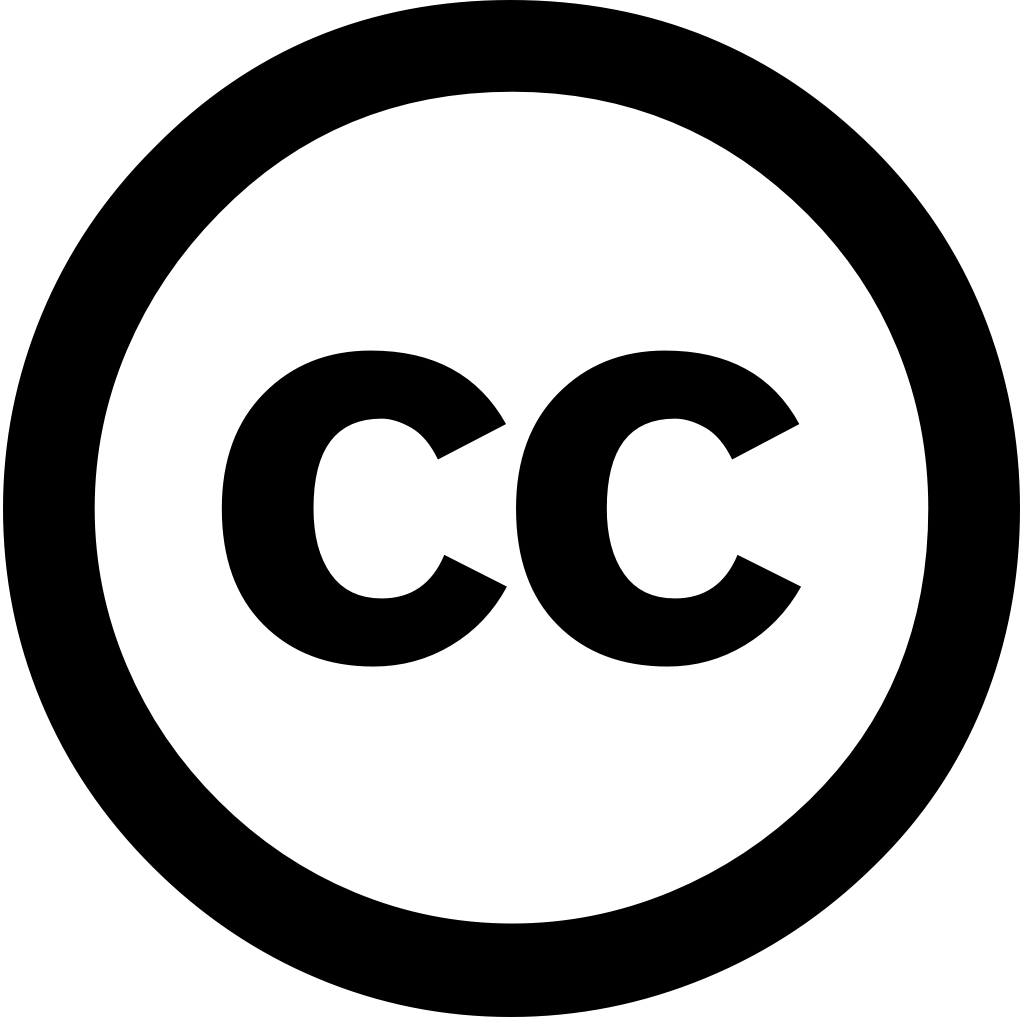
Deleted Journal, Journal Year: 2024, Volume and Issue: 27(1)
Published: Sept. 16, 2024
Language: Английский
Deleted Journal, Journal Year: 2024, Volume and Issue: 27(1)
Published: Sept. 16, 2024
Language: Английский
Knowledge and Information Systems, Journal Year: 2025, Volume and Issue: unknown
Published: May 2, 2025
Language: Английский
Citations
0Transactions on Emerging Telecommunications Technologies, Journal Year: 2025, Volume and Issue: 36(4)
Published: March 20, 2025
ABSTRACT Background Students often need help choosing the right courses to complete their degrees. Course recommender systems assist in selecting suitable academic courses. Recent attention‐based have been developed distinguish influence of past on recommendations. However, these models might not work well when users diverse interests, because effectiveness attention mechanism decreases with variety historical To overcome issues, this study introduces a new approach called Hierarchical Attention Network Deep Learning for Text Forward Harmonic Net (HHFHNet) course recommendations using H‐matrix. Methods Initially, input data obtained from dataset is processed into overview and genres. After that, Term Frequency‐Inverse Document Frequency (TF‐IDF) method applied both query, resulting output fed HHFHNet, which combines Texts (HDLTex) Networks (HAN). This generates Recommendation Probability Value (CRPV), used retrieve recommended Simultaneously, specific genre features are selected chord distance. Then, These CRPV then H‐matrix create ranking‐based Finally, Explainable Artificial Intelligence (XAI) utilized generate recommendation messages based ranking approach. Results The HHFHNet technique was evaluated performance metrics such as precision, recall, F‐measure, it achieved values 90.31%, 91.87%, 91.08%, respectively. Conclusions proposed significantly enhances accuracy offers robust solution guiding students selection.
Language: Английский
Citations
0Deleted Journal, Journal Year: 2024, Volume and Issue: 27(1)
Published: Sept. 16, 2024
Language: Английский
Citations
1