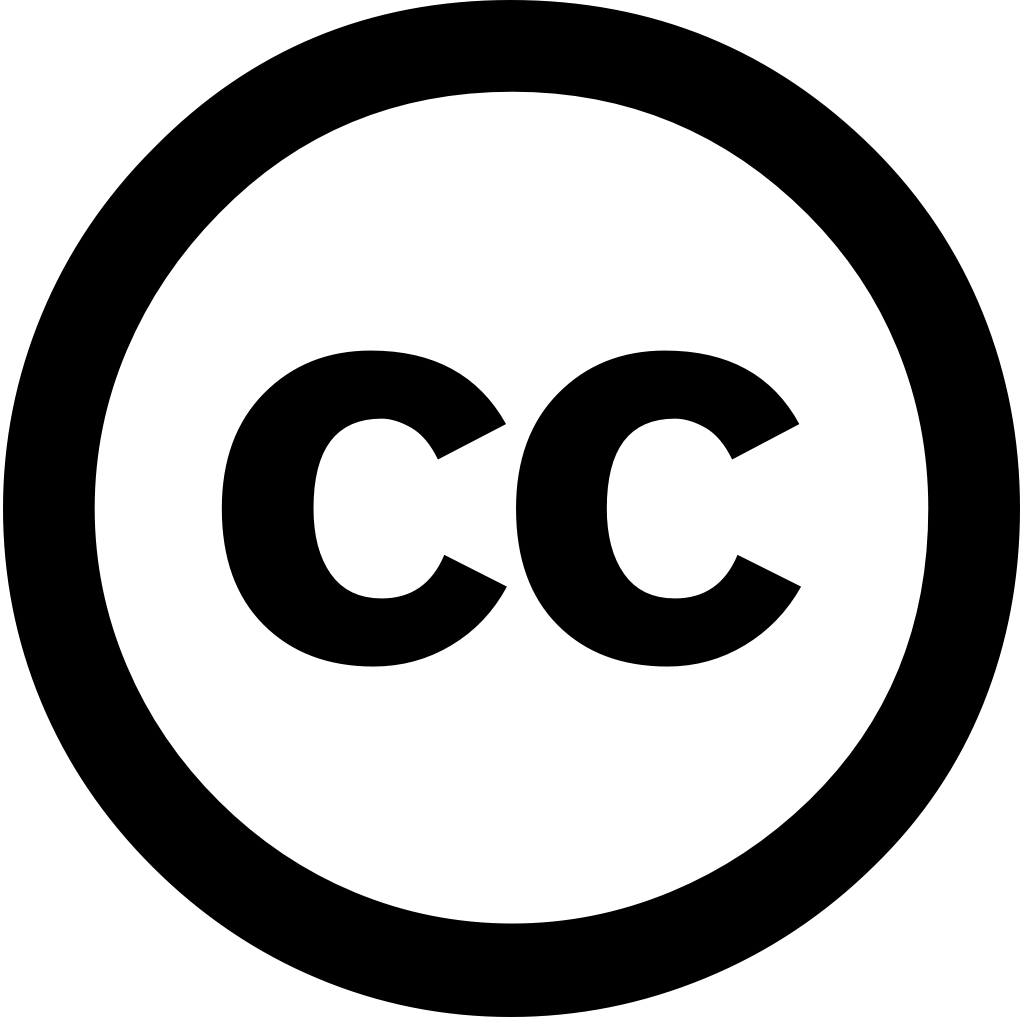
International Journal of Disaster Risk Reduction, Journal Year: 2025, Volume and Issue: unknown, P. 105441 - 105441
Published: March 1, 2025
Language: Английский
International Journal of Disaster Risk Reduction, Journal Year: 2025, Volume and Issue: unknown, P. 105441 - 105441
Published: March 1, 2025
Language: Английский
Technological Forecasting and Social Change, Journal Year: 2024, Volume and Issue: 202, P. 123325 - 123325
Published: March 11, 2024
Language: Английский
Citations
21Risks, Journal Year: 2024, Volume and Issue: 12(2), P. 19 - 19
Published: Jan. 23, 2024
This study examined the efficacy of artificial intelligence (AI) technologies in predictive risk assessment and their contribution to ensuring business continuity. research aimed understand how different AI components, such as natural language processing (NLP), AI-powered data analytics, AI-driven maintenance, integration incident response planning, enhance support continuity an environment where businesses face a myriad risks, including disasters, cyberattacks, economic fluctuations. A cross-sectional design quantitative method were used collect for this from sample 360 technology specialists. The results show that have major impact on assessment. Notably, it was discovered NLP improved accuracy speed procedures. into plans particularly effective, greatly decreasing company interruptions improving recovery unforeseen events. It is advised invest skills, fields automated assessment, analytics prompt detection, maintenance operational effectiveness, AI-enhanced planning crisis management.
