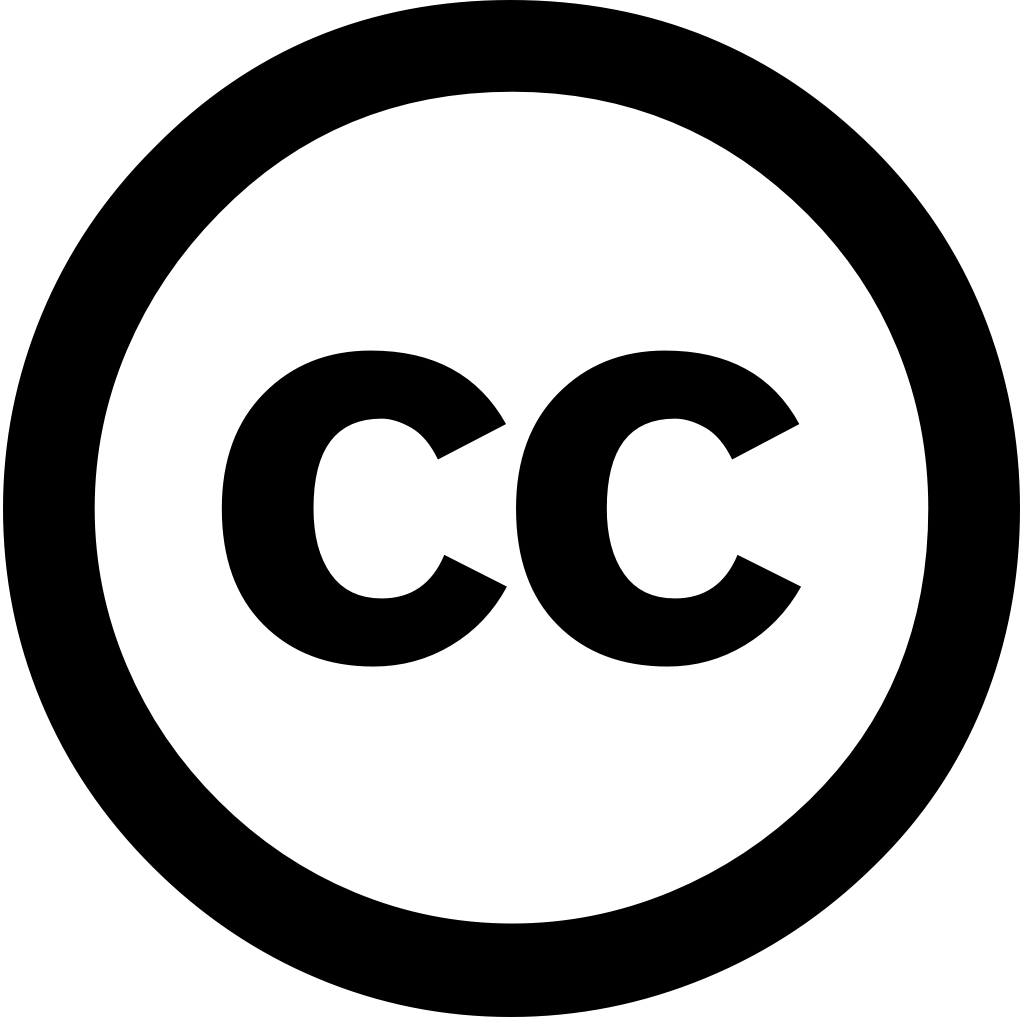
Ecotoxicology and Environmental Safety, Journal Year: 2024, Volume and Issue: 290, P. 117548 - 117548
Published: Dec. 16, 2024
Language: Английский
Ecotoxicology and Environmental Safety, Journal Year: 2024, Volume and Issue: 290, P. 117548 - 117548
Published: Dec. 16, 2024
Language: Английский
The Crop Journal, Journal Year: 2025, Volume and Issue: unknown
Published: April 1, 2025
Language: Английский
Citations
0Foods, Journal Year: 2025, Volume and Issue: 14(9), P. 1551 - 1551
Published: April 28, 2025
The distribution of moisture content in stacked tea leaves during processing significantly influences quality. Visualizing this is crucial for optimizing parameters. In study, we utilized hyperspectral imaging (HSI) technology combined with machine learning algorithms to evaluate the and its West Lake Longjing Tencha green products flow line. A spectral quantitative determination model was developed, achieving high accuracy (Rp2 > 0.940) demonstrated strong generalization ability, allowing it predict both types tea. Through imaging, visualized seven key steps observed that non-uniform, leaf tips petioles having higher levels than surface. This study offers a novel solution real-time monitoring production, ensuring consistent product Future research could focus on refining transfer techniques exploring additional varieties further enhance approach.
