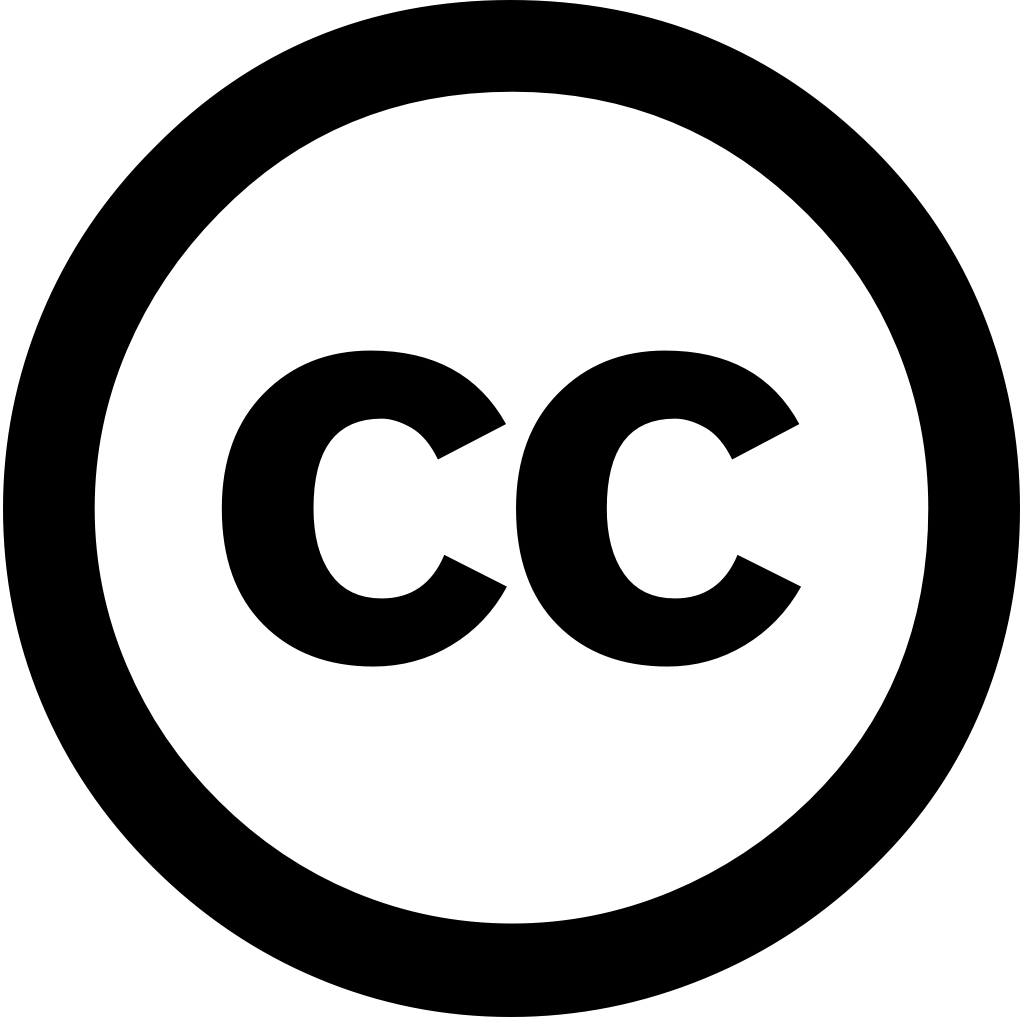
Journal of Hazardous Materials Advances, Journal Year: 2024, Volume and Issue: unknown, P. 100576 - 100576
Published: Dec. 1, 2024
Language: Английский
Journal of Hazardous Materials Advances, Journal Year: 2024, Volume and Issue: unknown, P. 100576 - 100576
Published: Dec. 1, 2024
Language: Английский
Journal of Hazardous Materials Advances, Journal Year: 2025, Volume and Issue: 17, P. 100604 - 100604
Published: Jan. 15, 2025
Language: Английский
Citations
1Physchem, Journal Year: 2025, Volume and Issue: 5(1), P. 5 - 5
Published: Jan. 23, 2025
Adsorption is a complex process since it affected by multiple variables related to the physicochemical properties of adsorbate, adsorbent and interface; therefore, understand adsorption in batch systems, kinetics, isotherms empiric models are commonly used. On other hand, artificial neural networks (ANNs) have proven be useful solving wide variety problems science engineering due their combination computational efficiency precision results; for this reason, recent years, ANNs begun used describing processes. In work, we present an ANN model fluoride ions water with layered double hydroxides (LDHs) its comparison empirical kinetic models. LHD was synthesized characterized using X-Ray diffraction, FT-Infrared spectroscopy, BET analyses zero point charge. Fluoride ion evaluated under different experimental conditions, including contact time, initial pH concentration. A total 262 experiments were conducted, resulting data training testing model. The results indicate that can accurately forecast conditions determination coefficient R2 0.9918.
Language: Английский
Citations
0Environmental Technology & Innovation, Journal Year: 2025, Volume and Issue: unknown, P. 104154 - 104154
Published: March 1, 2025
Language: Английский
Citations
0Journal of Environmental Management, Journal Year: 2025, Volume and Issue: 380, P. 125035 - 125035
Published: March 24, 2025
Language: Английский
Citations
0Chemosphere, Journal Year: 2024, Volume and Issue: 363, P. 142697 - 142697
Published: June 24, 2024
Language: Английский
Citations
3Smart Agricultural Technology, Journal Year: 2024, Volume and Issue: unknown, P. 100728 - 100728
Published: Dec. 1, 2024
Language: Английский
Citations
0Journal of Hazardous Materials Advances, Journal Year: 2024, Volume and Issue: unknown, P. 100576 - 100576
Published: Dec. 1, 2024
Language: Английский
Citations
0