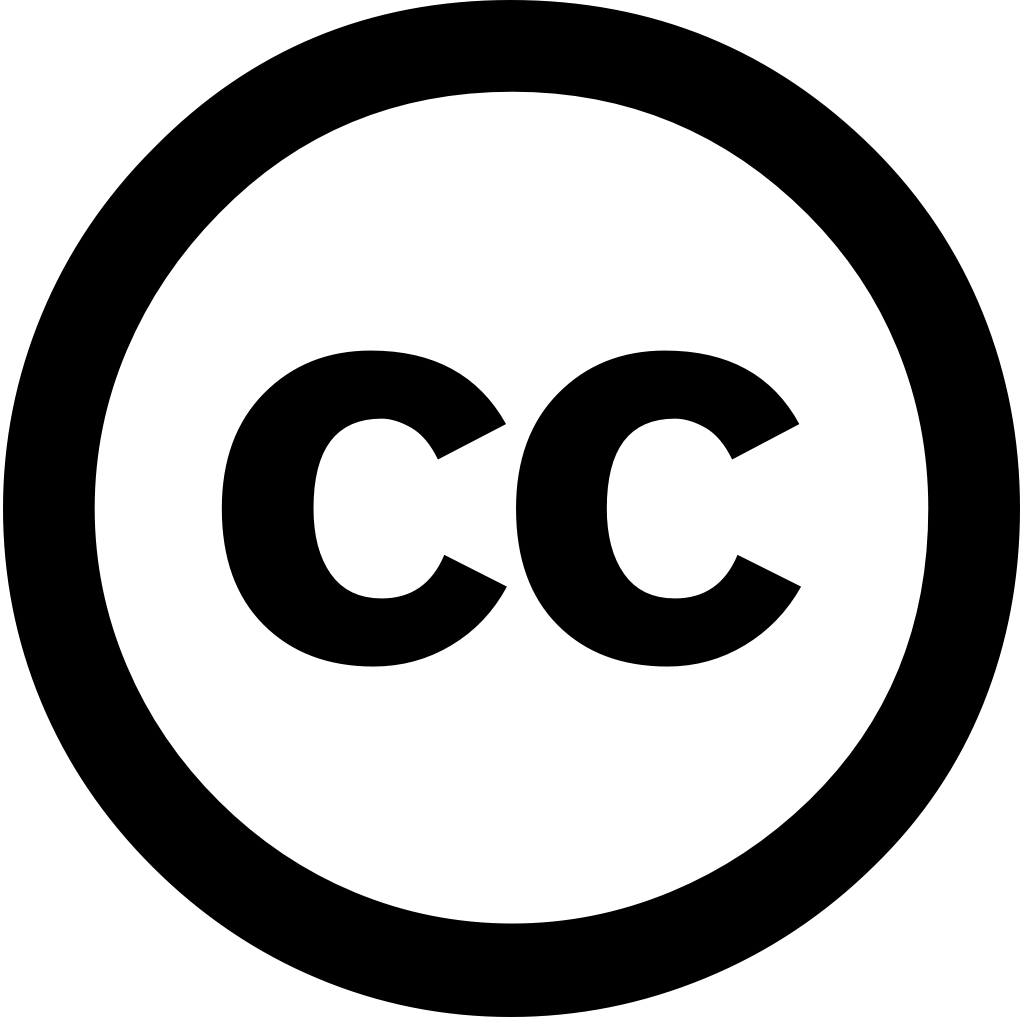
Agronomy, Journal Year: 2025, Volume and Issue: 15(5), P. 1170 - 1170
Published: May 11, 2025
Accurate segmentation of soil pore structure is crucial for studying water migration, nutrient cycling, and gas exchange. However, the low-contrast high-noise CT images in complex environments cause traditional methods to have obvious deficiencies accuracy robustness. This paper proposes a hybrid model combining Multi-Modal Low-Frequency Reconstruction algorithm (MMLFR) UNet (MMLFR-UNet). MMLFR enhances key feature expression by extracting image low-frequency signals suppressing noise interference through multi-scale spectral decomposition, whereas excels detail restoration complexity boundary processing virtue its coding-decoding hopping connection mechanism. In this paper, an undisturbed column was collected Hainan Province, China, which classified as Ferralsols (FAO/UNESCO), scans were utilized acquire high-resolution generate high-quality datasets suitable deep learning preprocessing operations such fixed-layer sampling, cropping, enhancement. The results show that MMLFR-UNet outperforms (e.g., Otsu Fuzzy C-Means (FCM)) terms Intersection over Union (IoU), Dice Similarity Coefficients (DSC), Pixel Accuracy (PA), similarity. Notably, exhibits exceptional robustness precision tasks involving structures images.
Language: Английский