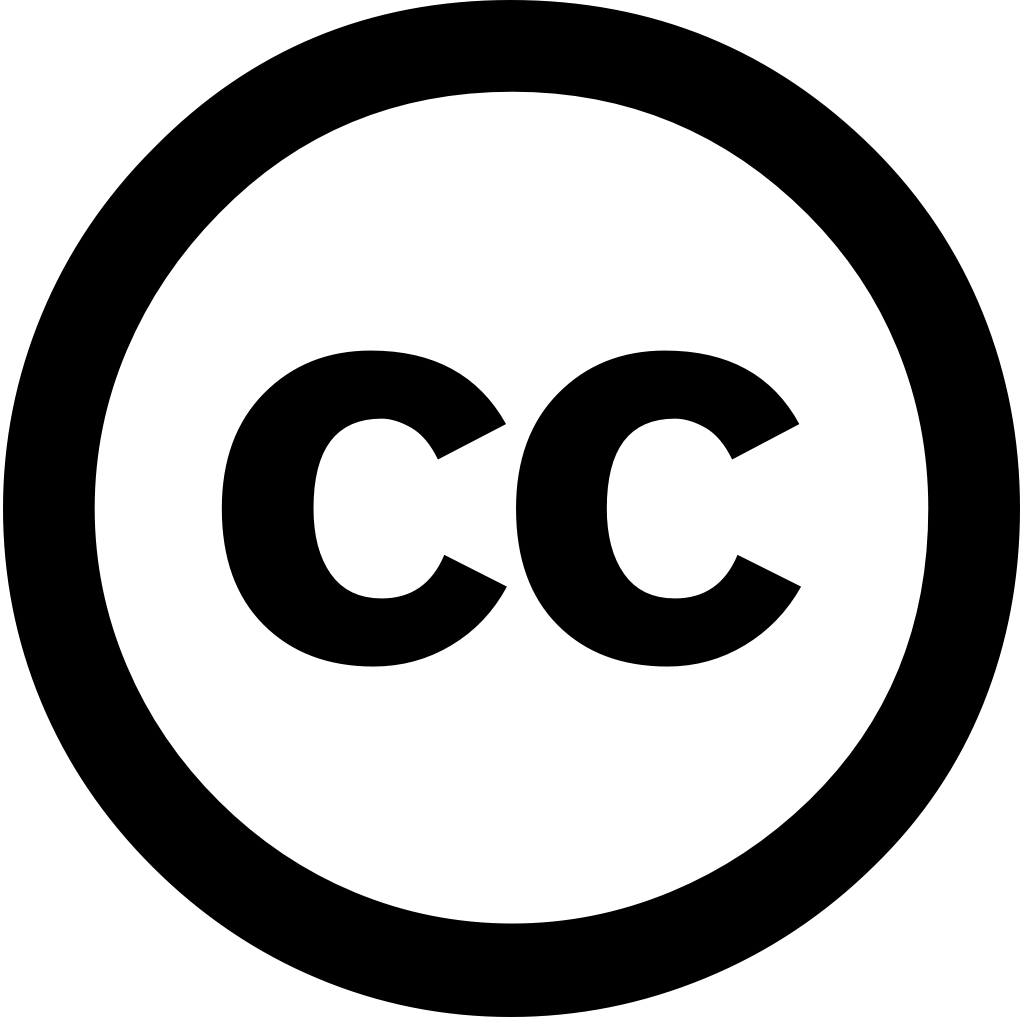
International Journal of Disaster Risk Reduction, Journal Year: 2025, Volume and Issue: unknown, P. 105441 - 105441
Published: March 1, 2025
Language: Английский
International Journal of Disaster Risk Reduction, Journal Year: 2025, Volume and Issue: unknown, P. 105441 - 105441
Published: March 1, 2025
Language: Английский
Remote Sensing, Journal Year: 2024, Volume and Issue: 16(15), P. 2842 - 2842
Published: Aug. 2, 2024
Wildfire susceptibility maps play a crucial role in preemptively identifying regions at risk of future fires and informing decisions related to wildfire management, thereby aiding mitigating the risks potential damage posed by wildfires. This study employs eXplainable Artificial Intelligence (XAI) techniques, particularly SHapley Additive exPlanations (SHAP), map Izmir Province, Türkiye. Incorporating fifteen conditioning factors spanning topography, climate, anthropogenic influences, vegetation characteristics, machine learning (ML) models (Random Forest, XGBoost, LightGBM) were used predict wildfire-prone areas using freely available active fire pixel data (MODIS Active Fire Collection 6 MCD14ML product). The evaluation trained ML showed that Random Forest (RF) model outperformed XGBoost LightGBM, achieving highest test accuracy (95.6%). All classifiers demonstrated strong predictive performance, but RF excelled sensitivity, specificity, precision, F-1 score, making it preferred for generating conducting SHAP analysis. Unlike prevailing approaches focusing solely on global feature importance, this fills critical gap employing summary dependence plots comprehensively assess each factor’s contribution, enhancing explainability reliability results. analysis reveals clear associations between such as wind speed, temperature, NDVI, slope, distance villages with increased susceptibility, while rainfall streams exhibit nuanced effects. spatial distribution classes highlights areas, flat coastal near settlements agricultural lands, emphasizing need enhanced awareness preventive measures. These insights inform targeted management strategies, highlighting importance tailored interventions like firebreaks management. However, challenges remain, including ensuring selected factors’ adequacy across diverse regions, addressing biases from resampling spatially varied data, refining broader applicability.
