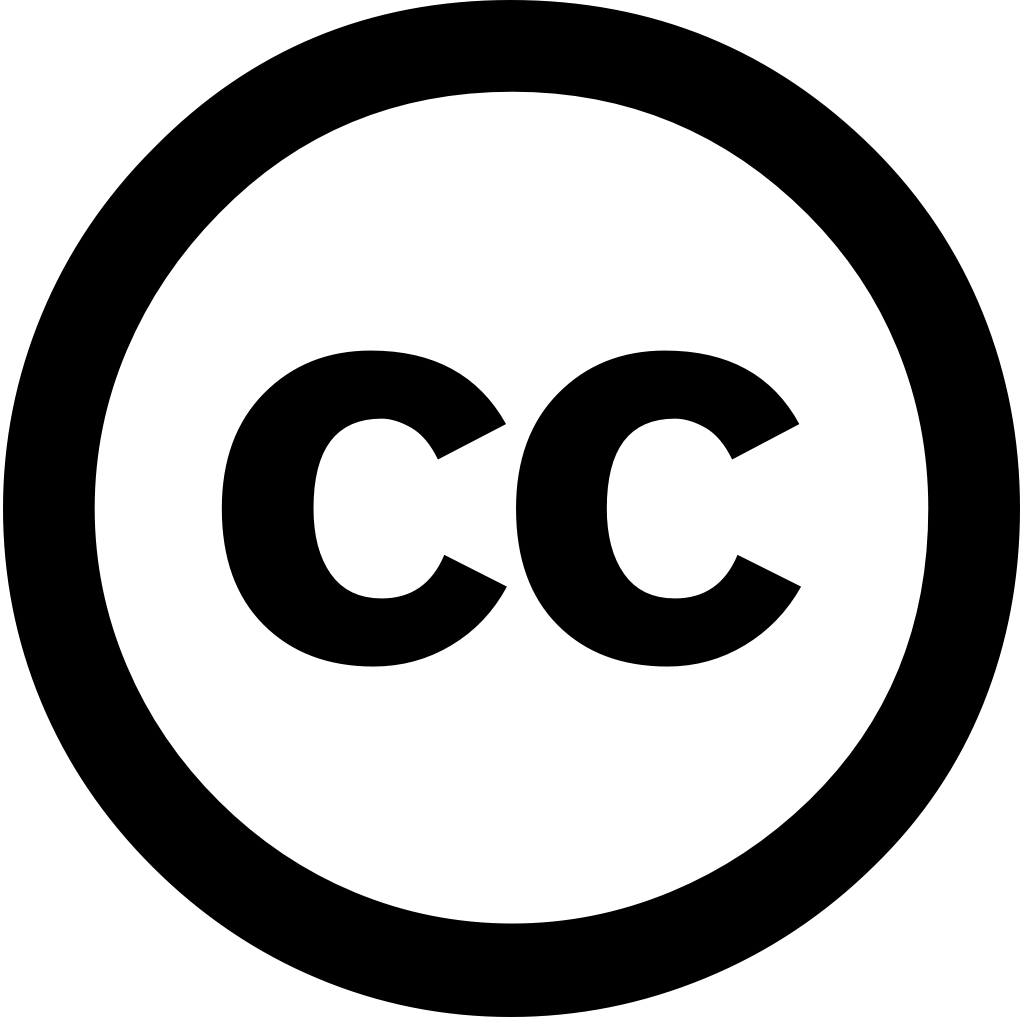
European Journal of Radiology, Journal Year: 2025, Volume and Issue: 186, P. 112049 - 112049
Published: March 14, 2025
Language: Английский
European Journal of Radiology, Journal Year: 2025, Volume and Issue: 186, P. 112049 - 112049
Published: March 14, 2025
Language: Английский
Annals of Surgical Oncology, Journal Year: 2024, Volume and Issue: 31(12), P. 7738 - 7749
Published: July 16, 2024
Language: Английский
Citations
5Ageing Research Reviews, Journal Year: 2024, Volume and Issue: 102, P. 102576 - 102576
Published: Nov. 7, 2024
Language: Английский
Citations
5Pharmaceutics, Journal Year: 2024, Volume and Issue: 16(2), P. 260 - 260
Published: Feb. 9, 2024
The use of data-driven high-throughput analytical techniques, which has given rise to computational oncology, is undisputed. widespread machine learning (ML) and mathematical modeling (MM)-based techniques widely acknowledged. These two approaches have fueled the advancement in cancer research eventually led uptake telemedicine care. For diagnostic, prognostic, treatment purposes concerning different types research, vast databases varied information with manifold dimensions are required, indeed, all this can only be managed by an automated system developed utilizing ML MM. In addition, MM being used probe relationship between pharmacokinetics pharmacodynamics (PK/PD interactions) anti-cancer substances improve treatment, also refine quality existing models incorporated at steps development related routine patient This review will serve as a consolidation benefits special focus on area prognosis anticancer therapy, leading identification challenges (data quantity, ethical consideration, data privacy) yet fully addressed current studies.
