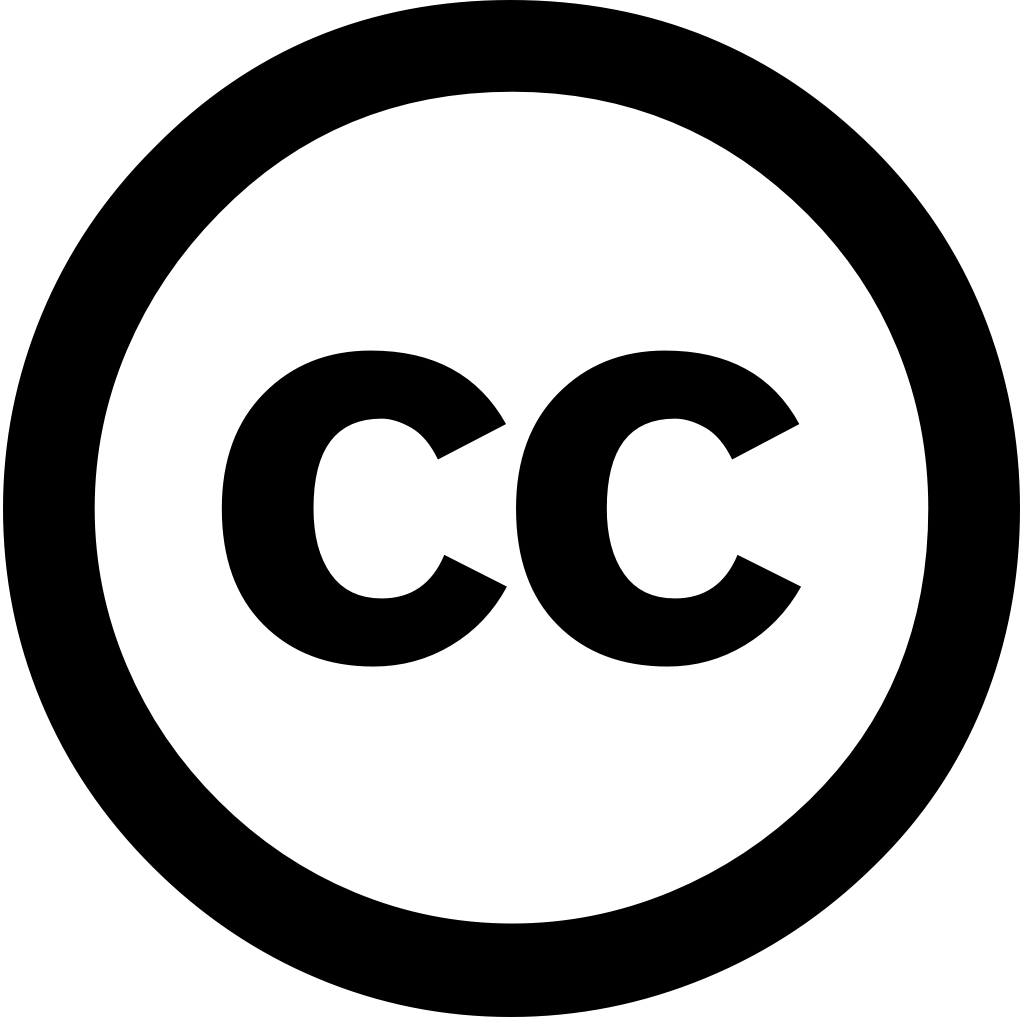
Ecotoxicology and Environmental Safety, Journal Year: 2024, Volume and Issue: 290, P. 117548 - 117548
Published: Dec. 16, 2024
Language: Английский
Ecotoxicology and Environmental Safety, Journal Year: 2024, Volume and Issue: 290, P. 117548 - 117548
Published: Dec. 16, 2024
Language: Английский
Computers and Electronics in Agriculture, Journal Year: 2025, Volume and Issue: 232, P. 110120 - 110120
Published: Feb. 24, 2025
Language: Английский
Citations
1Computers and Electronics in Agriculture, Journal Year: 2024, Volume and Issue: 227, P. 109565 - 109565
Published: Oct. 24, 2024
Language: Английский
Citations
6Plants, Journal Year: 2024, Volume and Issue: 13(1), P. 140 - 140
Published: Jan. 4, 2024
Nitrogen is a fundamental component for building amino acids and proteins, playing crucial role in the growth development of plants. Leaf nitrogen concentration (LNC) serves as key indicator assessing plant development. Monitoring LNC provides insights into absorption utilization from soil, offering valuable information rational nutrient management. This, turn, contributes to optimizing supply, enhancing crop yields, minimizing adverse environmental impacts. Efficient non-destructive estimation paramount importance on-field Spectral technology, with its advantages repeatability high-throughput observations, feasible method obtaining data. This study explores responsiveness spectral parameters soybean at different vertical scales, aiming refine management soybeans. research collected hyperspectral reflectance data leaf layers Three types parameters, nitrogen-sensitive empirical indices, randomly combined dual-band “three-edge” were calculated. Four optimal index selection strategies constructed based on correlation coefficients between each layer. These included combinations (Combination 1), 2), parameter 3), mixed combination 4). Subsequently, these four used input variables build models soybeans scales using partial least squares regression (PLSR), random forest (RF), backpropagation neural network (BPNN). The results demonstrated that reached highest values upper leaves, most showing significant correlations (p < 0.05). Notably, reciprocal difference (VI6) exhibited upper-layer 0.732, wavelength 841 nm 842 nm. In constructing layers, accuracy gradually improved increasing height layer best performance, validation set coefficient determination (R2) was higher by 9.9% 16.0% compared other layers. RF estimating LNC, R2 6.2% 8.8% models. RMSE lower 2.1% 7.0%, MRE 4.7% 5.6% Among combinations, Combination 4 achieved accuracy, 2.3% 13.7%. conclusion, employing input, model 0.856, 0.551, 10.405%. findings this provide technical support remote sensing monitoring LNCs spatial scales.