Language: Английский
Citations
0Agriculture, Journal Year: 2024, Volume and Issue: 14(10), P. 1797 - 1797
Published: Oct. 12, 2024
Plant nitrogen concentration (PNC) is a key indicator reflecting the growth and development status of plants. The timely accurate monitoring plant PNC great significance for refined management crop nutrition in field. rapidly developing sensor technology provides powerful means PNC. Although RGB images have rich spatial information, they lack spectral information red edge near infrared bands, which are more sensitive to vegetation. Conversely, multispectral offer superior resolution but typically lag detail compared images. Therefore, purpose this study improve accuracy efficiency by combining advantages through image-fusion technology. This was based on booting, heading, early-filling stages winter wheat, synchronously acquiring UAV MS data, using Gram–Schmidt (GS) principal component (PC) methods generate fused evaluate them with multiple image-quality indicators. Subsequently, models predicting wheat were constructed machine-selection algorithms such as RF, GPR, XGB. results show that RGB_B1 image contains richer details other bands. GS method PC method, performance fusing high-resolution band optimal. After fusion, correlation between vegetation indices (VIs) has been enhanced varying degrees different periods, significantly enhancing response ability To comprehensively assess potential estimating PNC, fully before after fusion machine learning Random Forest (RF), Gaussian Process Regression (GPR), eXtreme Gradient Boosting (XGB). model established high stability single period, varieties, treatments, making it better than image. most significant enhancements during booting stages, particularly RF algorithm, achieved an 18.8% increase R2, 26.5% RPD, 19.7% decrease RMSE. effective technical dynamic nutritional strong support precise nutrition.
Language: Английский
Citations
3Frontiers in Plant Science, Journal Year: 2024, Volume and Issue: 15
Published: June 7, 2024
Introduction The use of chemical fertilizers in rice field management directly affects yield. Traditional cultivation often relies on the experience farmers to develop fertilization plans, which cannot be adjusted according fertilizer requirements rice. At present, agricultural drones are widely used for early monitoring rice, but due their lack rationality, they guide fertilization. How accurately apply nitrogen during tillering stage stabilize yield is an urgent problem solved current large-scale production process. Methods WOFOST a highly mechanistic crop growth model that can effectively simulate effects and development. However, its spatial heterogeneity, ability at level weak. This study based UAV remote sensing obtain hyperspectral data canopy assimilation with model, decision-making method application stage. Extracting features using Continuous Projection Algorithm constructing inversion biomass Extreme Learning Machine. By two methods, Ensemble Kalman Filter Four-Dimensional Variational, inverted localized were assimilated, simulation results corrected. With average as goal, formulate decisions create prescription map achieve precise Results research indicate training set R 2 RMSE 0.953 0.076, respectively, while testing 0.914 0.110, respectively. When obtaining same yield, strategy ENKF applied less fertilizer, reducing 5.9% compared standard scheme. Discussion enhances rationality unmanned aerial vehicle machines through assimilation, providing new theoretical basis
Language: Английский
Citations
1Agronomy, Journal Year: 2024, Volume and Issue: 14(12), P. 2760 - 2760
Published: Nov. 21, 2024
Leaf nitrogen content (LNC) is a vital agronomic parameter in rice, commonly used to evaluate photosynthetic capacity and diagnose crop nutrient levels. Nitrogen deficiency can significantly reduce yield, underscoring the importance of accurate LNC estimation for practical applications. This study utilizes hyperspectral UAV imagery acquire rice canopy data, applying various machine learning regression algorithms (MLR) develop an model create concentration distribution map, offering valuable guidance subsequent field management. The analysis incorporates four types spectral data extracted throughout growth cycle: original reflectance bands (OR bands), vegetation indices (VIs), first-derivative (FD variable parameters (HSPs) as inputs, while measured serves output. Results demonstrate that random forest (RFR) gradient boosting decision tree (GBDT) performed effectively, with GBDT achieving highest average R2 0.76 across different treatments. Among models varieties, RFR exhibited superior accuracy, 0.95 SuXiangJing100 variety, reached 0.93. Meanwhile, support vector (SVMR) showed slightly lower partial least-squares (PLSR) was least effective. developed method applicable whole stage common varieties. suitable estimating stages, treatments, it also provides reference fertilization planning at flight altitudes other than 120 m this study.
Language: Английский
Citations
1Remote Sensing, Journal Year: 2024, Volume and Issue: 16(23), P. 4479 - 4479
Published: Nov. 29, 2024
Nitrogen is the main nutrient element in growth process of white radish, and accurate monitoring radish leaf nitrogen content (LNC) an important guide for precise fertilization decisions field. Using LNC as object, research on hyperspectral estimation methods was carried out based field sample data at multiple stages using feature selection integrated learning algorithm models. First, Vegetation Index (VI) constructed from data. We extracted sensitive features VI response to Pearson’s feature-selection approach. Second, a stacking-integrated approach proposed machine algorithms such Support Vector Machine (SVM), Random Forest (RF), Ridge K-Nearest Neighbor (KNN) base model first layer architecture, Lasso meta-model second realize LNC. The analysis results show following: (1) bands are mainly centered around 600–700 nm 1950 nm, VIs also concentrated this band range. (2) Stacking with spectral inputs achieved good prediction accuracy leaf, R2 = 0.7, MAE 0.16, MSE 0.05 estimated over whole stage radish. (3) variable filtering function chosen meta-model, which has redundant model-selection effect helps improve quality framework. This study demonstrates potential method stages.