Language: Английский
Citations
7Sustainability, Journal Year: 2024, Volume and Issue: 16(8), P. 3478 - 3478
Published: April 22, 2024
Soil quality is vital for ecosystem stability, impacting human, plant, and animal health. Traditional soil assessments are labor-intensive costly, making them unsuitable smart agriculture. To overcome this, Internet of Things (IoT) artificial intelligence (AI) technologies employed sustainable agriculture, enabling real-time data collection analysis, trend identification, health optimization. The Western Greece Information System (WΕSIS) offers open-access services sustainability. It includes modules indicators, fertilization management zones, property distribution, prediction, mapping, statistical water management, land use maps, digital crop calculation. Integrating the IoT AI allows remote monitoring conditions, managing interventions adaptively in a data-driven way, enhancing resources’ efficiency sustainability, increasing yield quality. algorithms assist farmers regional stakeholders optimizing production lines, methodologies, field practices, reducing costs profitability. This promotes circular economy, soil- climate-resilient future, biodiversity protection targets, enhanced fertility productivity. proposed IoT/AI technical architecture can underpin development platforms, integrating from various sources, automating collection, providing decision support tools.
Language: Английский
Citations
6International Journal of Disaster Risk Reduction, Journal Year: 2024, Volume and Issue: 107, P. 104468 - 104468
Published: April 16, 2024
Language: Английский
Citations
5International Journal of Disaster Risk Reduction, Journal Year: 2024, Volume and Issue: 114, P. 104723 - 104723
Published: Aug. 6, 2024
Despite the rising global flood risk, impacts of flooding remain systematically underestimated, leading to significant consequences for particularly vulnerable river deltas. Most studies focus either on single hazards or social vulnerability while overlooking interconnected dynamics deltaic social-ecological systems. In response first priority Sendai Framework, which calls an understanding disaster risk in all its dimensions, we apply Global Delta Risk Index Ayeyarwady Myanmar. We combine 55 indicators social- and ecosystem with 100-year, 500-year, 1,000-year scenarios pluvial, fluvial, coastal exposure at sub-delta scale. Using townships as units analysis allows bridging gap between local case studies, providing insights that are meaningful risk-informed development delta a whole system. also examine distinctive characteristics define systems prone flooding. Our results reveal patterns drivers affect least 65 % delta's population 60 ecosystem, self-reinforcing dynamics, but those contribute mutual resilience both argue principles integrated management should be applied leverage scarce resources simultaneously reduce secure livelihoods preserve services.
Language: Английский
Citations
5Intelligent Systems with Applications, Journal Year: 2024, Volume and Issue: 23, P. 200430 - 200430
Published: Aug. 28, 2024
Language: Английский
Citations
4Advances in environmental engineering and green technologies book series, Journal Year: 2025, Volume and Issue: unknown, P. 73 - 114
Published: Jan. 24, 2025
This chapter examines using artificial intelligence (AI) and deep learning (DL) in disaster management. It describes a paradigm shift towards proactive measures preventing managing natural disasters. Traditional, reactive methods often reach their limits. At the same time, AI-based approaches can improve early warning systems allocate resources more efficiently through analysis of large, heterogeneous data sets ability to recognize complex patterns. The article highlights application DL models, such as Convolutional Neural Networks (CNNs), analyze satellite imagery utility response. Both technical ethical challenges are discussed, particularly protection, bias, transparency models. Finally, framework is presented that provides guidelines for effective responsible use AI management promotes long-term sustainability fairness this area.
Language: Английский
Citations
0Advances in environmental engineering and green technologies book series, Journal Year: 2025, Volume and Issue: unknown, P. 115 - 144
Published: Jan. 24, 2025
Wildfires increasingly threaten Portugal's ecosystem and population, with climate change intensifying fire seasons. AI has emerged as a powerful tool for predicting responding to wildfires, offering improved accuracy speed over traditional methods. This paper examines AI's role in wildfire management Portugal, focusing on machine learning, image recognition, real-time data analytics, IoT integration. It addresses potential enhance emergency responses, reduce environmental damage, optimize firefighting resources. Case studies, including the Bee2FireDetection system sensor networks, highlight impact, concluding strategic recommendations future AI-driven mitigation Portugal.
Language: Английский
Citations
0Natural Hazards, Journal Year: 2025, Volume and Issue: unknown
Published: Feb. 16, 2025
Language: Английский
Citations
0Climate change management, Journal Year: 2025, Volume and Issue: unknown, P. 99 - 114
Published: Jan. 1, 2025
Language: Английский
Citations
0Journal of Technology in Human Services, Journal Year: 2025, Volume and Issue: unknown, P. 1 - 25
Published: Feb. 27, 2025
In an era of increasingly frequent and severe natural disasters, the need for effective disaster support is critical. AI technologies offer a transformative approach to support. This study explores innovative use in providing psychosocial disasters. Employing semi-systematic literature analysis, four-step process was used evaluate select 18 relevant articles based on inclusion exclusion criteria. These were analyzed their focus AI's role support, including types used, phases management, implementation challenges. The research highlights application via social media platforms other tools, identifies discusses challenges implementation. findings suggest incorporating AI-based training tools into curriculum workers mental health professionals. At policy level, this underscores governments response agencies integrate AI-driven systems management strategies. practice, implementing AI-powered can significantly enhance capacity relief organizations provide timely targeted assistance, ultimately improving well-being resilience disaster-affected communities.
Language: Английский
Citations
0