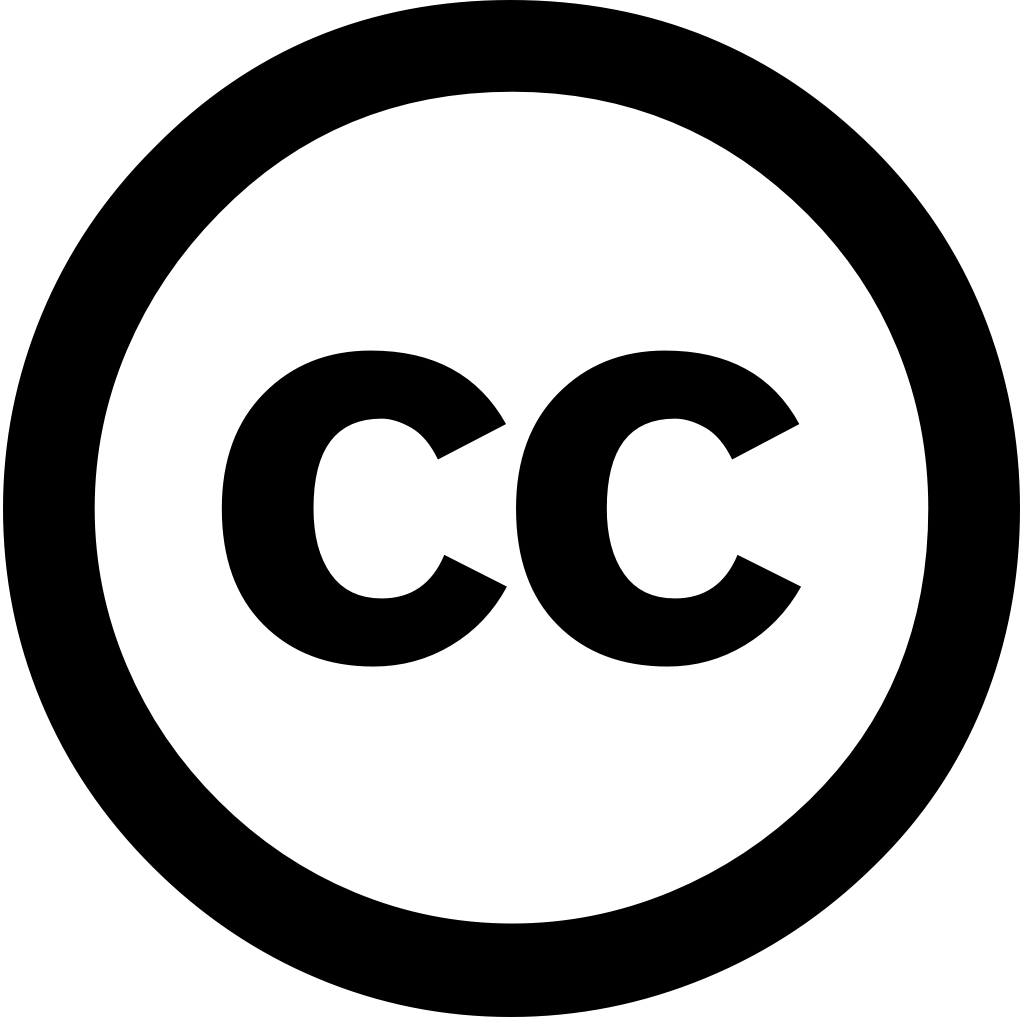
Journal of Engineering Research, Journal Year: 2024, Volume and Issue: unknown
Published: Nov. 1, 2024
Language: Английский
Journal of Engineering Research, Journal Year: 2024, Volume and Issue: unknown
Published: Nov. 1, 2024
Language: Английский
Expert Systems with Applications, Journal Year: 2024, Volume and Issue: unknown, P. 126031 - 126031
Published: Dec. 1, 2024
Language: Английский
Citations
4Diagnostics, Journal Year: 2025, Volume and Issue: 15(2), P. 130 - 130
Published: Jan. 8, 2025
Background: The rapid global spread of the monkeypox virus has led to serious issues for public health professionals. According related studies, and other types skin conditions can through direct contact with infected animals, humans, or contaminated items. This disease cause fever, headaches, muscle aches, enlarged lymph nodes, followed by a rash that develops into lesions. To facilitate early detection monkeypox, researchers have proposed several AI-based techniques accurately classifying identifying condition. However, there is still room improvement detect classify cases. Furthermore, currently pre-trained deep learning models consume extensive resources achieve accurate classification monkeypox. Hence, these often need significant computational power memory. Methods: paper proposes novel lightweight framework called DeepGenMonto various diseases, such as chickenpox, melasma, others. suggested leverages an attention-based convolutional neural network (CNN) genetic algorithm (GA) enhance accuracy while optimizing hyperparameters model. It first applies attention mechanism highlight assign weights specific regions image are relevant model's decision-making process. Next, CNN employed process visual input extract hierarchical features data multiple classes. Finally, CNN's adjusted using robustness accuracy. Compared state-of-the-art (SOTA) models, DeepGenMon design requires significantly lower easier train few parameters. Its effective integration GA further enhances its performance, making it particularly well suited low-resource environments. evaluated on two datasets. dataset comprises 847 images diverse second contains 659 classified categories. Results: model demonstrates superior performance compared SOTA across key evaluation metrics. On 1, achieves precision 0.985, recall 0.984, F-score 0.985. Similarly, 2, attains 0.981, 0.982, 0.982. Moreover, findings demonstrate ability inference time 2.9764 s 1 2.1753 2. Conclusions: These results also show DeepGenMon's effectiveness in different conditions, highlighting potential reliable tool clinical settings.
Language: Английский
Citations
0Computers in Biology and Medicine, Journal Year: 2025, Volume and Issue: 186, P. 109676 - 109676
Published: Jan. 23, 2025
Language: Английский
Citations
0Journal of Ambient Intelligence and Humanized Computing, Journal Year: 2025, Volume and Issue: unknown
Published: Jan. 27, 2025
Language: Английский
Citations
