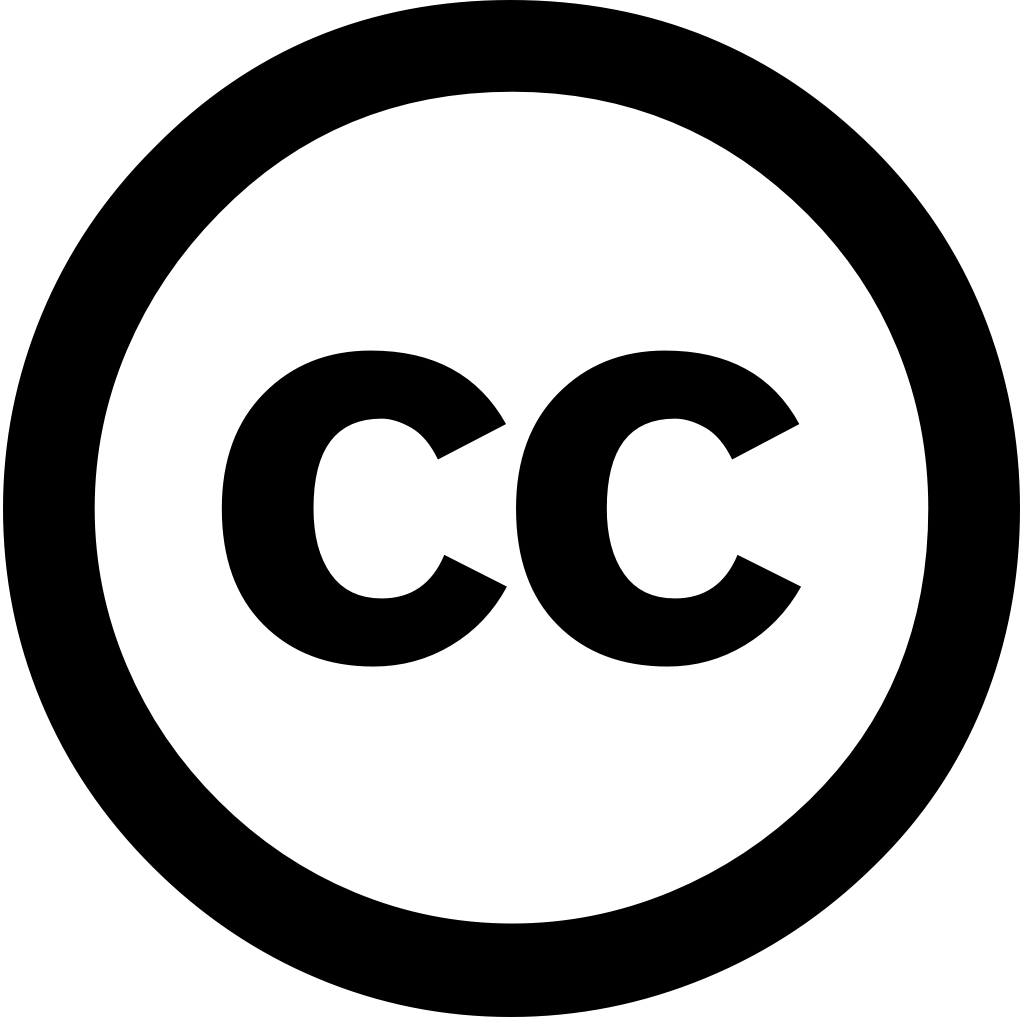
e-Prime - Advances in Electrical Engineering Electronics and Energy, Journal Year: 2024, Volume and Issue: 10, P. 100817 - 100817
Published: Oct. 20, 2024
Language: Английский
e-Prime - Advances in Electrical Engineering Electronics and Energy, Journal Year: 2024, Volume and Issue: 10, P. 100817 - 100817
Published: Oct. 20, 2024
Language: Английский
Renewable Energy, Journal Year: 2025, Volume and Issue: unknown, P. 122821 - 122821
Published: March 1, 2025
Language: Английский
Citations
1Discover Sustainability, Journal Year: 2024, Volume and Issue: 5(1)
Published: Nov. 12, 2024
An accurate renewable energy output forecast is essential for efficiency and power system stability. Long Short-Term Memory(LSTM), Bidirectional LSTM(BiLSTM), Gated Recurrent Unit(GRU), Convolutional Neural Network-LSTM(CNN-LSTM) Deep Network (DNN) topologies are tested solar wind production forecasting in this study. ARIMA was compared to the models. This study offers a unique architecture Networks (DNNs) that specifically tailored forecasting, optimizing accuracy by advanced hyperparameter tuning incorporation of meteorological temporal variables. The optimized LSTM model outperformed others, with MAE (0.08765), MSE (0.00876), RMSE (0.09363), MAPE (3.8765), R2 (0.99234) values. GRU, CNN-LSTM, BiLSTM models predicted well. Meteorological time-based factors enhanced accuracy. addition sun data improved its prediction. results show deep neural network can predict energy, highlighting importance carefully selecting characteristics fine-tuning model. work improves estimates promote more reliable environmentally sustainable electricity system.
