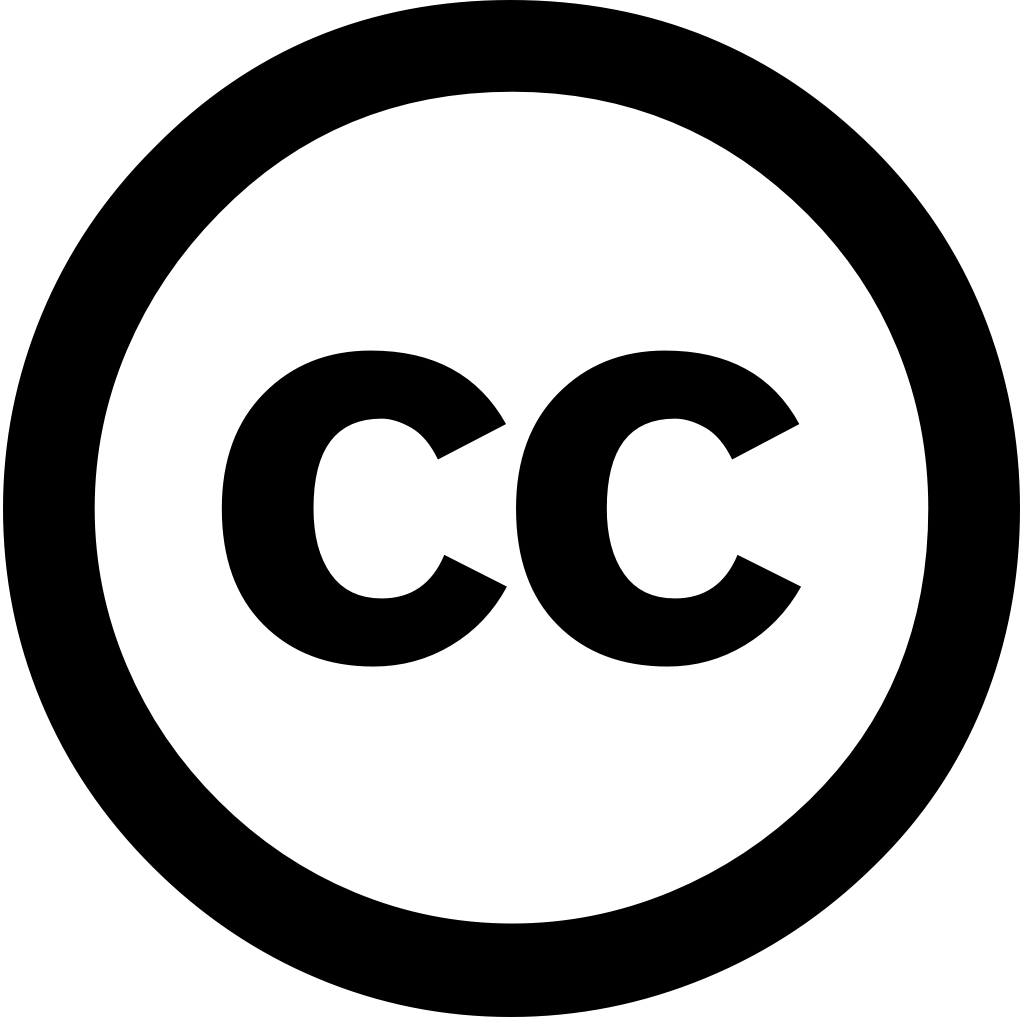
Natural Hazards Research, Journal Year: 2024, Volume and Issue: unknown
Published: Dec. 1, 2024
Language: Английский
Natural Hazards Research, Journal Year: 2024, Volume and Issue: unknown
Published: Dec. 1, 2024
Language: Английский
Natural Hazards, Journal Year: 2025, Volume and Issue: unknown
Published: March 11, 2025
Language: Английский
Citations
0The Science of The Total Environment, Journal Year: 2025, Volume and Issue: 976, P. 179311 - 179311
Published: April 9, 2025
Language: Английский
Citations
0Scientific Reports, Journal Year: 2025, Volume and Issue: 15(1)
Published: April 11, 2025
Language: Английский
Citations
0Journal of Environmental Management, Journal Year: 2025, Volume and Issue: 373, P. 123842 - 123842
Published: Jan. 1, 2025
Language: Английский
Citations
0Heliyon, Journal Year: 2024, Volume and Issue: 10(18), P. e37789 - e37789
Published: Sept. 1, 2024
Language: Английский
Citations
3Heliyon, Journal Year: 2024, Volume and Issue: 10(18), P. e38013 - e38013
Published: Sept. 1, 2024
Language: Английский
Citations
3Physics and Chemistry of the Earth Parts A/B/C, Journal Year: 2024, Volume and Issue: unknown, P. 103772 - 103772
Published: Oct. 1, 2024
Language: Английский
Citations
2Natural Hazards, Journal Year: 2024, Volume and Issue: unknown
Published: Nov. 4, 2024
Language: Английский
Citations
1Watershed Ecology and the Environment, Journal Year: 2024, Volume and Issue: unknown
Published: Sept. 1, 2024
Language: Английский
Citations
0Journal of Physics Conference Series, Journal Year: 2024, Volume and Issue: 2908(1), P. 012005 - 012005
Published: Nov. 1, 2024
Abstract Over 169 people along the Simangulampe upstream were under devastating flood and worst landslide watches in December 2023 due to a significant storm bringing heaviest rainfall moving giant boulders. Indeed, there are far fewer studies information on susceptibility hazards Simangalumpe than others. First-rate impressive risk mitigation strategies increased climate-change consideration reduced risk. We adopt C-band synthetic aperture radar multispectral imagery from Sentinel identify, visualize, analyze flash mapping mitigating address this issue. Precisely, is considered surface water indices with various parameters: Normalized Difference Vegetation Index (NDVI), Water (NDWI), Modified NDWI (MNDWI), SAR inundation mapping. Results show low NDVI values- over 50 percent of plant canopies damaged (uprooted broken trees) upstream. Combining properties index shows extent bodies Simagalumpe covers Finally, developing spatial temporal analysis data results flooding reducing unnecessary threats.
Language: Английский
Citations
0