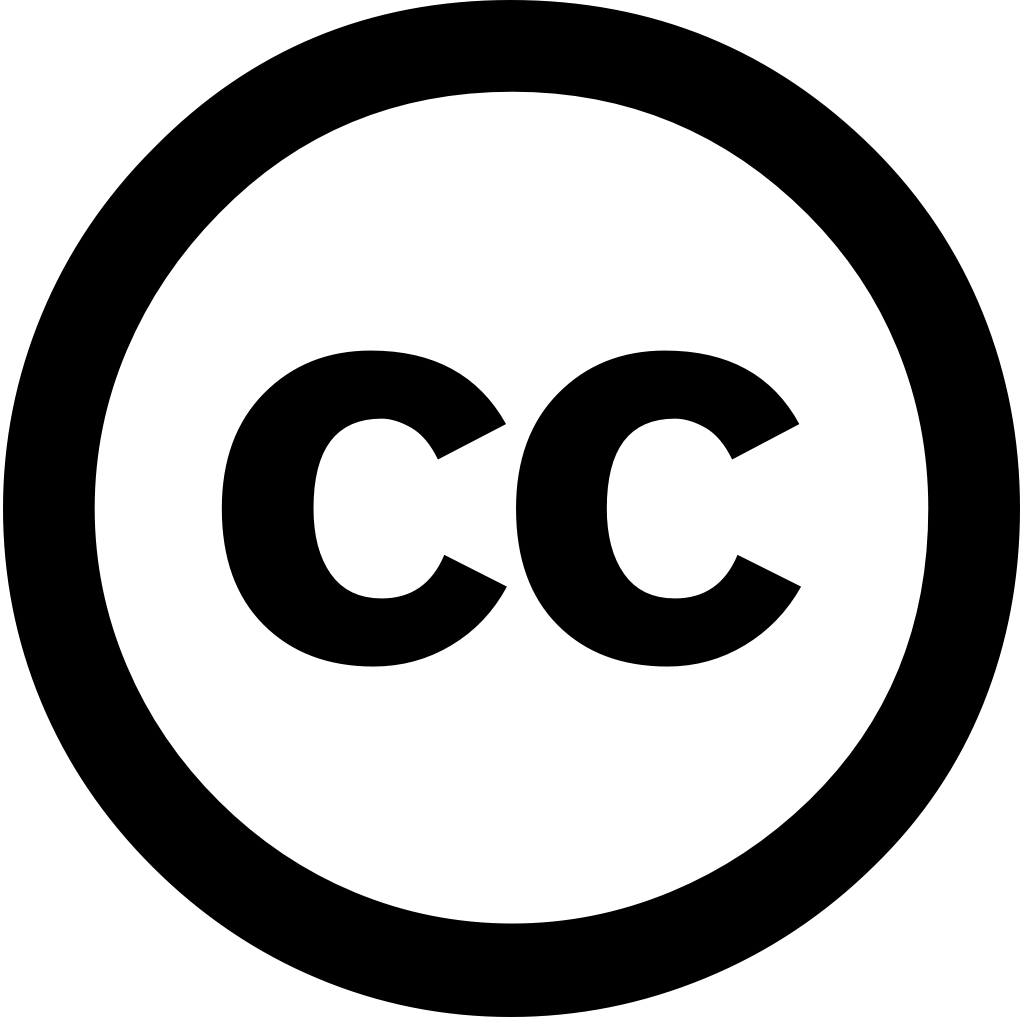
Frontiers in Immunology, Journal Year: 2025, Volume and Issue: 16
Published: May 8, 2025
Background The rapid development of COVID-19 vaccines highlighted the transformative potential artificial intelligence (AI) in modern vaccinology, accelerating timelines from years to months. Nevertheless, specific roles and effectiveness AI enhancing vaccine research, development, distribution, acceptance remain dispersed across various reviews, underscoring need for a unified synthesis. Methods We conducted an umbrella review consolidate evidence on AI’s contributions discovery, optimization, clinical testing, supply-chain logistics, public acceptance. Five databases were systematically searched up January 2025 systematic, scoping, narrative, as well meta-analyses explicitly focusing contexts. Quality assessments performed using ROBIS AMSTAR 2 tools evaluate risk bias methodological rigor. Results Among 27 traditional machine learning approaches—random forests, support vector machines, gradient boosting, logistic regression—dominated tasks antigen discovery epitope prediction supply‑chain optimization. Deep architectures, including convolutional recurrent neural networks, generative adversarial variational autoencoders, proved instrumental multiepitope design adaptive trial simulations. AI‑driven multi‑omic integration accelerated mapping, shrinking by months, while predictive analytics optimized manufacturing workflows operations (including temperature‑controlled, “cold‑chain” logistics). Sentiment analysis conversational demonstrated promising capabilities real‑time monitoring attitudes tailored communication address hesitancy. Nonetheless, persistent challenges emerged—data heterogeneity, algorithmic bias, limited regulatory frameworks, ethical concerns over transparency equity. Discussion implications These findings illustrate lifecycle but underscore that translating promise into practice demands five targeted action areas: robust data governance multi‑omics consortia harmonize share high‑quality datasets; comprehensive frameworks featuring transparent model explainability, standardized performance metrics, interdisciplinary ethics committees ongoing oversight; adoption designs simulations enable safety silico process modeling; AI‑enhanced engagement strategies—such routinely audited chatbots, sentiment dashboards, culturally messaging—to mitigate hesitancy; concerted focus global equity pandemic preparedness through capacity building, digital infrastructure expansion, routine audits, sustained funding low‑resource settings. Conclusion This confirms pivotal role efficacy safety, bolstering Realizing these benefits requires not only investments stakeholder also documentation, oversight, audits. Moreover, bridging gap real‑world impact large‑scale validation studies methods can accommodate heterogeneous evidence, ensuring innovations deliver equitable health outcomes reinforce preparedness.
Language: Английский