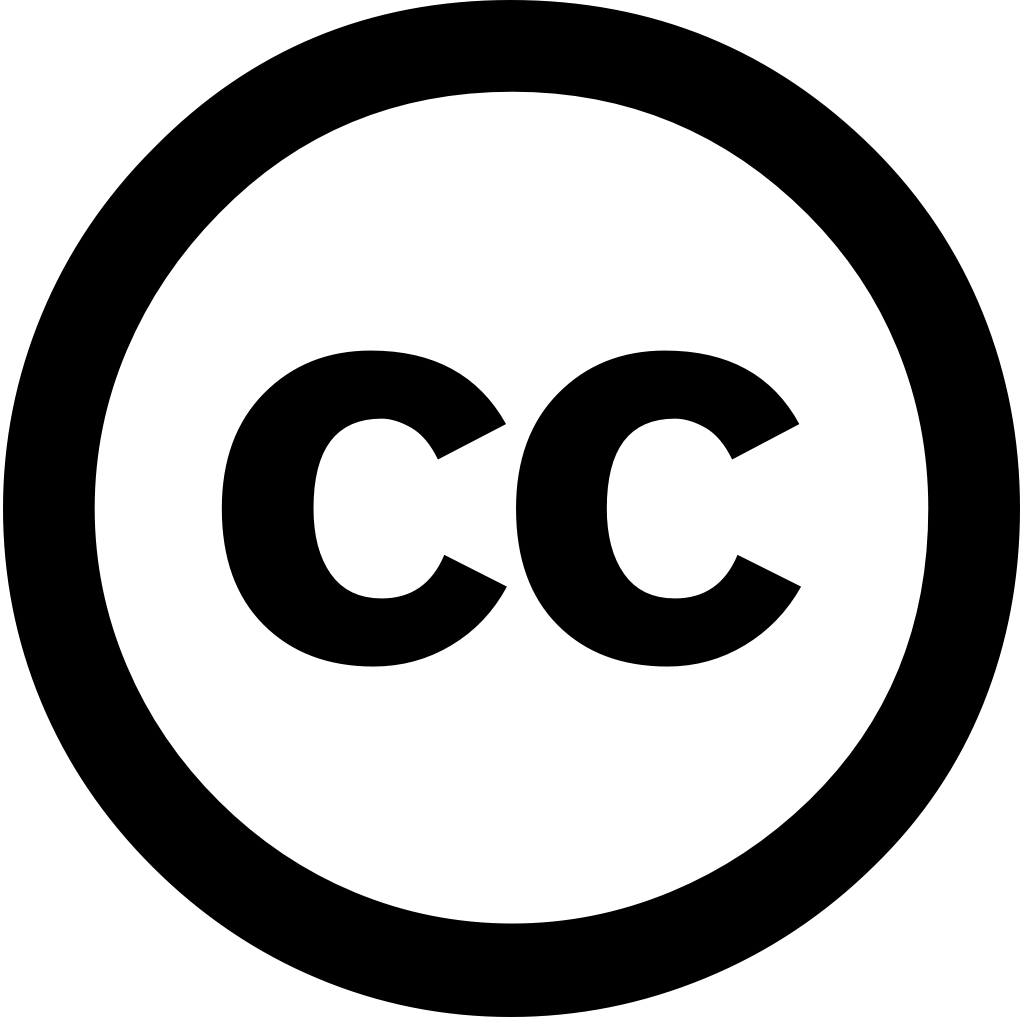
European Journal of Agronomy, Journal Year: 2024, Volume and Issue: 164, P. 127461 - 127461
Published: Dec. 9, 2024
Language: Английский
European Journal of Agronomy, Journal Year: 2024, Volume and Issue: 164, P. 127461 - 127461
Published: Dec. 9, 2024
Language: Английский
Crop Science, Journal Year: 2025, Volume and Issue: 65(1)
Published: Jan. 1, 2025
Abstract Variety testing programs (VTPs) use multi‐environment trials (MET) to evaluate and report the performance of commercially available pre‐commercial soybean ( Glycine max L. Merr.) varieties targeting a specific set environments. Adequate modeling environmental variability genotype–environment interactions (G × E) within VTP would help farmers seed companies decide which variety choose or recommend. We propose an approach characterize environments using data from University Missouri VTP. modeled trend (EnvT) based on phenotypic mean observed phenotype in each environment. The were classified into four different EnvT environment types, soil climate used as predictors through eXtreme Gradient Boosting (XGBoost) model. Temperature late vegetative flowering, soil‐saturated hydraulic conductivity, silt content key drivers EnvT. identified overrepresented (62%) increased ratio between G E variance. A simulation case study verified that random removal sites dataset quickly degraded analysis, implying increasing number underrepresented is recommended. Our results demonstrate characterization essential for optimizing resource allocation VTP, thereby supporting end goal aiding utilize best their production
Language: Английский
Citations
0Plants, Journal Year: 2025, Volume and Issue: 14(3), P. 484 - 484
Published: Feb. 6, 2025
Plants produce a diverse array of compounds that play crucial roles in growth, development, and responses to abiotic biotic stresses. Understanding the fluxes within metabolic pathways is essential for guiding strategies aimed at directing metabolism crop improvement plant natural product industry. Over past decade, network modeling has emerged as predominant tool integration, quantification, prediction spatial temporal distribution flows. In this review, we present primary methods constructing mathematical models systems highlight recent achievements using modeling. Furthermore, discuss current challenges applying flux analysis plants explore potential use machine learning technologies The practical application expected provide significant insights into structure regulation networks.
Language: Английский
Citations
0Agricultural Systems, Journal Year: 2024, Volume and Issue: 224, P. 104237 - 104237
Published: Dec. 12, 2024
Language: Английский
Citations
1European Journal of Agronomy, Journal Year: 2024, Volume and Issue: 164, P. 127461 - 127461
Published: Dec. 9, 2024
Language: Английский
Citations
0