0Biomedical Signal Processing and Control, Journal Year: 2025, Volume and Issue: 107, P. 107819 - 107819
Published: March 12, 2025
Language: Английский
Citations
0Biomedical Signal Processing and Control, Journal Year: 2025, Volume and Issue: 108, P. 107934 - 107934
Published: April 29, 2025
Language: Английский
Citations
0IoT, Journal Year: 2025, Volume and Issue: 6(2), P. 30 - 30
Published: May 16, 2025
Medical image classification often relies on CNNs to capture local details (e.g., lesions, nodules) or transformers model long-range dependencies. However, each paradigm alone is limited in addressing both fine-grained structures and broader anatomical context. We propose ConvTransGFusion, a hybrid that fuses ConvNeXt (for refined convolutional features) Swin Transformer hierarchical global attention) using learnable dual-attention gating mechanism. By aligning spatial dimensions, scaling branch adaptively, applying channel attention, the proposed architecture bridges representations, melding lesion with context essential for accurate diagnosis. Tested four diverse medical imaging datasets—including X-ray, ultrasound, MRI scans—the consistently achieves superior accuracy, precision, recall, F1, AUC over state-of-the-art transformers. Our findings highlight benefits of combining inductive biases transformer-based single framework, positioning ConvTransGFusion as robust versatile solution real-world clinical applications.
Language: Английский
Citations
0Applied Soft Computing, Journal Year: 2024, Volume and Issue: unknown, P. 112553 - 112553
Published: Dec. 1, 2024
Language: Английский
Citations
2Information Fusion, Journal Year: 2024, Volume and Issue: 117, P. 102858 - 102858
Published: Dec. 10, 2024
Language: Английский
Citations
2Applied Data Science and Analysis, Journal Year: 2024, Volume and Issue: 2024, P. 148 - 164
Published: Sept. 8, 2024
Monkeypox is a rather rare viral infectious disease that initially did not receive much attention but has recently become subject of concern from the point view public health. Artificial intelligence (AI) techniques are considered beneficial when it comes to diagnosis and identification through medical big data, including imaging other details patients’ information systems. Therefore, this work performs bibliometric analysis incorporate fields AI bibliometrics discuss trends future research opportunities in Monkeypox. A search over various databases was performed title abstracts articles were reviewed, resulting total 251 articles. After eliminating duplicates irrelevant papers, 108 found be suitable for study. In reviewing these studies, given on who contributed topics or fields, what new appeared time, papers most notable. The main added value outline reader process how conduct correct comprehensive by examining real case study related disease. As result, shows great potential improve diagnostics, treatment, health recommendations connected with Possibly, application can enhance responses outcomes since hasten effective interventions.
Language: Английский
Citations
1