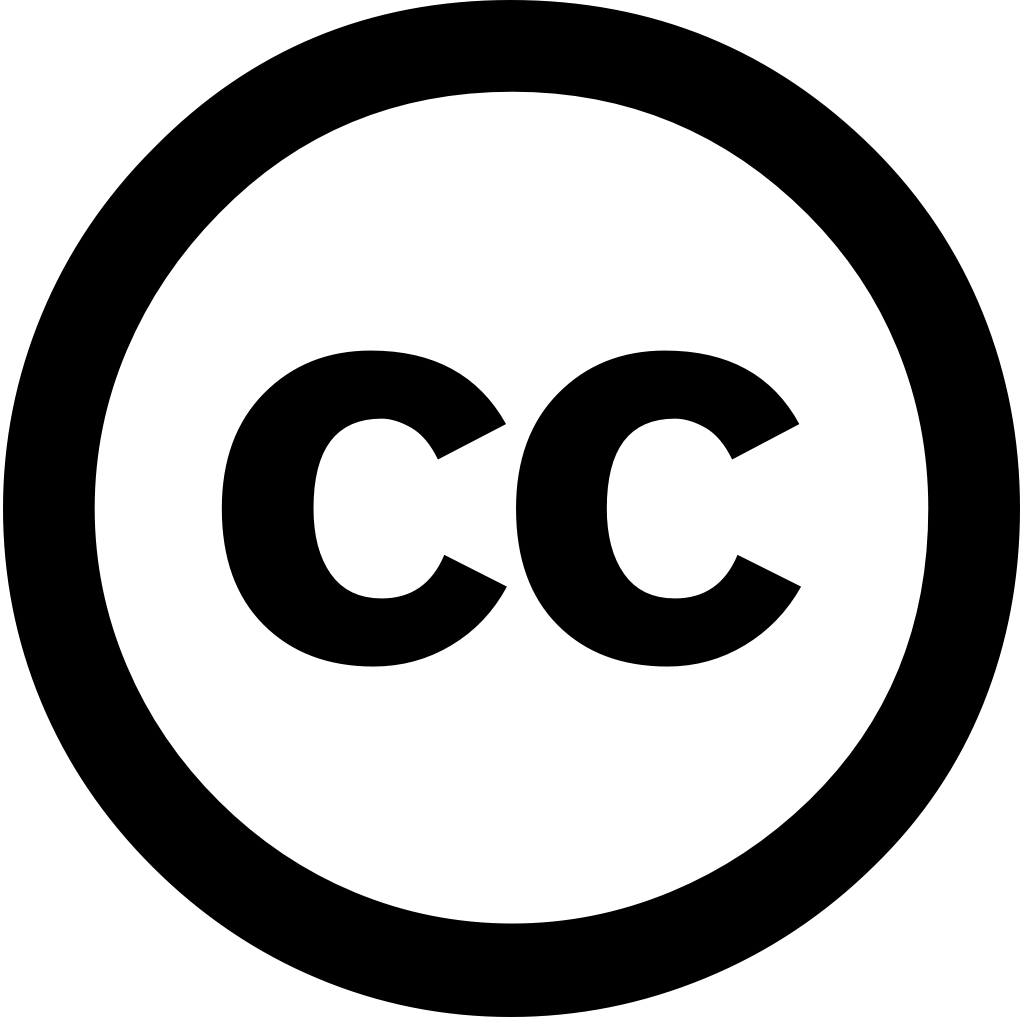
Frontiers in Pediatrics, Journal Year: 2025, Volume and Issue: 13
Published: April 17, 2025
Artificial Intelligence is revolutionizing prenatal diagnostics by enhancing the accuracy and efficiency of procedures. This review explores AI machine learning (ML) in early detection, prediction, assessment neural tube defects (NTDs) through ultrasound imaging. Recent studies highlight effectiveness techniques, such as convolutional networks (CNNs) support vector machines (SVMs), achieving detection rates up to 95% across various datasets, including fetal images, genetic data, maternal health records. SVM models have demonstrated 71.50% on training datasets 68.57% testing for NTD classification, while advanced deep (DL) methods report patient-level prediction 94.5% an area under receiver operating characteristic curve (AUROC) 99.3%. integration with genomic analysis has identified key biomarkers associated NTDs, Growth Associated Protein 43 (GAP43) Glial Fibrillary Acidic (GFAP), logistic regression 86.67% accuracy. Current AI-assisted technologies improved diagnostic accuracy, yielding sensitivity specificity 88.9% 98.0%, respectively, compared traditional 81.5% 92.2% specificity. systems also streamlined workflows, reducing median scan times from 19.7 min 11.4 min, allowing sonographers prioritize critical patient care. Advancements DL algorithms, Oct-U-Net PAICS, achieved recall precision 0.93 0.96, identifying abnormalities. Moreover, AI's evolving role research supports personalized prevention strategies enhances public awareness AI-generated messages. In conclusion, significantly improves leading greater As continues advance, it potential further enhance healthcare raise about ultimately contributing better outcomes.
Language: Английский