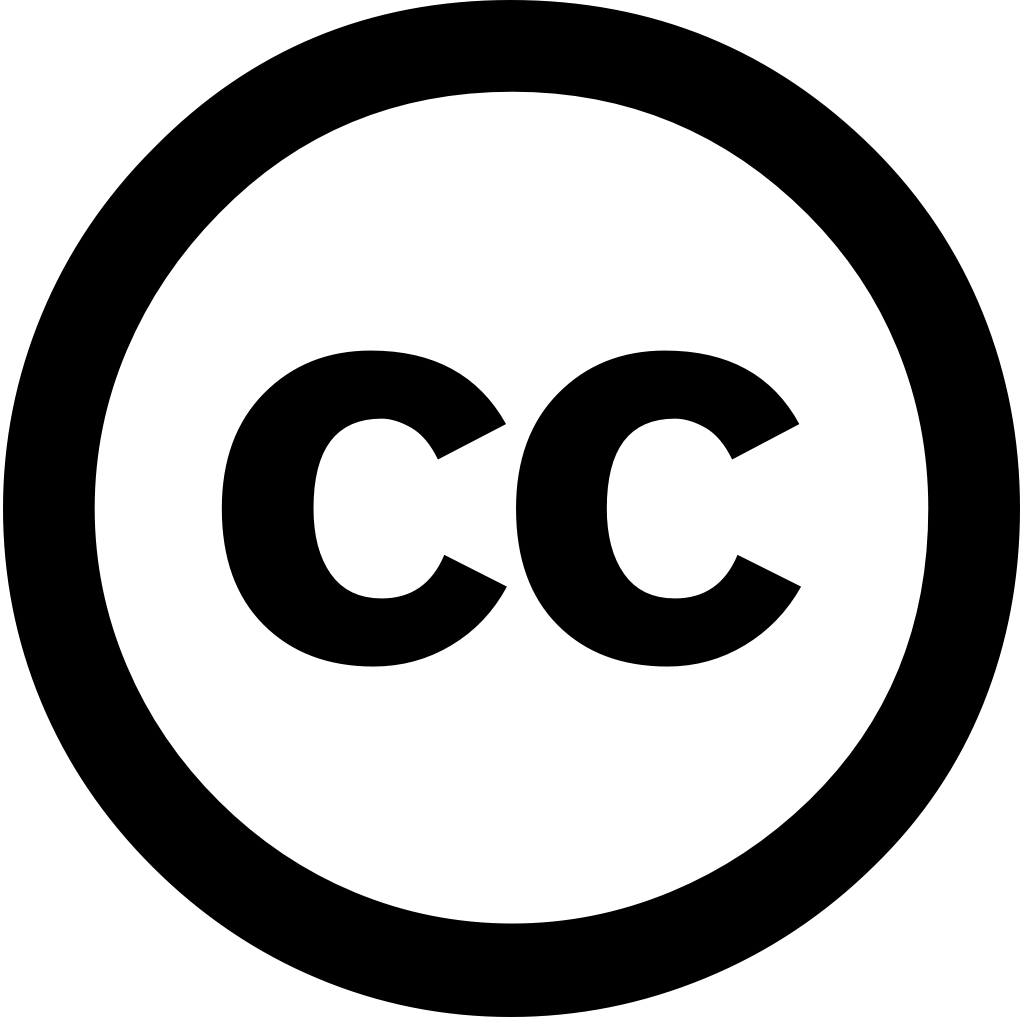
Geomorphology, Journal Year: 2024, Volume and Issue: 455, P. 109185 - 109185
Published: April 4, 2024
Field surveys to collect data from fluvial ecosystems traditionally focus on specific phenomena related geomorphology or hydrology. Low-cost unmanned aerial vehicles (UAVs) additionally empower the fast and massive collection of airborne photogrammetry, providing geospatially explicit information. This remote sensing complements field by offering contextual information geomorphological conditions, including digital terrain models. AI-based image recognition can augment extrapolate archetypal object classes through name labels, such as "gravel", "sand", "plant", "large wood". However, obtaining sufficient ground truth for these classifications, particularly in morphodynamic environments, is challenging induces high costs. study introduces a transfer learning approach address challenge low availability, enabling mapping complex objects landscapes. We leverage learned general structure deep convolutional neural network (CNN) pre-trained broad range images. The fixed latent features CNN stem GoogLeNet. A feature extractor serves classify with limited amounts. Satisfactory performance measured recall rate, expressing ability model find all occurrences class an image. High spatial heterogeneity locations measurements x-y plane improves performance. With minimum 400 labeled instances, achieves satisfactory 93.75-% wood" target class, evidence effectiveness studies. detect large woods river environments critical restoration efforts it helps create fish habitat, which essential supporting biodiversity.
Language: Английский