Language: Английский
Citations
17International Journal of Disaster Risk Reduction, Journal Year: 2024, Volume and Issue: 110, P. 104629 - 104629
Published: June 24, 2024
Digital Twins (DT) is the real-time virtual representation of systems, communities, cities, or even human beings with substantial potential to revolutionize post-disaster risk management efforts and achieve resilient communities against adverse effects disasters. However, this remains largely unrecognized poorly understood in disaster management. This study explores current achievements, existing challenges, untapped DT management, accordingly, proposes an improved twin-based framework. paper employs a systematic literature review approach focusing on digital twinning (DPRMT) derived from two databases: Scopus Web Science. After screening process exclusion criteria, final analysis synthesizes findings selected set 96 papers. The results revealed that previous studies are not beyond only providing general statements about DT. There need for diverse data collection methods, considering demographic financial aspects, understanding social dynamics, employing dynamic models, recognizing interconnected giving due attention often-neglected recovery phase. comprehensive DPRMT concept framework leveraging decision-makers holistic efficient offers real-time, detailed, data-driven modeling solutions insights into disaster-affected areas communities. It also helpful optimize response planning, resource allocation, scenario testing by capturing complex behaviors systems entities often overlooked studies.
Language: Английский
Citations
16Nature Geoscience, Journal Year: 2025, Volume and Issue: unknown
Published: Feb. 5, 2025
Language: Английский
Citations
2Results in Engineering, Journal Year: 2025, Volume and Issue: unknown, P. 104349 - 104349
Published: Feb. 1, 2025
Language: Английский
Citations
2Nature Communications, Journal Year: 2025, Volume and Issue: 16(1)
Published: Feb. 24, 2025
In recent years, artificial intelligence (AI) has deeply impacted various fields, including Earth system sciences, by improving weather forecasting, model emulation, parameter estimation, and the prediction of extreme events. The latter comes with specific challenges, such as developing accurate predictors from noisy, heterogeneous, small sample sizes data limited annotations. This paper reviews how AI is being used to analyze climate events (like floods, droughts, wildfires, heatwaves), highlighting importance creating accurate, transparent, reliable models. We discuss hurdles dealing data, integrating real-time information, deploying understandable models, all crucial steps for gaining stakeholder trust meeting regulatory needs. provide an overview can help identify explain more effectively, disaster response communication. emphasize need collaboration across different fields create solutions that are practical, understandable, trustworthy enhance readiness risk reduction. Artificial Intelligence transforming study like helping overcome challenges integration. review article highlights models improve response, communication trust.
Language: Английский
Citations
2Advances in computational intelligence and robotics book series, Journal Year: 2024, Volume and Issue: unknown, P. 64 - 94
Published: Feb. 16, 2024
Global warming worsens natural disasters and humanitarian issues. Disaster prediction relies on satellites weather stations. AI may help catastrophe management. reduces disaster risk in many ways. Early warning systems, forecasts, recovery, reconstruction improve. could us predict, prepare, recover from calamities. These technologies provide climate change mitigation community protection hope. They propose a better future amid catastrophes. DRR is aggressively adopting AI, notably ML. This field encompasses severe event prediction, hazard mapping, real-time detection, situational awareness, decision assistance, more. Growing usage of management raises questions about its benefits. We face what issues? How can these difficulties be resolved opportunities maximised? What tell policymakers, stakeholders, the public to reduce disasters? The chapter introduces implementation issues, solutions make world more peaceful will addressed.