Language: Английский
Citations
8Processes, Journal Year: 2025, Volume and Issue: 13(2), P. 477 - 477
Published: Feb. 10, 2025
As a large number of large-scale photovoltaic (PV) stations are integrated into the power grid, penetration rate PV is growing higher and higher. The intermittency volatility generation bring great pressure to safe stable operation distribution network. In order realize scientific energy dispatching optimization, predicted output data basis prerequisite. prediction method studied in this paper, based on gradient-boosting decision trees proposed. method, original first collected, sample set established through steps interpolation, supplement, integration, then pre-processed by cleaning normalization. model training during test period carried out data. Finally, results imported error analysis module. feasibility accuracy proposed analyzed comparing it with traditional method. show that normalized mean absolute (nMAE) root square (nRMSE) 7.31% 11.78%, respectively, while nMAE nRMSE 11.67% 20.39%, respectively. Thus, performance superior
Language: Английский
Citations
0Engineering Science and Technology an International Journal, Journal Year: 2024, Volume and Issue: 61, P. 101889 - 101889
Published: Nov. 30, 2024
Language: Английский
Citations
2Discover Sustainability, Journal Year: 2024, Volume and Issue: 5(1)
Published: Dec. 31, 2024
This study evaluates and differentiates five advanced machine learning models—LSTM, GRU, CNN-LSTM, Random Forest, SVR—aimed at precisely estimating solar wind power generation to enhance renewable energy forecasting. LSTM achieved a remarkable Mean Squared Error (MSE) of 0.010 R2 score 0.90, highlighting its proficiency in capturing intricate temporal relationships. GRU closely followed, demonstrating potential as viable option due combination computational efficiency accuracy (MSE = 0.015, 0.88). In datasets abundant spatial correlations, the CNN-LSTM hybrid demonstrated utility by providing novel insights into spatial–temporal patterns; nonetheless, it lagged considerably accuracy, with mean squared error 0.020 0.87. Conversely, traditional models reliable albeit less dynamic ability elucidate complexities data; for instance, Forest exhibited 0.025, while Support Vector Regression (SVR) recorded an MSE 0.030. The results affirm that deep architectures, particularly LSTM, offer transformative method forecasting, hence enhancing reliability management systems.
Language: Английский
Citations
2Applied Sciences, Journal Year: 2024, Volume and Issue: 14(23), P. 11192 - 11192
Published: Nov. 30, 2024
This paper presents a method for predicting the energy yield of photovoltaic (PV) system based on ARIMA algorithm. We analyze two key time series: specific and total PV system. Two models are developed each one selected by authors determined SPSS. Model performance is evaluated through fit statistics, providing comprehensive assessment model accuracy. The residuals’ ACF PACF examined to ensure adequacy, confidence intervals calculated residuals validate models. A monthly forecast then generated both series, complete with intervals, demonstrate models’ predictive capabilities. results highlight effectiveness in forecasting yields, offering valuable insights optimizing planning. study contributes field renewable demonstrating applicability systems.
Language: Английский
Citations
1Buildings, Journal Year: 2024, Volume and Issue: 15(1), P. 39 - 39
Published: Dec. 26, 2024
This study evaluates the performance of 15 machine learning models for predicting energy consumption (30–100 kWh/m2·year) and occupant dissatisfaction (Percentage Dissatisfied, PPD: 6–90%), key metrics optimizing building performance. Ten evaluation metrics, including Mean Absolute Error (MAE, average prediction error), Root Squared (RMSE, penalizing large errors), coefficient determination (R2, variance explained by model), are used. XGBoost achieves highest accuracy, with an MAE 1.55 kWh/m2·year a PPD 3.14%, alongside R2 values 0.99 0.97, respectively. While these highlight XGBoost’s superiority, its margin improvement over LightGBM (energy MAE: 2.35 kWh/m2·year, 3.89%) is context-dependent, suggesting application in high-precision scenarios. ANN excelled at predictions, achieving lowest (1.55%) Percentage (MAPE: 4.97%), demonstrating ability to model complex nonlinear relationships. modeling advantage contrasts LightGBM’s balance speed making it suitable computationally constrained tasks. In contrast, traditional like linear regression KNN exhibit high errors (e.g., 17.56 17.89%), underscoring their limitations respect capturing complexities datasets. The results indicate that advanced methods particularly effective owing intricate relationships manage high-dimensional data. Future research should validate findings diverse real-world datasets, those representing varying types climates. Hybrid combining interpretability precision ensemble or neural be explored. Additionally, integrating techniques digital twin platforms could address real-time optimization challenges, dynamic behavior time-dependent consumption.
Language: Английский
Citations
0e-Prime - Advances in Electrical Engineering Electronics and Energy, Journal Year: 2024, Volume and Issue: 10, P. 100817 - 100817
Published: Oct. 20, 2024
Language: Английский
Citations
0