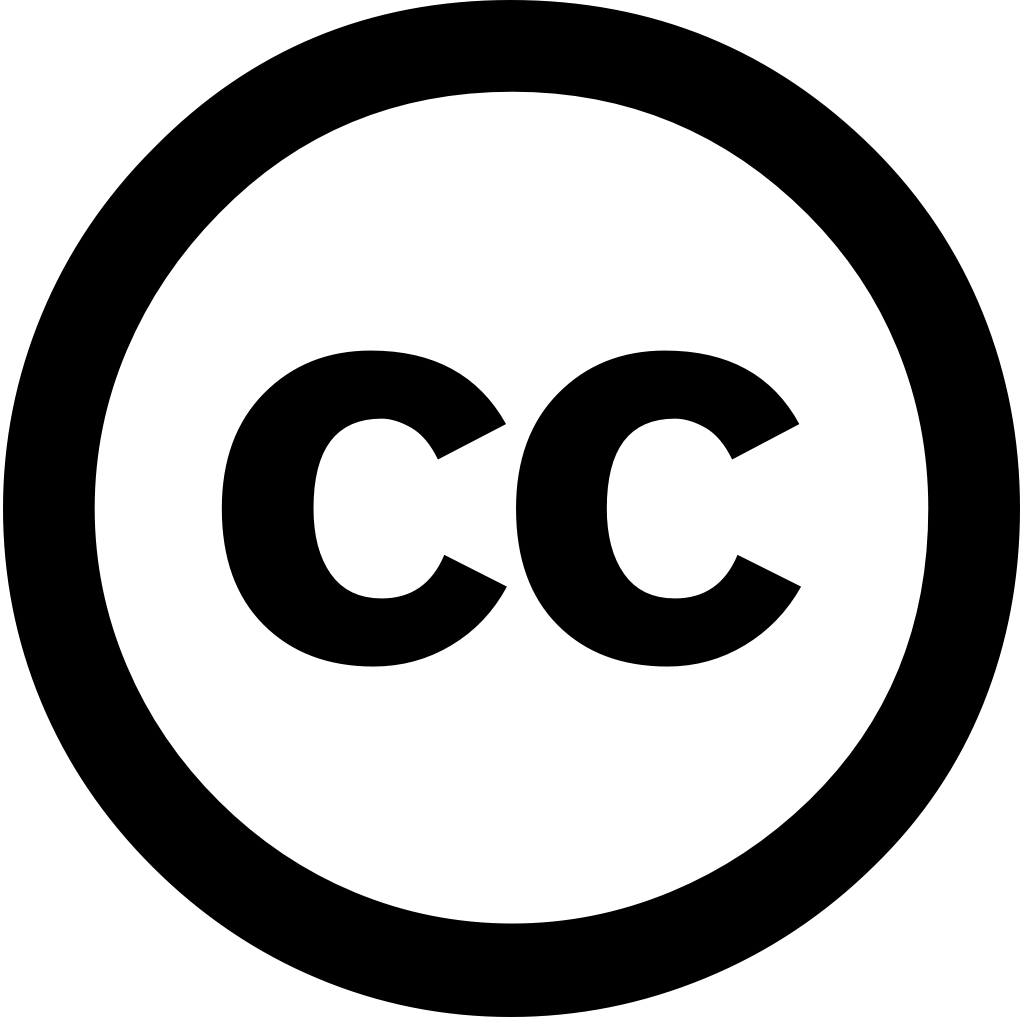
Engineering Optimization, Journal Year: 2025, Volume and Issue: unknown, P. 1 - 36
Published: April 4, 2025
Language: Английский
Engineering Optimization, Journal Year: 2025, Volume and Issue: unknown, P. 1 - 36
Published: April 4, 2025
Language: Английский
IEEE Access, Journal Year: 2024, Volume and Issue: 12, P. 39887 - 39901
Published: Jan. 1, 2024
The
rapid
growth
of
data
quantity
directly
leads
to
the
increasing
feature
dimension,
which
challenges
machine
learning
and
mining.
Wrapper-based
intelligent
swarm
algorithms
are
effective
solution
techniques.
Grey
Wolf
Optimization
(GWO)
algorithm
is
a
novel
population
algorithm.
Simple
principles
few
parameters
characterize
it.
However,
basic
GWO
has
disadvantages,
such
as
difficulty
coordinating
exploration
exploitation
capabilities
premature
convergence.
As
result,
fails
identify
many
irrelevant
redundant
features.
To
improve
performance
algorithm,
this
paper
proposes
velocity-guided
grey
wolf
optimization
with
adaptive
weights
Laplace
operators
(VGWO-AWLO).
Firstly,
by
introducing
uniformly
distributed
dynamic
weighting
mechanism,
control
Language: Английский
Citations
3Computer Modeling in Engineering & Sciences, Journal Year: 2024, Volume and Issue: 140(2), P. 1563 - 1593
Published: Jan. 1, 2024
In classification problems, datasets often contain a large amount of features, but not all them are relevant for accurate classification.In fact, irrelevant features may even hinder accuracy.Feature selection aims to alleviate this issue by minimizing the number in subset while simultaneously error rate.Single-objective optimization approaches employ an evaluation function designed as aggregate with parameter, results obtained depend on value parameter.To eliminate parameter's influence, problem can be reformulated multi-objective problem.The Whale Optimization Algorithm (WOA) is widely used problems because its simplicity and easy implementation.In paper, we propose multi-strategy assisted WOA (MSMOWOA) address feature selection.To enhance algorithm's search ability, integrate multiple strategies such Levy flight, Grey Wolf Optimizer, adaptive mutation into it.Additionally, utilize external repository store non-dominant solution sets grid technology maintain diversity.Results fourteen University California Irvine (UCI) demonstrate that our proposed method effectively removes redundant improves performance.The source code accessed from website: https://github.com/zc0315/MSMOWOA.
Language: Английский
Citations
3Water Resources Management, Journal Year: 2024, Volume and Issue: 38(12), P. 4883 - 4909
Published: June 19, 2024
Language: Английский
Citations
3Journal of Water Process Engineering, Journal Year: 2024, Volume and Issue: 65, P. 105717 - 105717
Published: July 13, 2024
Language: Английский
Citations
3Engineering Optimization, Journal Year: 2025, Volume and Issue: unknown, P. 1 - 36
Published: April 4, 2025
Language: Английский
Citations
0