Language: Английский
Citations
4Journal of Cloud Computing Advances Systems and Applications, Journal Year: 2024, Volume and Issue: 13(1)
Published: April 19, 2024
Abstract The recent advancements in automated lung cancer diagnosis through the application of Convolutional Neural Networks (CNN) on Computed Tomography (CT) scans have marked a significant leap medical imaging and diagnostics. precision these CNN-based classifiers detecting analyzing symptoms has opened new avenues early detection treatment planning. However, despite technological strides, there are critical areas that require further exploration development. In this landscape, computer-aided diagnostic systems artificial intelligence, particularly deep learning methods like region proposal network, dual path local binary patterns, become pivotal. face challenges such as limited interpretability, data variability handling issues, insufficient generalization. Addressing is key to enhancing accurate diagnosis, fundamental for effective planning improving patient outcomes. This study introduces an advanced approach combines Network with DenseNet, leveraging fusion mobile edge computing identification classification. integration techniques enables system amalgamate information from multiple sources, robustness accuracy model. Mobile facilitates faster processing analysis CT scan images by bringing computational resources closer source, crucial real-time applications. undergo preprocessing, including resizing rescaling, optimize feature extraction. DenseNet-CNN model, strengthened capabilities, excels extracting features scans, effectively distinguishing between healthy cancerous tissues. classification categories include Normal, Benign, Malignant, latter sub-categorized into adenocarcinoma, squamous cell carcinoma, large carcinoma. controlled experiments, outperformed existing state-of-the-art methods, achieving impressive 99%. indicates its potential powerful tool cancer, advancement technology.
Language: Английский
Citations
4Therapeutic Advances in Respiratory Disease, Journal Year: 2024, Volume and Issue: 18
Published: Jan. 1, 2024
Background: Invasive lung adenocarcinoma with MPP/SOL components has a poor prognosis and often shows tendency to recurrence metastasis. This may require adjustment of treatment strategies. Preoperative identification is essential for decision-making subsequent treatment. Objective: study aimed preoperatively predict the probability in adenocarcinomas by comprehensive model that includes radiomics features, clinical characteristics, serum tumor biomarkers. Design: A retrospective case control, diagnostic accuracy study. Methods: retrospectively recruited 273 patients (males: females, 130: 143; mean age ± standard deviation, 63.29 10.03 years; range 21–83 years) who underwent resection invasive adenocarcinoma. Sixty-one (22.3%) were diagnosed components. Radiomic features extracted from CT before surgery. Clinical, radiomic, combined models developed using logistic regression algorithm. The radiomic signatures integrated into nomogram. performance was evaluated area under curve (AUC). Studies scored according Radiomics Quality Score Transparent Reporting Multivariable Prediction Model Individual Prognosis or Diagnosis guidelines. Results: achieved best AUC values 0.858 0.822 training test cohort, respectively. Tumor size (T_size), solid (ST_size), consolidation-to-tumor ratio (CTR), years smoking, CYFRA 21-1, squamous cell carcinoma antigen used construct model. 0.741 0.705 nomogram showed higher AUCs 0.894 0.843 Conclusion: validated nomogram, visual tool integrates indicators innovative facilitates differentiation micropapillary within achieves AUC, indicating superior predictive accuracy.
