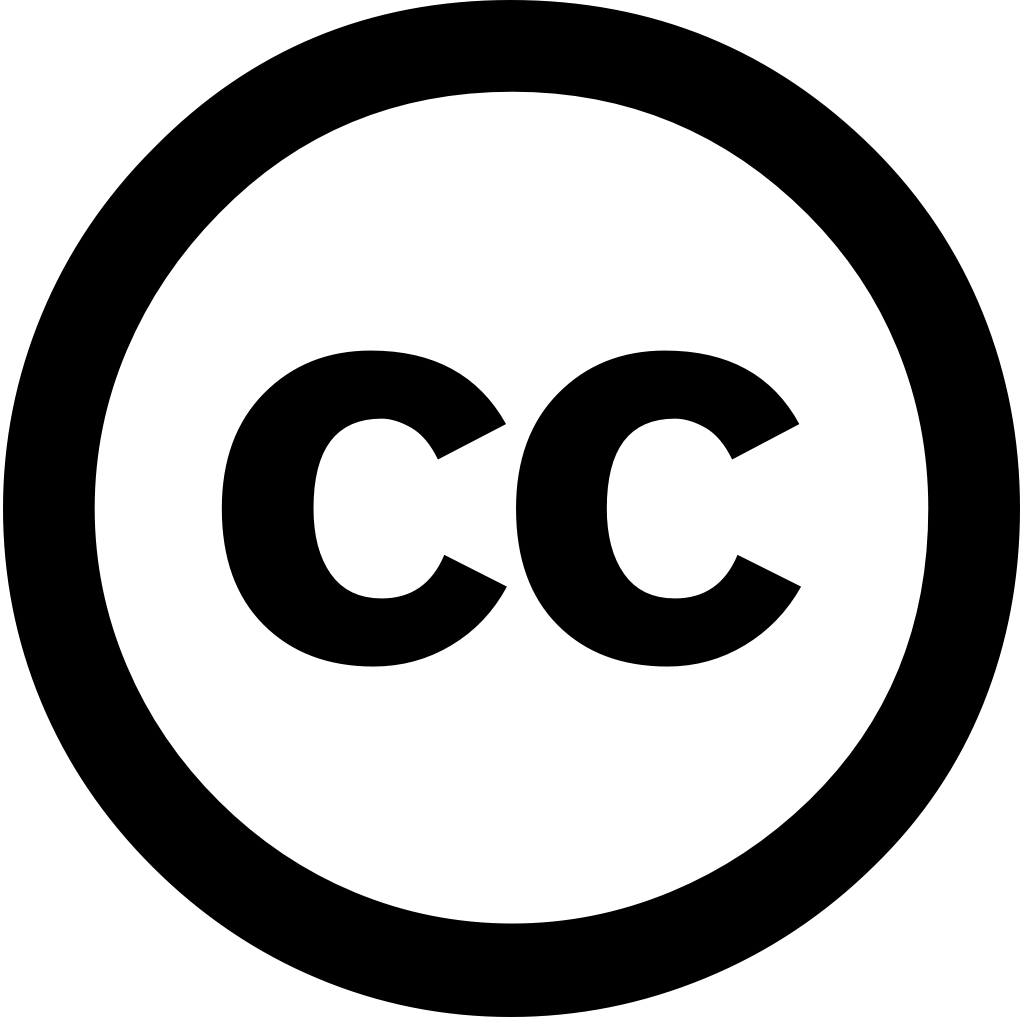
Decision Analytics Journal, Journal Year: 2024, Volume and Issue: unknown, P. 100539 - 100539
Published: Dec. 1, 2024
Language: Английский
Decision Analytics Journal, Journal Year: 2024, Volume and Issue: unknown, P. 100539 - 100539
Published: Dec. 1, 2024
Language: Английский
Healthcare Analytics, Journal Year: 2024, Volume and Issue: 6, P. 100366 - 100366
Published: Oct. 9, 2024
Language: Английский
Citations
3Healthcare Analytics, Journal Year: 2024, Volume and Issue: unknown, P. 100373 - 100373
Published: Dec. 1, 2024
Language: Английский
Citations
3EAI Endorsed Transactions on Internet of Things, Journal Year: 2024, Volume and Issue: 11
Published: Nov. 19, 2024
INTRODUCTION: Cervical cancer is a significant global health concern, particularly in underdeveloped nations where preventive healthcare measures are limited. Early identification of the risks associated with cervical essential for both prevention and treatment. OBJECTIVES: In recent years, machine-learning algorithms have gained popularity as potential techniques determining person's risk developing based on demographic medical information. This study uses dataset that contains patient demographics, clinical history, results from diagnostic tests to examine how machine learning-based can be used predict cancer. METHODS: Various learning approaches create predictive systems, including Support Vector Machine (SVM), Naïve Bayes (NB), Decision Tree (DT), K-Nearest Neighbors (KNN), Random Forest (RF), Logistic Regression (LR), Gradient Boosting (GB), Nearest Centroid (NC), Multilayer Perceptron(MP), AdaBoost (AB). RESULTS: The prediction capability these models assessed using performance metrics such accuracy, sensitivity, specificity, f-measure, precision, area under receiver operating characteristic curve (AUC-ROC). Our show decision tree has highest f1-score (98.91%, 97.81%, 0.9889). Additionally, model was optimized by use hyperparameter tuning. After adjustment, (SVM) showed superior accuracy 99.64%, precision 99.26%, an F1-score 0.9963, thereby indicating its probability prediction. We also created web application estimate CONCLUSION: findings this highlight significance SVM demonstrate capabilities enhance accurate outcomes screening.
Language: Английский
Citations
1Decision Analytics Journal, Journal Year: 2024, Volume and Issue: unknown, P. 100539 - 100539
Published: Dec. 1, 2024
Language: Английский
Citations
0