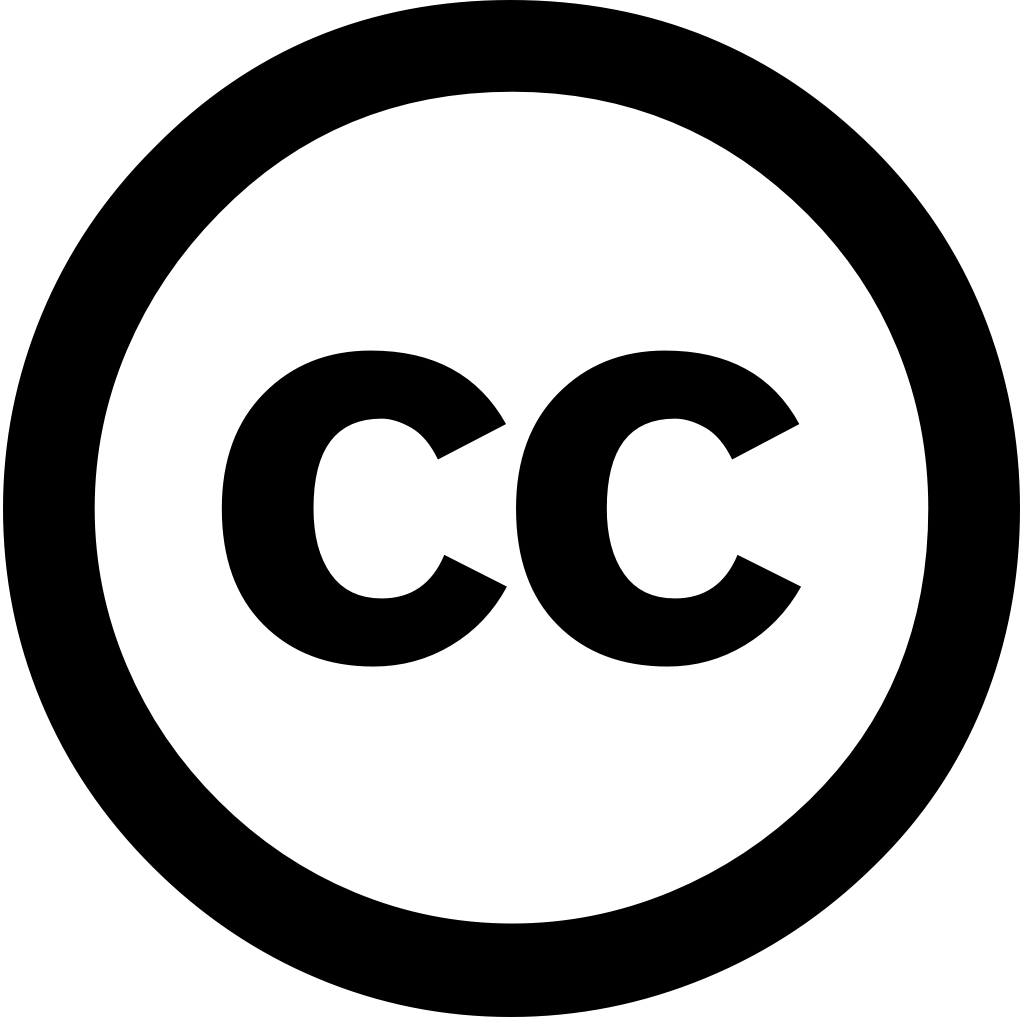
Applied Sciences, Journal Year: 2025, Volume and Issue: 15(2), P. 655 - 655
Published: Jan. 11, 2025
Anomaly prediction in time series is crucial for ensuring the stability and security of data centers, especially scientific contexts such as INFN-CNAF, National Center Research Development Information Communication Technology Institute Nuclear Physics. At large volumes heterogeneous critical to international experiments are managed using dedicated monitoring systems. To ensure continuous availability, artificial intelligence solutions being explored detect anomalies predict potential failures proactively. This work presents a machine learning-based approach automatic anomaly operational metrics INFN-CNAF’s WebDav service. We evaluate several methods, including Long Short-Term Memory, Random Forest, various neural networks, assessing their Accuracy sensitivity distinguishing normal from anomalous behaviors. The results demonstrate effectiveness these not only predicting but also pinpointing areas within monitored metrics. contributes more proactive IT resource enhances center management efficiency.
Language: Английский