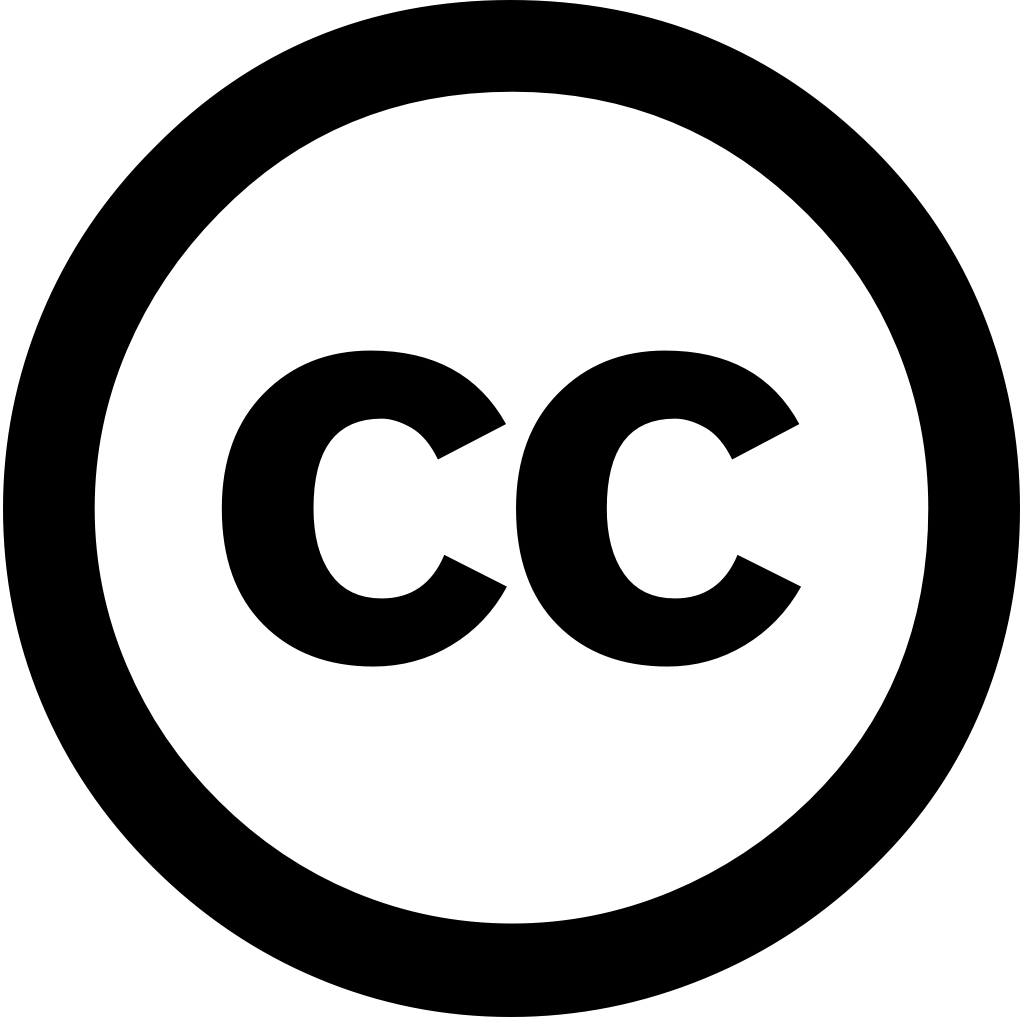
Decision Analytics Journal, Journal Year: 2024, Volume and Issue: unknown, P. 100539 - 100539
Published: Dec. 1, 2024
Language: Английский
Decision Analytics Journal, Journal Year: 2024, Volume and Issue: unknown, P. 100539 - 100539
Published: Dec. 1, 2024
Language: Английский
International Journal of Medical Informatics, Journal Year: 2024, Volume and Issue: 193, P. 105689 - 105689
Published: Nov. 4, 2024
Language: Английский
Citations
9Computers & Electrical Engineering, Journal Year: 2025, Volume and Issue: 123, P. 110106 - 110106
Published: Jan. 28, 2025
Language: Английский
Citations
1Scientific Reports, Journal Year: 2024, Volume and Issue: 14(1)
Published: Oct. 1, 2024
Language: Английский
Citations
5International Journal of Imaging Systems and Technology, Journal Year: 2025, Volume and Issue: 35(2)
Published: Feb. 18, 2025
ABSTRACT Cervical cancer persists as a significant global health concern, underscoring the vital importance of early detection for effective treatment and enhanced patient outcomes. While traditional Pap smear tests remain an invaluable diagnostic tool, they are inherently time‐consuming susceptible to human error. This study introduces innovative approach that employs convolutional neural networks (CNN) enhance accuracy efficiency cervical cell classification. The proposed model incorporates Convolutional Block Attention Module (CBAM) parallel branch architectures, which facilitate feature extraction by focusing on crucial spatial channel information. process entails identification utilization most pertinent elements within image purpose was meticulously assessed SIPaKMeD dataset, attaining exceptional degree (92.82%), surpassed performance CNN models. incorporation sophisticated attention mechanisms enables not only accurately classify images but also interpretability emphasizing regions images. highlights transformative potential cutting‐edge deep learning techniques in medical analysis, particularly screening, providing powerful tool support pathologists accurate diagnosis. Future work will explore additional extend application this architecture other imaging tasks, further enhancing its clinical utility impact
Language: Английский
Citations
0Electronics, Journal Year: 2025, Volume and Issue: 14(6), P. 1140 - 1140
Published: March 14, 2025
Cervical cancer remains a significant global public health challenge, particularly in low- and middle-income countries where invasive diagnostic methods are underutilized due to limited medical resources. Machine learning has provided new pathway address this but existing machine prediction face three major challenges: feature redundancy, class imbalance, sample scarcity. To these issues, study proposes hybrid data processing strategy with Conditional Tabular