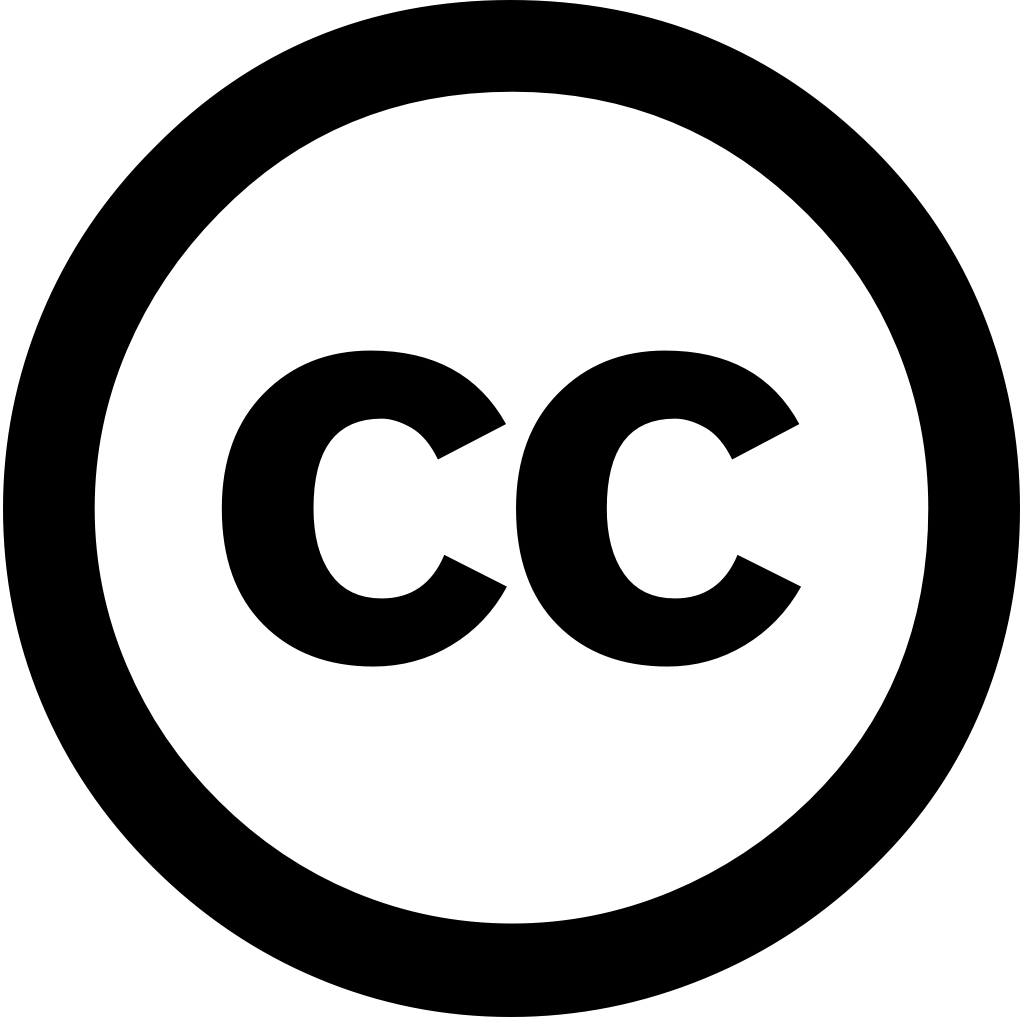
Annals of Data Science, Journal Year: 2025, Volume and Issue: unknown
Published: May 5, 2025
Language: Английский
Annals of Data Science, Journal Year: 2025, Volume and Issue: unknown
Published: May 5, 2025
Language: Английский
International Journal of Intelligent Computing and Cybernetics, Journal Year: 2025, Volume and Issue: unknown
Published: April 11, 2025
Purpose This study aims to enhance stock price prediction accuracy by integrating a gated recurrent unit (GRU) with an artificial rabbit optimization (ARO) algorithm. The objective is address the issues in hyperparameter and deliver high-performance predictive model for market trends tested on Dow Jones Industrial Average (DJIA) dataset. Design/methodology/approach proposed ARO-GRU hybrid uses GRU time-series ARO dynamically optimize model’s parameters. was benchmarked against various models, including single-layer multi-layer GRU, BiLSTM long short-term memory (LSTM) models optimized genetic algorithms (GA) or ARO. Performance assessed using metrics such as mean square error (MSE), absolute (MAE), percentage (MAPE) R -squared ( 2 ). Findings experimental results showed that significantly outperformed its counterparts. Compared best alternative (LSTM-ARO), reduced MSE 81.8% (from 22.731 1.864 AAPL stock) MAPE 64% 0.025 0.009). It achieved average score improvement of 5.3% across all stocks, demonstrating better fit. In addition, required 83% less computational time than LSTM-ARO model, further validating efficiency. Originality/value introduces integration algorithm prediction, marking novel combination efficiency optimization. By significant improvements computation time, this provides robust scalable solution dynamic stock-trading systems.
Language: Английский
Citations
0Annals of Data Science, Journal Year: 2025, Volume and Issue: unknown
Published: May 5, 2025
Language: Английский
Citations
0