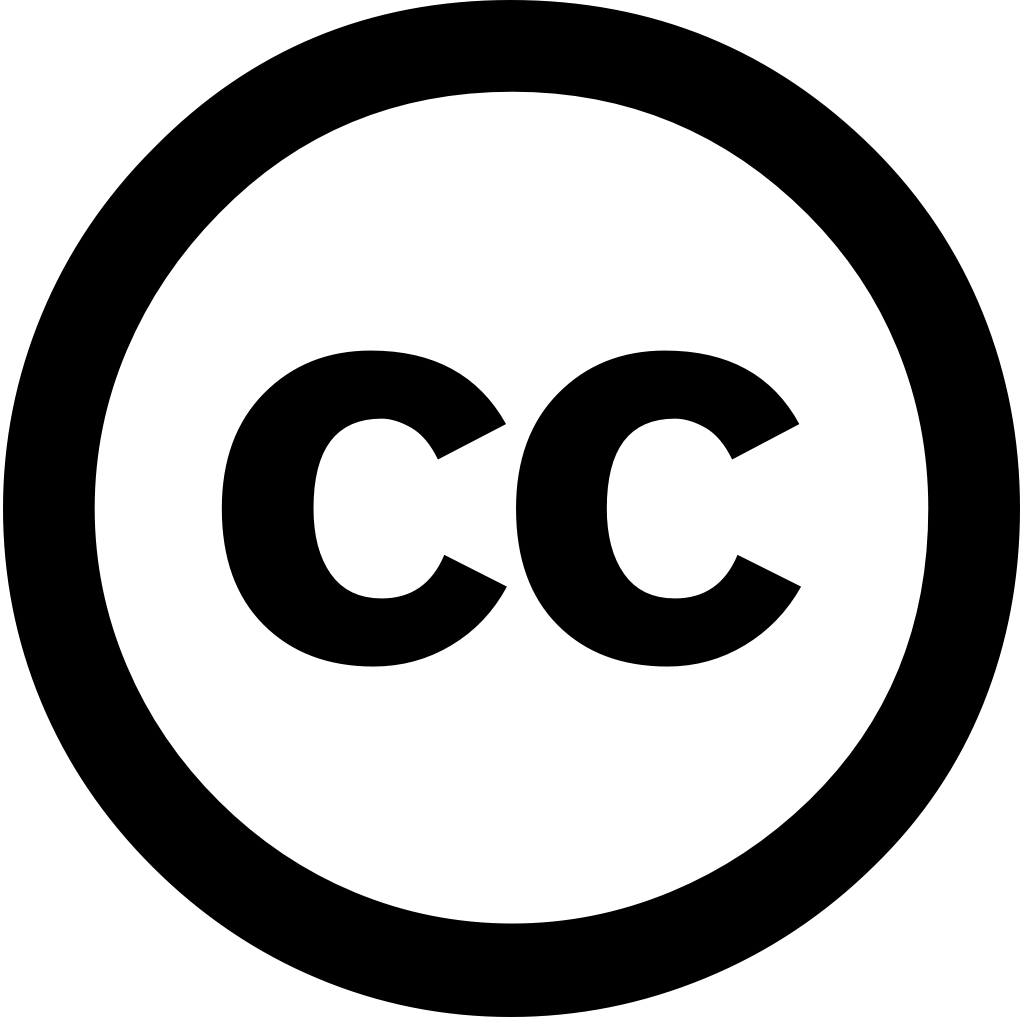
Sensors, Journal Year: 2025, Volume and Issue: 25(7), P. 2146 - 2146
Published: March 28, 2025
In the oil and gas IIoT environment, fire detection systems heavily depend on sensor data, which can be prone to inaccuracies due faulty or unreliable sensors. These issues, such as noise, missing values, outliers, drift, readings, lead delayed missed predictions, posing significant safety operational risks in industrial IoT environment. This paper presents an approach for handling sensors edge servers within environment enhance reliability accuracy of prediction through multi-sensor fusion preprocessing, machine learning (ML)-driven probabilistic model adjustment, uncertainty handling. First, a real-time anomaly statistical assessment mechanism is employed preprocess filtering out readings normalizing data from multiple types using dynamic thresholding, adapts behavior real-time. The proposed also deploys algorithms dynamically adjust models based reliability, thereby improving even presence data. A belief mass assignment introduced, giving more weight reliable ensure they have stronger influence detection. Simultaneously, update strategy continuously adjusts trust levels, reducing impact over time. Additionally, measurements Hellinger Deng entropy, along with Dempster-Shafer Theory, enable integration conflicting inputs decision-making improves by managing discrepancies provides solution mitigating environments.
Language: Английский