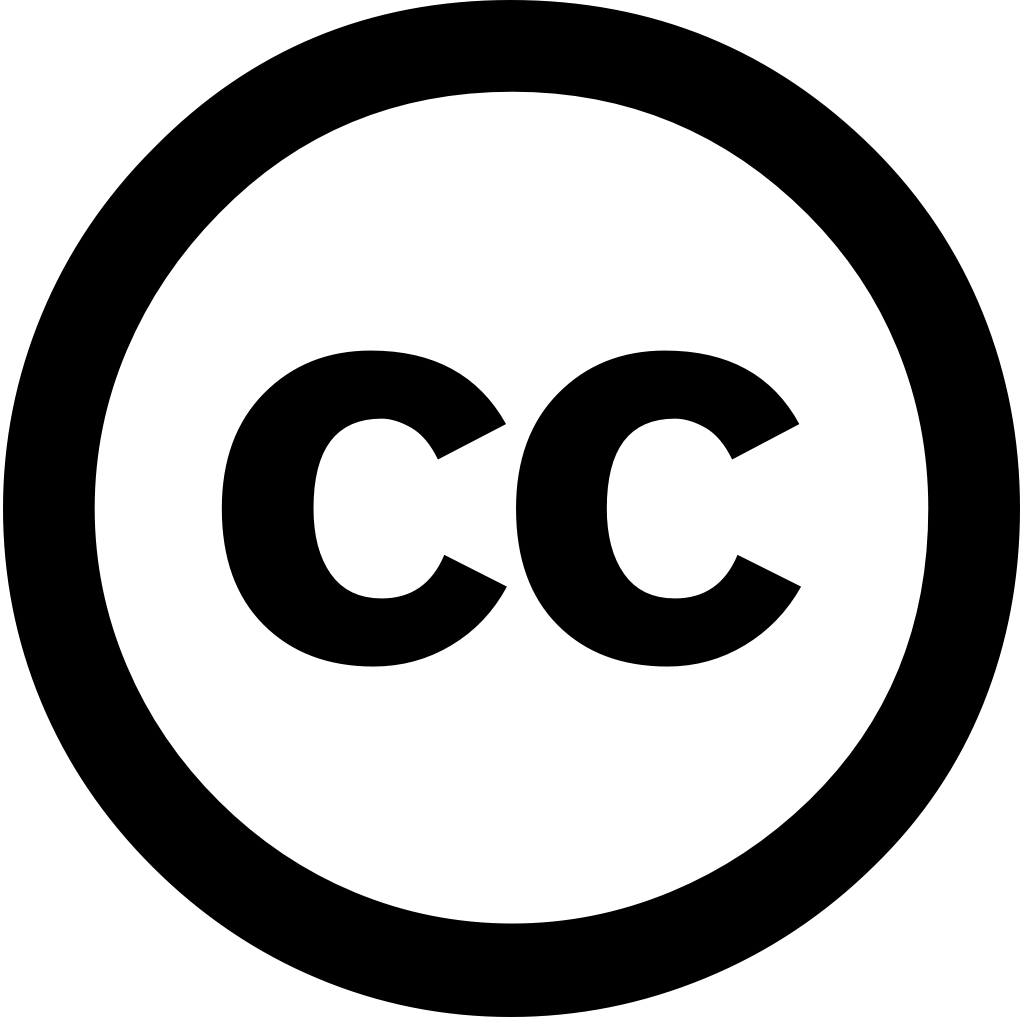
Remote Sensing, Journal Year: 2025, Volume and Issue: 17(9), P. 1597 - 1597
Published: April 30, 2025
Africa’s rapidly growing population is driving unprecedented demands on agricultural production systems. However, yields in Africa are far below their potential. One of the challenges leading to low productivity Africa‘s poor soil quality. Effective fertility management an essential key factor for optimizing while ensuring environmental sustainability. Key properties—such as organic carbon (SOC), nutrient levels (i.e., nitrogen (N), phosphorus (P), potassium (K), moisture retention (MR) or content (MC), and texture (clay, sand, loam fractions)—are critical factors influencing crop yield. In this context, study conducts extensive literature review use hyperspectral remote sensing technologies, with a particular focus freely accessible data (e.g., PRISMA, EnMAP), well evaluation advanced Artificial Intelligence (AI) models analyzing processing spectral map attributes. More specifically, examined progress applying technologies monitoring mapping properties over last 15 years (2008–2024). Our results demonstrated that (i) only very few studies have explored high-resolution sensors satellite sensors) property Africa; (ii) there considerable value AI approaches estimating attributes, strong recommendation further explore potential deep learning techniques; (iii) despite advancements AI-based methodologies availability sensors, combined application remains underexplored African context. To our knowledge, no yet integrated these Africa. This also highlights adopting encompassing both imaging spectroscopy) enhance accurate Africa, thereby constituting base addressing question yield gap.
Language: Английский