Language: Английский
Citations
12Water, Journal Year: 2025, Volume and Issue: 17(1), P. 85 - 85
Published: Jan. 1, 2025
Increasing numbers of emerging contaminants (ECs) detected in water environments require a detailed understanding these chemicals’ fate, distribution, transport, and risk aquatic ecosystems. Modeling is useful approach for determining ECs’ characteristics their behaviors environments. This article proposes systematic taxonomy EC models addresses gaps the comprehensive analysis applications. The reviewed include conventional quality models, multimedia fugacity machine learning (ML) models. Conventional have higher prediction accuracy spatial resolution; nevertheless, they are limited functionality can only be used to predict contaminant concentrations Fugacity excellent at depicting how travel between different environmental media, but cannot directly analyze variations parts same media because model assumes that constant within compartment. Compared other ML applied more scenarios, such as identification assessments, rather than being confined concentrations. In recent years, with rapid development artificial intelligence, surpassed becoming one newest hotspots study ECs. primary challenge faced by outcomes difficult interpret understand, this influences practical value an some extent.
Language: Английский
Citations
1Applied Sciences, Journal Year: 2025, Volume and Issue: 15(6), P. 3139 - 3139
Published: March 13, 2025
Sinkholes, naturally occurring formations in karst regions, represent a significant environmental hazard, threatening infrastructure, agricultural lands, and human safety. In recent years, machine learning (ML) techniques have been extensively employed for sinkhole susceptibility mapping (SSM). However, the lack of explainability inherent these methods remains critical issue decision-makers. this study, Konya Closed Basin was mapped using an interpretable model based on SHapley Additive exPlanations (SHAP). The Random Forest (RF), eXtreme Gradient Boosting (XGBoost), Light Machine (LightGBM) algorithms were employed, interpretability results enhanced through SHAP analysis. Among compared models, RF demonstrated highest performance, achieving accuracy 95.5% AUC score 98.8%, consequently selected development final map. analyses revealed that factors such as proximity to fault lines, mean annual precipitation, bicarbonate concentration difference are most variables influencing formation. Additionally, specific threshold values quantified, effects contributing analyzed detail. This study underscores importance employing eXplainable Artificial Intelligence (XAI) natural hazard modeling, SSM example, thereby providing decision-makers with more reliable comparable risk assessment.
Language: Английский
Citations
1Energies, Journal Year: 2025, Volume and Issue: 18(7), P. 1618 - 1618
Published: March 24, 2025
Traditional centralized energy grids struggle to meet urban areas’ increasingly complex demands, necessitating the development of more sustainable and resilient solutions. Smart microgrids offer a decentralized approach that enhances efficiency, facilitates integration renewable sources, improves resilience. This study follows systematic review approach, analyzing literature published in peer-reviewed journals, conference proceedings, industry reports between 2011 2025. The research draws from academic publications institutions alongside regulatory reports, examining actual smart microgrid deployments San Diego, Barcelona, Seoul. Additionally, this article provides real-world case studies New York London, showcasing successful unsuccessful deployments. Brooklyn Microgrid demonstrates peer-to-peer trading, while London faces regulations funding challenges its systems. paper also explores economic policy frameworks such as public–private partnerships (PPPs), localized markets, standardized models enable adoption at scale. While PPPs provide financial infrastructural support for deployment, they introduce stakeholder alignment compliance complexities. Countries like Germany India have successfully used development, leveraging low-interest loans, government incentives, mechanisms encourage innovation technologies. In addition, examines new trends utilization AI quantum computing optimize energy, climate design before outlining future agenda focused on cybersecurity, decarbonization, inclusion technology. Contributions include modular scalable framework, innovative hybrid storage systems, performance-based model suited environment. These contributions help fill gap what is possible today needed systems create foundation cities next century.
Language: Английский
Citations
1