Language: Английский
Citations
4Frontiers in Artificial Intelligence, Journal Year: 2025, Volume and Issue: 7
Published: Jan. 8, 2025
Tumor heterogeneity significantly complicates the selection of effective cancer treatments, as patient responses to drugs can vary widely. Personalized therapy has emerged a promising strategy enhance treatment effectiveness and precision. This study aimed develop personalized drug recommendation model leveraging genomic profiles optimize therapeutic outcomes. A content-based filtering algorithm was implemented predict sensitivity. Patient features were characterized by tumor microenvironment (TME), represented fingerprints. The trained validated using Genomics Drug Sensitivity in Cancer (GDSC) database, followed independent validation with Cell Line Encyclopedia (CCLE) dataset. Clinical application assessed Genome Atlas (TCGA) dataset, Best Overall Response (BOR) serving clinical efficacy measure. Two multilayer perceptron (MLP) models built IC50 values for 542 cell lines across 18 drugs. exhibited high predictive accuracy, correlation coefficients (R) 0.914 training set 0.902 test set. Predictions cytotoxic drugs, including Docetaxel (R = 0.72) Cisplatin 0.71), particularly robust, whereas predictions targeted therapies less accurate < 0.3). Validation CCLE (MFI endpoint) showed strong correlations 0.67). Application TCGA data successfully predicted outcomes, significant association 6-month progression-free survival (PFS, P 0.007, AUC 0.793). demonstrates performance preclinical datasets, showing its potential real-world therapy. By bridging BOR endpoints, this approach provides tool optimizing patient-specific treatments.
Language: Английский
Citations
0Heliyon, Journal Year: 2025, Volume and Issue: unknown, P. e41850 - e41850
Published: Jan. 1, 2025
:BackgroundAs one of the cancers with highest incidence and mortality rates worldwide, timeliness accuracy cell type diagnosis in lung cancer are crucial for patients' treatment decisions. This study aims to develop a novel deep learning model provide efficient, accurate, cost-effective auxiliary pathological types cells.MethodThis paper introduces named PortNet, designed significantly reduce model's parameter size achieve lightweight characteristics without compromising classification accuracy. We incorporated 1x1 convolutional blocks into Depthwise Separable Convolution architecture further decrease count. Additionally, integration Squeeze-and-Excitation self-attention module enhances feature representation substantially increasing number parameters, thereby maintaining high predictive performance.ResultOur tests demonstrated that PortNet reduces total count 2,621,827, which is over fifth smaller compared some mainstream CNN models, marking substantial advancement deployment portable devices. also established widely-used traditional models as benchmarks illustrate efficacy PortNet. In external tests, achieved an average (ACC) 99.89% Area Under Curve (AUC) 99.27%. During five-fold cross-validation, maintained ACC 99.51%±1.50% F1 score 99.50%±1.51%, showcasing its capability exceptionally presents promising opportunity hospital systems assist physicians diagnosis.ConclusionThis through innovative structure while stability, demonstrating outstanding performance tasks. The holds potential become diagnostic tool future.
Language: Английский
Citations
0Medical Oncology, Journal Year: 2025, Volume and Issue: 42(2)
Published: Jan. 21, 2025
Abstract Lung and colon cancer are among the most commonly diagnosed fatal types in world. Due to their metastatic properties, they complicate treatment process pose a great threat human health. These aggressive of resistant chemotherapy drugs. Therefore, it is extremely important investigate therapeutic effects natural compounds. In our previous study, effective doses Royal Jelly (RJ) (100 mg/mL) Aloe vera (AVE) (20 µg/mL) were determined tested separately combination on lung colorectal cells. Glycolytic capacities using Seahorse XFe24 Analyzer, total transcriptome profiles sequenced NovaSeq 6000, BAX BCL-2 gene levels RT-qPCR. It was seen that RJ + AVE affected glycolytic capacity more genes HT29, alone reduce expression level related cell proliferation cycle. After treatments, apoptotic which triggered via MAPK pathway found cancer. Moreover, increased decreased both observed glycolysis process, cycle, apoptosis particular, be
Language: Английский
Citations
0BMC Cancer, Journal Year: 2025, Volume and Issue: 25(1)
Published: Feb. 7, 2025
Gastric cancer patients are prone to lower extremity deep vein thrombosis (DVT) after surgery, which is an important cause of death in postoperative patients. Therefore, it particularly find a suitable way predict the risk occurrence DVT GC This study aims explore effectiveness using machine learning (ML) assisted radiomics build imaging models for prediction surgery. Included this retrospective were eligible who underwent surgery GC. CT data from these collected and divided into training set validation set. The least absolute shrinkage selection operator (LASSO) algorithm was applied reduce dimensionality variables Four algorithms, known as random forest (RF), extreme gradient boosting (XGBoost), support vector (SVM) naive Bayes (NB), used develop predicting These subsequently validated internal external cohort. LASSO analysis identified 10 variables, based on four ML established, then incorporated with clinical characteristics Among models, RF NB demonstrated highest predictive performance, achieving AUC 0.928, while SVM XGBoost achieved slightly 0.915 0.869, respectively. algorithms information may prove be novel non-invasive
Language: Английский
Citations
0Published: Jan. 1, 2025
Language: Английский
Citations
0