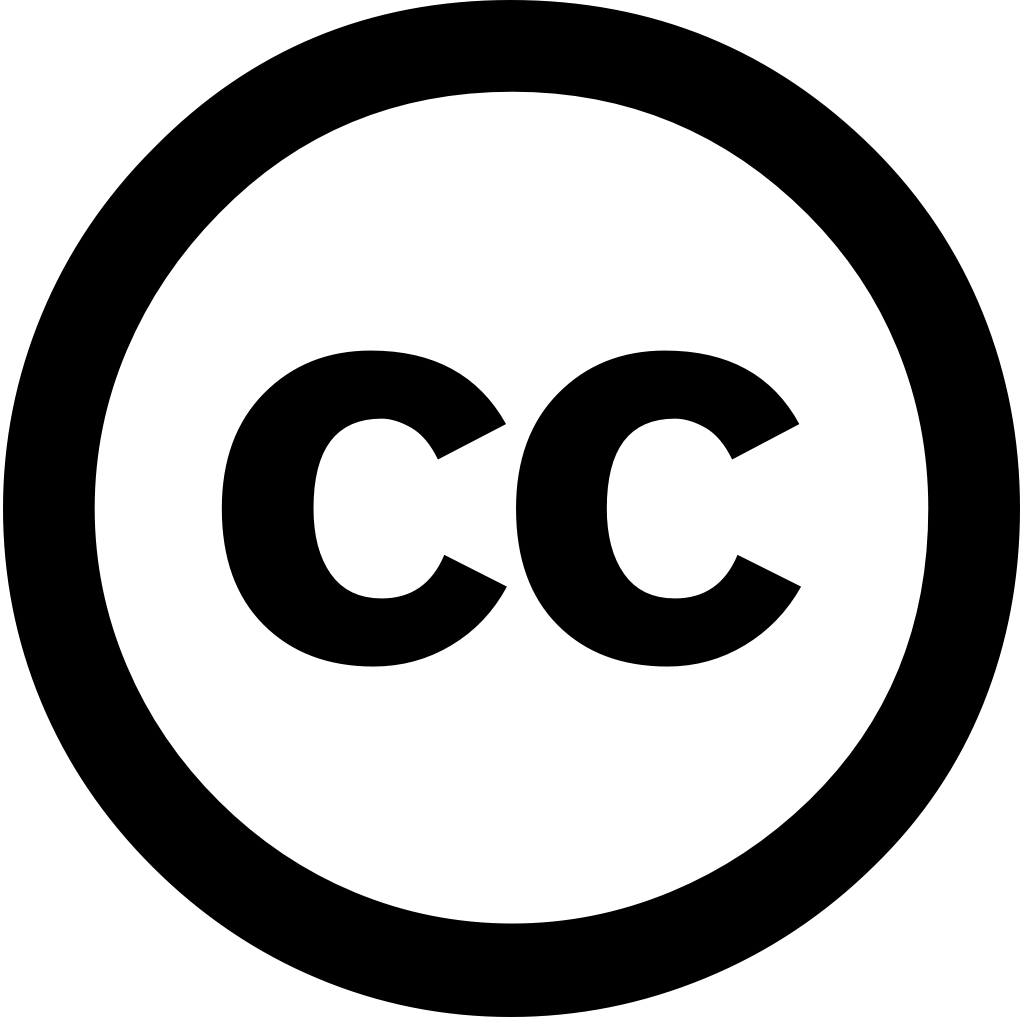
Research Square (Research Square), Journal Year: 2023, Volume and Issue: unknown
Published: Dec. 12, 2023
Abstract This study introduces a comprehensive framework aimed at automating the process of detecting yam tuber quality attributes. is achieved through integration Internet Things (IoT) devices and robotic systems. The primary focus development specialized computer codes that extract relevant image features categorize tubers into one three classes: "Good," "Diseased," or "Insect Infected." By employing variety machine learning algorithms, including tree support vector machines (SVMs), k-nearest neighbors (KNN), an impressive accuracy over 90% in effective classification. Furthermore, algorithm was designed utilizing artificial neural network (ANN), which exhibited 92.3% based on its confusion matrix analysis. effectiveness developed were substantiated deployment testing. Although few instances misclassification observed, overall outcomes indicate significant potential for transforming assessment contributing to realm precision agriculture. alignment with prior research endeavors within field, highlighting pivotal role automated precise assessment. IoT systems agricultural practices presents exciting possibilities data-driven decision-making heightened productivity. minimizing human intervention providing real-time insights, approach has optimize processes. Therefore, this successfully demonstrates practical application technologies purpose detection, laying groundwork progress sector.
Language: Английский