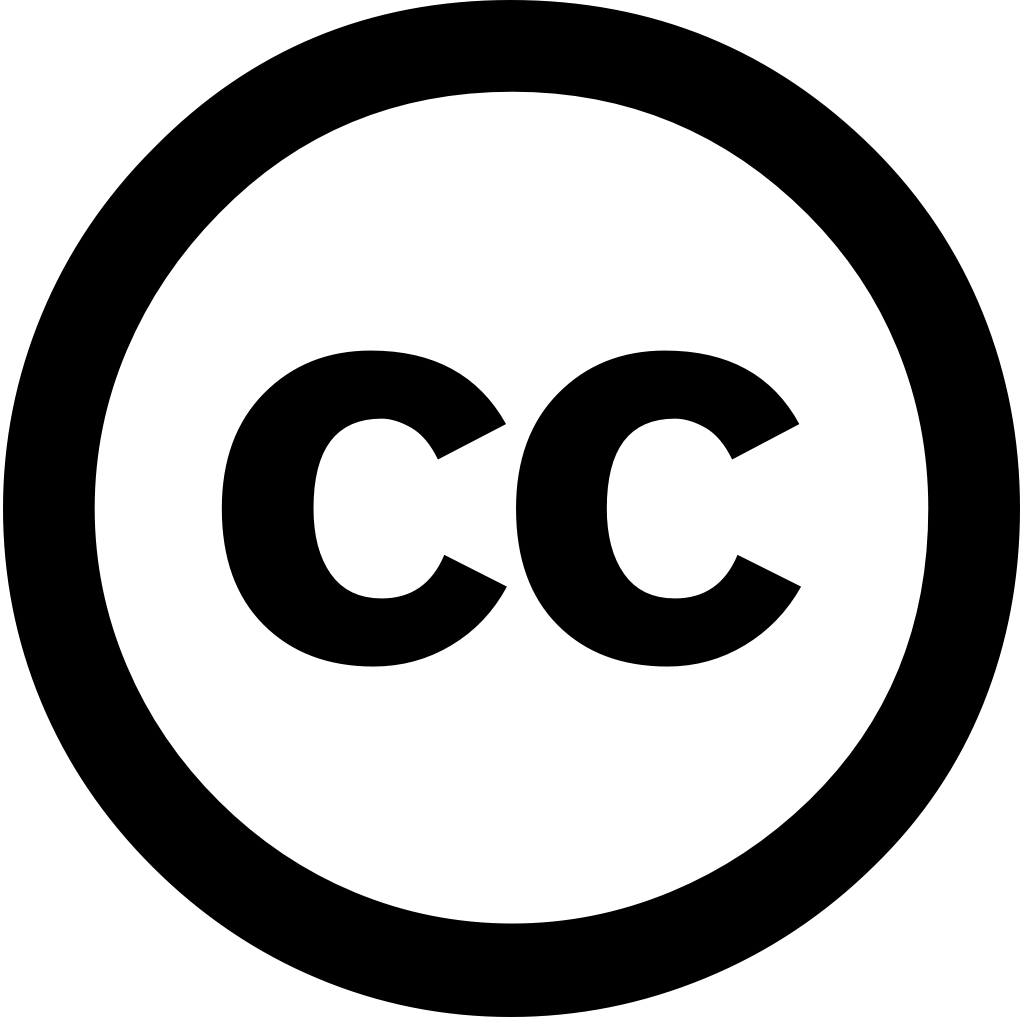
Future Internet, Journal Year: 2025, Volume and Issue: 17(3), P. 107 - 107
Published: March 1, 2025
Integrating deep learning (DL) with the Internet of Medical Things (IoMT) is a paradigm shift in modern healthcare, offering enormous opportunities for patient care, diagnostics, and treatment. Implementing DL IoMT has potential to deliver better diagnosis, treatment, management. However, practical implementation challenges, including data quality, privacy, interoperability, limited computational resources. This survey article provides conceptual framework synthesizes identifies state-of-the-art solutions that tackle challenges current applications DL, analyzes existing limitations future developments. Through an analysis case studies real-world implementations, this work insights into best practices lessons learned, importance robust preprocessing, integration legacy systems, human-centric design. Finally, we outline research directions, emphasizing development transparent, scalable, privacy-preserving models realize full healthcare. aims serve as foundational reference researchers practitioners seeking navigate harness rapidly evolving field.
Language: Английский