Language: Английский
Citations
1Agronomy, Journal Year: 2024, Volume and Issue: 15(1), P. 38 - 38
Published: Dec. 27, 2024
One of the most important nutrients needed for fruit tree growth is nitrogen. For orchards to get targeted, well-informed nitrogen fertilizer, accurate, large-scale, real-time monitoring, and assessment nutrition essential. This study examines Leaf Nitrogen Content (LNC) custard apple tree, a noteworthy that extensively grown in China’s Yunnan Province. uses an ensemble learning technique based on multiple machine algorithms effectively precisely monitor leaf content canopy using multispectral footage trees taken via Unmanned Aerial Vehicle (UAV) across different phases. First, shadows background noise from soil are removed UAV imagery by spectral shadow indices The noise-filtered then used extract number vegetation (VIs) textural features (TFs). Correlation analysis determine which pertinent LNC estimation. A two-layer model built quantitatively estimate stacking (Stacking) principles. Random Forest (RF), Adaptive Boosting (ADA), Gradient Decision Trees (GBDT), Linear Regression (LR), Extremely Randomized (ERT) among basis estimators integrated first layer. By detecting eliminating redundancy base estimators, Least Absolute Shrinkage Selection Operator regression (Lasso)model second layer improves According results, Lasso successfully finds redundant suggested approach, yields maximum estimation accuracy trees’ leaves. With root mean square error (RMSE) 0.059 absolute (MAE) 0.193, coefficient determination (R2) came 0. 661. significant potential UAV-based techniques tracking leaves highlighted this work. Additionally, approaches investigated might offer insightful information point reference remote sensing applications monitoring other crops.
Language: Английский
Citations
1Remote Sensing, Journal Year: 2024, Volume and Issue: 16(12), P. 2190 - 2190
Published: June 17, 2024
Leaf chlorophyll content (LCC) is an important indicator of plant nutritional status and can be a guide for disease diagnosis. In this study, we took apple leaves infected with mosaic as research object extracted two types information on spectral textural features from hyperspectral images, view to realizing non-destructive detection LCC. First, the collected images were preprocessed reflectance was in region interest. Subsequently, used successive projections algorithm (SPA) select optimal wavelengths (OWs) eight basic using gray-level co-occurrence matrix (GLCM). addition, composite metrics, including vegetation indices (VIs), normalized difference texture (NDTIs), (DTIs), ratio (RTIs) calculated. Third, applied maximal coefficient (MIC) significant VIs textures, well tandem method fuse features. Finally, employ support vector regression (SVR), backpropagation neural network (BPNN), K-nearest neighbors (KNNR) methods explore efficacy single combined feature models estimating The results showed that model (R2 = 0.8532, RMSE 2.1444, RPD 2.6179) NDTIs 0.7927, 2.7453, 2.2032) achieved best among spectra texture, respectively. However, generally exhibit inferior performance compared are unsuitable standalone applications. Combining potentially improve models. Specifically, when combining input parameters, three machine learning outperform model. Ultimately, SVR achieves highest LCC 0.8665, 1.8871, 2.7454). This study reveals improves quantitative disease, leading higher estimation accuracy.
Language: Английский
Citations
1Journal of Agriculture and Food Research, Journal Year: 2024, Volume and Issue: 18, P. 101208 - 101208
Published: July 6, 2024
Nitrogen is the most important nutritional element during vegetative growth phase of pineapple crop; however, its presence in soil insufficient to meet plant demands. In this study, nine machine learning techniques were validated estimate total nitrogen (TN) content MD2 crops from data multiple sources. These sources included multispectral images captured by an unmanned aerial vehicle (UAV); situ sensors, which collected information on ecological factors such as pH, temperature, solar radiation, relative humidity, moisture, wind speed and direction, well SPAD values indicating leaf chlorophyll content. Total taken tissue samples, then analyzed a laboratory. To introduce variability, complete randomized block experimental design was implemented, applying five different treatments blocks, each with 12 replications, 6-month period crop located Tauramena, Colombia. address inherent variability agricultural environmental data, dimensionality reduced using Principal Component Analysis (PCA). addition, regularization applied, including cross-validation, feature selection, boost methods, L1 (Lasso) L2 (Ridge) regularization, hyperparameter optimization. strategies generated more robust accurate models, multilayer perceptron regressor (MLP regressor) extreme gradient boosting (XGBoost) algorithms standing out. On first sampling date, XGBoost achieved R2 86.98 %, being highest. following dates, MLP 59.11 % second date; 68.00 third last 69.4 %. results indicate that integration use models could greatly improve precision nitro-gen (N) diagnostics crops, especially real-time applications. findings highlight promising potential developing integrate multisensor fusion for various applications agriculture.
Language: Английский
Citations
1International Journal of Remote Sensing, Journal Year: 2024, Volume and Issue: unknown, P. 1 - 28
Published: Oct. 3, 2024
Language: Английский
Citations
1