Generative Adversarial Networks (CTGAN) construct more accurate efficient auxiliary model for cervical cancer. The first employs the Minimal Redundancy Maximal Relevance (mRMR) algorithm XGBoost-based Recursive Feature Elimination (RFE) secondary screening. Subsequently, SMOTE-ENN combination sampling method is applied handle extreme CTGAN utilized augment dataset, thereby mitigating Experimental validation on Risk Factors of Cancer (RFCC) dataset from Venezuelan hospital demonstrates that, after proposed strategy, Logistic Regression (LR) achieves best overall results, accuracy, precision, recall, F1-score reaching 99.00%, 99.28%, 98.77%, 99.02%, respectively, outperforming methods.
Language: Английский
Citations
0Digital Health, Journal Year: 2025, Volume and Issue: 11
Published: March 1, 2025
Objectives This study develops a machine learning (ML)-based cervical cancer prediction system emphasizing explainability. A hybrid feature selection method is proposed to enhance predictive accuracy and stability, alongside evaluation of multiple classification algorithms. The integration explainable artificial intelligence (XAI) techniques ensures transparency interpretability in model decisions. Methods approach combining correlation-based recursive elimination introduced. An ensemble integrating random forest, extreme gradient boosting, logistic regression compared against eight classical ML Generative methods, such as variational autoencoders generative teaching networks, were evaluated but showed suboptimal performance. research integrates global local XAI techniques, including individual contributions tree-based explanations, interpret effects data balancing on performance are examined stabilize precision, recall, F1 scores. Classical models without preprocessing achieve 95-96% exhibit instability. Results strategies significantly creating robust model. achieves 98% with an area under the curve 99.50%, outperforming other models. Domain experts validate critical contributing features, confirming practical relevance. Incorporating domain knowledge increases transparency, making predictions interpretable trustworthy for clinical use. Conclusion Hybrid combined substantially improves reliability. supporting trustworthiness, demonstrating significant potential decision-making.
Language: Английский
Citations
0Scientific Reports, Journal Year: 2025, Volume and Issue: 15(1)
Published: April 14, 2025
Language: Английский
Citations
0Published: Jan. 1, 2025
Language: Английский
Citations
0Journal of Pathology Informatics, Journal Year: 2024, Volume and Issue: 15, P. 100398 - 100398
Published: Sept. 27, 2024
Language: Английский
Citations
3Healthcare Analytics, Journal Year: 2024, Volume and Issue: 6, P. 100366 - 100366
Published: Oct. 9, 2024
Language: Английский
Citations
3