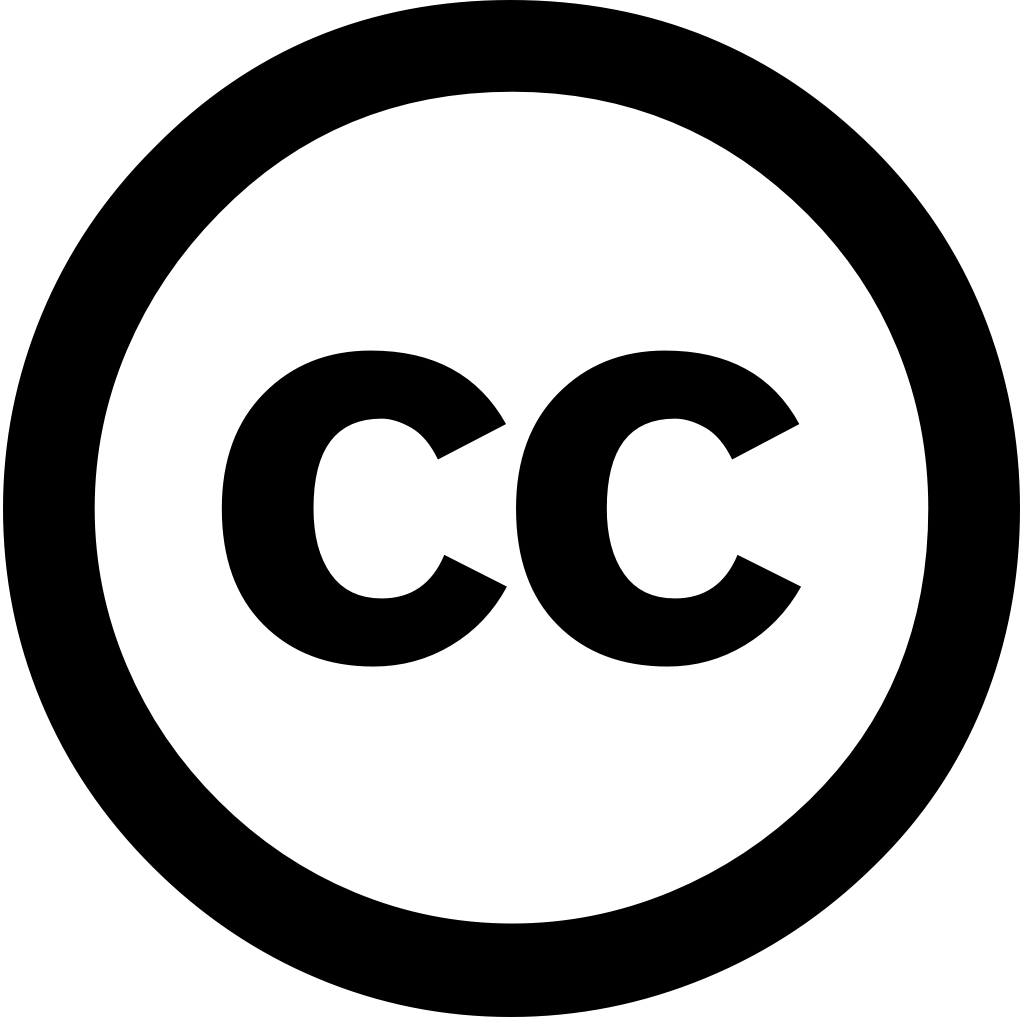
Heliyon, Journal Year: 2024, Volume and Issue: 10(18), P. e36940 - e36940
Published: Aug. 28, 2024
Language: Английский
Heliyon, Journal Year: 2024, Volume and Issue: 10(18), P. e36940 - e36940
Published: Aug. 28, 2024
Language: Английский
Environmental Science and Pollution Research, Journal Year: 2024, Volume and Issue: 31(10), P. 14503 - 14536
Published: Feb. 2, 2024
Language: Английский
Citations
6Journal of Water Process Engineering, Journal Year: 2024, Volume and Issue: 61, P. 105306 - 105306
Published: April 16, 2024
Language: Английский
Citations
6Energy, Journal Year: 2022, Volume and Issue: 262, P. 125536 - 125536
Published: Sept. 22, 2022
Language: Английский
Citations
28Water, Journal Year: 2022, Volume and Issue: 14(19), P. 3147 - 3147
Published: Oct. 6, 2022
Environmental sensors are utilized to collect real-time data that can be viewed and interpreted using a visual format supported by server. Machine learning (ML) methods, on the other hand, excellent in statistically evaluating complicated nonlinear systems assist modeling prediction. Moreover, it is important implement precise online monitoring of complex wastewater treatment plants increase stability. Thus, this study, novel approach based ML methods suggested predict effluent concentration total nitrogen (TNeff) few hours ahead. The method consists different algorithms training stage, best selected models concatenated prediction stage. Recursive feature elimination reduce overfitting curse dimensionality finding eliminating irrelevant features identifying optimal subset features. Performance indicators multi-attention-based recurrent neural network partial least squares had highest accurate performance, representing 41% improvement over methods. Then, proposed was assessed with multistep horizons. It predicted 1-h ahead TNeff 98.1% accuracy rate, whereas 3-h TN 96.3% rate.
Language: Английский
Citations
27Environmental Research, Journal Year: 2023, Volume and Issue: 224, P. 115549 - 115549
Published: Feb. 22, 2023
Language: Английский
Citations
14Journal of Water Process Engineering, Journal Year: 2023, Volume and Issue: 54, P. 103949 - 103949
Published: June 30, 2023
Language: Английский
Citations
14Environmental Science and Pollution Research, Journal Year: 2024, Volume and Issue: 31(10), P. 14610 - 14640
Published: Jan. 26, 2024
Language: Английский
Citations
5Journal of Environmental Management, Journal Year: 2024, Volume and Issue: 354, P. 120324 - 120324
Published: Feb. 15, 2024
Language: Английский
Citations
5Mathematics, Journal Year: 2024, Volume and Issue: 12(5), P. 627 - 627
Published: Feb. 20, 2024
The concentration of ammonia nitrogen is significant for intensive aquaculture, and if the too high, it will seriously affect survival state aquaculture. Therefore, prediction control in advance essential. This paper proposed a combined model based on X Adaptive Boosting (XAdaBoost) Long Short-Term Memory neural network (LSTM) to predict mariculture. Firstly, weight assignment strategy was improved, number correction iterations introduced retard shortcomings data error accumulation caused by AdaBoost basic algorithm. Then, XAdaBoost algorithm generated several LSTM su-models concentration. Finally, there were two experiments conducted verify effectiveness model. In experiment, compared with other comparison models, RMSE XAdaBoost–LSTM reduced about 0.89–2.82%, MAE 0.72–2.47%, MAPE 8.69–18.39%. stability RMSE, MAE, decreased 1–1.5%, 0.7–1.7%, 7–14%. From these experiments, evaluation indexes superior which proves that has good accuracy lays foundation monitoring regulating change future.
Language: Английский
Citations
5Desalination, Journal Year: 2024, Volume and Issue: 592, P. 118092 - 118092
Published: Sept. 7, 2024
Language: Английский
Citations
5