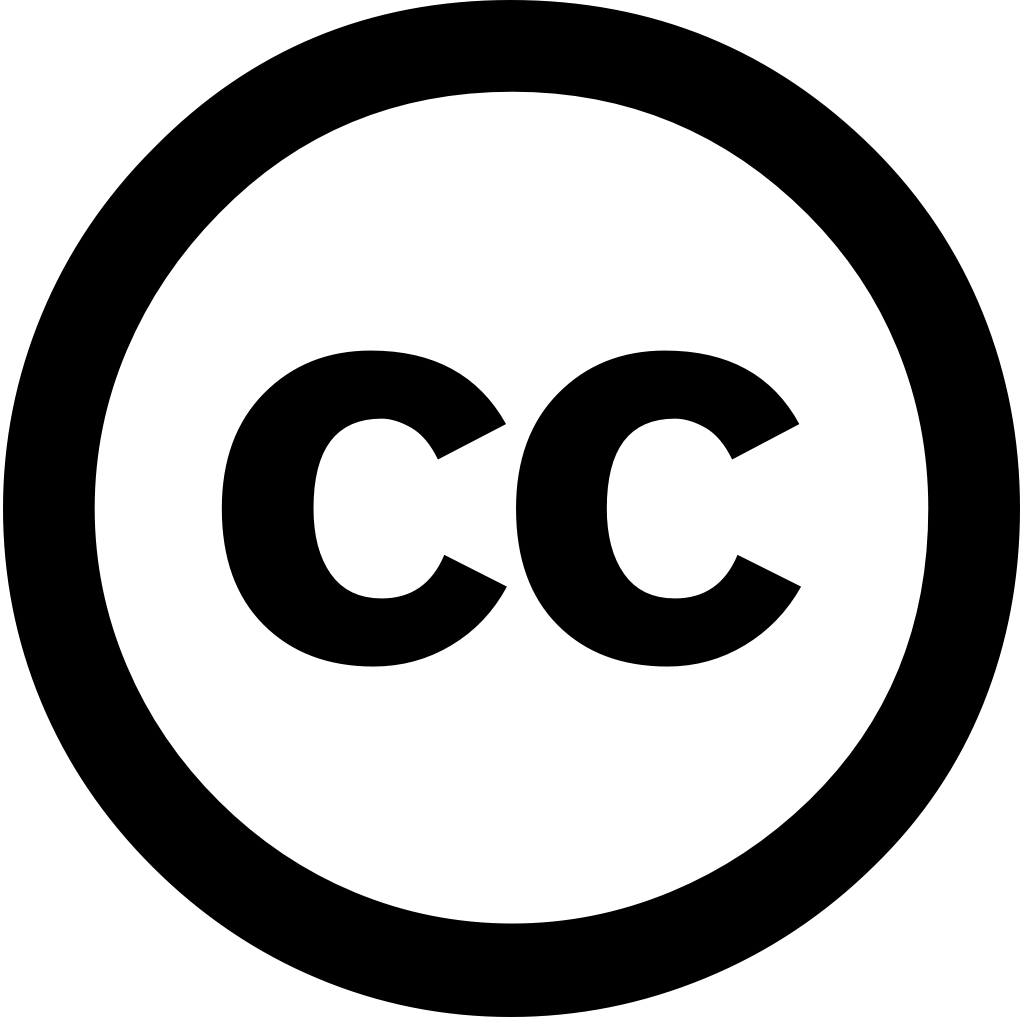
Environmental and Sustainability Indicators, Journal Year: 2024, Volume and Issue: 25, P. 100559 - 100559
Published: Dec. 16, 2024
Language: Английский
Environmental and Sustainability Indicators, Journal Year: 2024, Volume and Issue: 25, P. 100559 - 100559
Published: Dec. 16, 2024
Language: Английский
Frontiers in Environmental Science, Journal Year: 2024, Volume and Issue: 12
Published: Aug. 16, 2024
Rainfall plays an important role in maintaining the water cycle by replenishing aquifers, lakes, and rivers, supporting aquatic life, sustaining terrestrial ecosystems. Accurate prediction is crucial given intricate interplay of atmospheric oceanic phenomena, especially amidst contemporary challenges. In this study, to predict rainfall, 12,852 data points from open-source global weather for three cities Indonesia were utilized, incorporating input variables such as maximum temperature (°C), minimum wind speed (m/s), relative humidity (%), solar radiation (MJ/m 2 ). Three novel robust Deep Learning models used: Recurrent Neural Network (DRNN), Gated Unit (DGRU), Long Short-Term Memory (DLSTM). Evaluation results, including statistical metrics like Root-Mean-Square Errors Correction Coefficient (R ), revealed that model outperformed DRNN with values 0.1289 0.9995, respectively. DLSTM networks offer several advantages rainfall prediction, particularly sequential time series excelling handling long-term dependencies capturing patterns over extended periods. Equipped memory cell architecture forget gates, effectively retain retrieve relevant information. Furthermore, enable parallelization, enhancing computational efficiency, flexibility design regularization techniques improved generalization performance. Additionally, results indicate parameters exhibit indirect influence on while temperature, speed, have a direct relationship rainfall.
Language: Английский
Citations
5Ingeniería e Investigación, Journal Year: 2025, Volume and Issue: 44(3), P. e108609 - e108609
Published: Jan. 31, 2025
Precipitation estimation at the river basin level is essential for watershed management, analysis of extreme events and weather climate dynamics, hydrologic modeling. In recent years, new approaches tools such as artificial intelligence techniques have been used precipitation estimation, offering advantages over traditional methods. Two major paradigms are neural networks fuzzy logic systems, which can be in a wide variety configurations, including hybrid modular models. This work presents literature review on metaheuristic models based signal processes, focusing applications these estimation. The selection comparison criteria were model type, input output variables, performance metrics, fields application. An increase number this type studies was identified, mainly involving network models, tend to get more sophisticated according availability quality training data. On other hand, hybridize with There still challenges related prediction spatial temporal resolution micro-basin levels, but, overall, very promising analysis.
Language: Английский
Citations
0Discover Water, Journal Year: 2024, Volume and Issue: 4(1)
Published: Nov. 26, 2024
Artificial Intelligence (AI), including models like ChatGPT, is transforming water resources management by improving hydrological modeling, quality assessment, and flood prediction. AI techniques such as Neural Networks (ANNs) Support Vector Machines (SVMs) have enhanced streamflow predictions groundwater management, particularly in data-scarce regions. AI-powered systems Smart Microclimate Control Systems (SMCS) optimize agricultural practices, leading to better resource conservation higher crop yields. However, the scalability applicability of across diverse environments pose challenges, especially where data limited. The success depends on quality, requiring ongoing interdisciplinary research refine these technologies for real-world use. Additionally, tools while valuable knowledge dissemination analysis, raise concerns about accuracy critical decision-making contexts. In conclusion, offers significant potential addressing challenges related model scalability, collaboration essential achieving sustainable effective outcomes.
Language: Английский
Citations
1Environmental and Sustainability Indicators, Journal Year: 2024, Volume and Issue: 25, P. 100559 - 100559
Published: Dec. 16, 2024
Language: Английский
Citations
1