Language: Английский
Citations
5Plants, Journal Year: 2024, Volume and Issue: 13(6), P. 773 - 773
Published: March 8, 2024
Smooth bromegrass (Bromus inermis) is a perennial, high-quality forage grass. However, its seed yield influenced by agronomic practices, climatic conditions, and the growing year. The rapid effective prediction of can assist growers in making informed production decisions reducing agricultural risks. Our field trial design followed completely randomized block with four blocks three nitrogen levels (0, 100, 200 kg·N·ha−1) during 2022 2023. Data on remote vegetation index (RVI), normalized difference (NDVI), leaf content (LNC), area (LAI) were collected at heading, anthesis, milk stages. Multiple linear regression (MLR), support vector machine (SVM), random forest (RF) models utilized to predict yield. In 2022, results indicated that application provided sufficiently large range variation (ranging from 45.79 379.45 kg ha⁻¹). Correlation analysis showed indices RVI, NDVI, LNC, LAI presented significant positive correlation yield, highest coefficient was observed heading stage. data formulate predictive model for suggested utilizing stage produced best performance. SVM RF outperformed MLR prediction, demonstrating performance (R2 = 0.75, RMSE 51.93 ha−1, MAE 29.43 MAPE 0.17). Notably, accuracy predicting year 2023 using this had decreased. Feature importance revealed LNC crucial indicator smooth Further studies an expanded dataset integration weather are needed improve generalizability adaptability
Language: Английский
Citations
4Agronomy, Journal Year: 2024, Volume and Issue: 14(6), P. 1248 - 1248
Published: June 9, 2024
Efficiently obtaining leaf nitrogen content (LNC) in rice to monitor the nutritional health status is crucial achieving precision fertilization on demand. Unmanned aerial vehicle (UAV)-based hyperspectral technology an important tool for determining LNC. However, intricate coupling between spectral information and remains elusive. To address this, this study proposed estimation method LNC that integrates hybrid preferred features with deep learning modeling algorithms based UAV imagery. The approach leverages XGBoost, Pearson correlation coefficient (PCC), a synergistic combination of both identify characteristic variables estimation. We then construct models using statistical regression methods (partial least-squares (PLSR)) machine (random forest (RF); neural networks (DNN)). optimal model utilized map spatial distribution at field scale. was conducted National Agricultural Science Technology Park, Guangzhou, located Baiyun District Guangdong, China. results reveal combined PCC-XGBoost algorithm significantly enhances accuracy inversion compared standalone screening approach. Notably, built DNN exhibits highest predictive performance demonstrates great potential mapping This indicates role enhancement utilization efficiency cultivation. outcomes offer valuable reference enhancing agricultural practices sustainable crop management.
Language: Английский
Citations
4Crop Protection, Journal Year: 2024, Volume and Issue: 184, P. 106805 - 106805
Published: June 11, 2024
Language: Английский
Citations
4Agronomy, Journal Year: 2025, Volume and Issue: 15(1), P. 175 - 175
Published: Jan. 13, 2025
In agricultural production, the nitrogen content of sugarcane is assessed with precision and economy, which crucial for balancing fertilizer application, reducing resource waste, minimizing environmental pollution. As an important economic crop, productivity significantly influenced by various factors, especially supply. Traditional methods based on manually extracted image features are not only costly but also limited in accuracy generalization ability. To address these issues, a novel regression prediction model estimating sugarcane, named SC-ResNeXt (Enhanced Self-Attention, Spatial Attention, Channel Attention ResNeXt), has been proposed this study. The Self-Attention (SA) mechanism Convolutional Block Module (CBAM) have incorporated into ResNeXt101 to enhance model’s focus key its information extraction capability. It was demonstrated that achieved test R2 value 93.49% predicting leaves. After introducing SA CBAM attention mechanisms, improved 4.02%. Compared four classical deep learning algorithms, exhibited superior performance. This study utilized images captured smartphones combined automatic feature technologies, achieving precise economical predictions compared traditional laboratory chemical analysis methods. approach offers affordable technical solution small farmers optimize management plants, potentially leading yield improvements. Additionally, it supports development more intelligent farming practices providing predictions.
Language: Английский
Citations
0Computers and Electronics in Agriculture, Journal Year: 2025, Volume and Issue: 234, P. 110213 - 110213
Published: March 12, 2025
Language: Английский
Citations
0Smart Agricultural Technology, Journal Year: 2025, Volume and Issue: unknown, P. 100908 - 100908
Published: March 1, 2025
Language: Английский
Citations
0European Journal of Agronomy, Journal Year: 2025, Volume and Issue: 168, P. 127653 - 127653
Published: April 24, 2025
Language: Английский
Citations
0