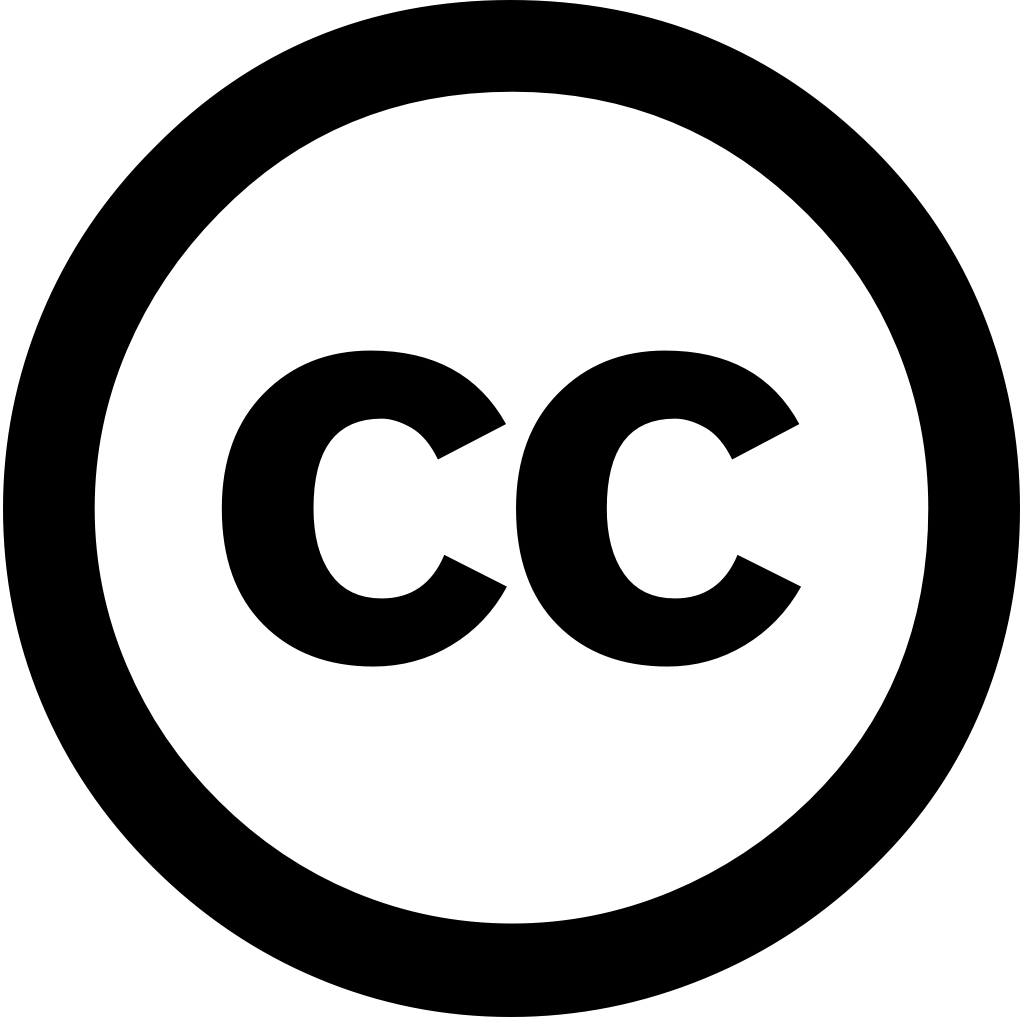
Scientific Reports, Journal Year: 2025, Volume and Issue: 15(1)
Published: March 27, 2025
Language: Английский
Scientific Reports, Journal Year: 2025, Volume and Issue: 15(1)
Published: March 27, 2025
Language: Английский
Cluster Computing, Journal Year: 2024, Volume and Issue: 27(9), P. 13005 - 13027
Published: June 20, 2024
Language: Английский
Citations
3Scientific Reports, Journal Year: 2025, Volume and Issue: 15(1)
Published: Jan. 17, 2025
Drug discovery and development is a challenging time-consuming process. Laboratory experiments conducted on Vidarabine showed IC50 6.97 µg∕mL, 25.78 ˃ 100 µg∕mL against non-small Lung cancer (A-549), Human Melanoma (A-375), epidermoid Skin carcinoma (skin/epidermis) (A-431) respectively. To address these challenges, this paper presents an Artificial Intelligence (AI) model that combines the capabilities of Deep Learning (DL) to identify potential new drug candidates, Fuzzy Rough Set (FRS) theory determine most important chemical compound features, Explainable (XAI) explain features' importance in last layer, medicinal chemistry rediscover anticancer drugs based natural products like Vidarabine. The proposed aims candidates. By analyzing results from laboratory Vidarabine, identifies Sulfur magnesium oxide (MgO) as agents. selected MgO Interpreting their promising further were validate model's predictions. demonstrated that, while was inactive A-431 cell line (IC50 µg∕mL), exhibited significant activity 4.55 17.29 µg/ml respectively). displayed strong A-549 A-375 lines 3.06 1.86 respectively) better than However, weaker two lines. This emphasizes uncovering hidden features may not be discernible without assistance AI. highlights ability AI discover novel compounds with therapeutic potential, which can significantly impact field discovery. by warrants preclinical studies.
Language: Английский
Citations
0Mathematics, Journal Year: 2025, Volume and Issue: 13(4), P. 668 - 668
Published: Feb. 18, 2025
With the rapid development of large model technology, data storage as well collection is very important to improve accuracy training, and Feature Selection (FS) methods can greatly eliminate redundant features in warehouse interpretability model, which makes it particularly field training. In order better reduce warehouses, this paper proposes an enhanced Secretarial Bird Optimization Algorithm (SBOA), called BSFSBOA, by combining three learning strategies. First, for problem insufficient algorithmic population diversity SBOA, best-rand exploration strategy proposed, utilizes randomness optimality random individuals optimal effectively algorithm. Second, address imbalance exploration/exploitation phase segmented balance proposed segmenting population, targeting different natures with degrees exploitation performance, improving quality FS subset when algorithm solved. Finally, performance a four-role strengthens effective ability enhances classification guidance through four population. Subsequently, BSFSBOA-based method applied solve 36 problems involving low, medium, high dimensions, experimental results show that, compared BSFSBOA improves more than 60%, also ranks first feature size, obtains least runtime, confirms that robust efficient solution stability, practicality.
Language: Английский
Citations
0Knowledge-Based Systems, Journal Year: 2025, Volume and Issue: unknown, P. 113286 - 113286
Published: March 1, 2025
Language: Английский
Citations
0Scientific Reports, Journal Year: 2025, Volume and Issue: 15(1)
Published: March 27, 2025
Language: Английский
Citations
0