathered Web Science subjected an analysis based on approach, primary focus objectives, year publication, geographical distribution, results. Additionally, study included co-occurrence keyword covered techniques, systematic reviews, review articles data, sourced Scopus database through Elsevier Journal, were then visualized using VOS Viewer further examination. The results demonstrated ANN is widely utilized despite proven potential methods engineering due real-world laboratory civil engineers often encounter. However, when it comes behavior scenarios, techniques outperform three other methods. discussed here assist understanding benefits geo area. enables practitioners select most suitable creating certainty resilient ecosystem.
Language: Английский
Citations
20IEEE Access, Journal Year: 2024, Volume and Issue: 12, P. 50459 - 50488
Published: Jan. 1, 2024
Wireless Sensor Networks (WSNs) play a critical role in numerous applications, and the accurate localization of sensor nodes is vital for their effective operation. In recent years, optimization algorithms have garnered significant attention as means to enhance WSN node localization. This paper presents an in-depth exploration necessity offers comprehensive review used this purpose. The encompasses diverse range techniques, including evolutionary algorithms, swarm intelligence, metaheuristic approaches. Key factors, such accuracy, scalability, computational complexity, robustness, are systematically evaluated compared across various algorithms. Additionally, sheds light on strengths limitations each approach discusses applicability different deployment scenarios. insights provided serve valuable resource researchers practitioners seeking optimize localization, thus promoting efficient reliable operation WSNs real-world applications.
Language: Английский
Citations
16IEEE Access, Journal Year: 2024, Volume and Issue: 12, P. 111858 - 111881
Published: Jan. 1, 2024
Short-term load forecasting (STLF) is vital in effectively managing the reserve requirement modern power grids. Subsequently, it supports grid operator making effective and economical decisions during balancing operation. Therefore, this study comprehensively reviews STLF methods, including time series analysis, regression-based frameworks, artificial neural networks (ANNs), hybrid models that employ different approaches. Detailed mathematical graphical analyses a comparative evaluation of these methods are provided, highlighting their advantages disadvantages. Further, proposes CNN-LSTM model comprised Convolutional (CNN) for feature extraction high dimensional data Long short-term memory (LSTM) to boost model's efficiency temporal sequence prediction. This assessed using comprehensive dataset from Pakistan's NTDC national grid. The analysis revealed superior performance prediction, achieving enhanced accuracy. For single-step forecasting, yielded an RMSE 538.71, MAE 371.97, MAPE 2.72. In 24-hour achieved 951.94, 656.35, 4.72 on dataset. Moreover, has outperformed previous comparison AEP dataset, demonstrating its superiority enhancing management supply demand electricity networks.
Language: Английский
Citations
15Energy, Journal Year: 2024, Volume and Issue: 300, P. 131571 - 131571
Published: May 7, 2024
Language: Английский
Citations
14Electric Power Systems Research, Journal Year: 2024, Volume and Issue: 229, P. 110138 - 110138
Published: Jan. 16, 2024
Language: Английский
Citations
11Remote Sensing Applications Society and Environment, Journal Year: 2024, Volume and Issue: 35, P. 101265 - 101265
Published: June 2, 2024
Language: Английский
Citations
10