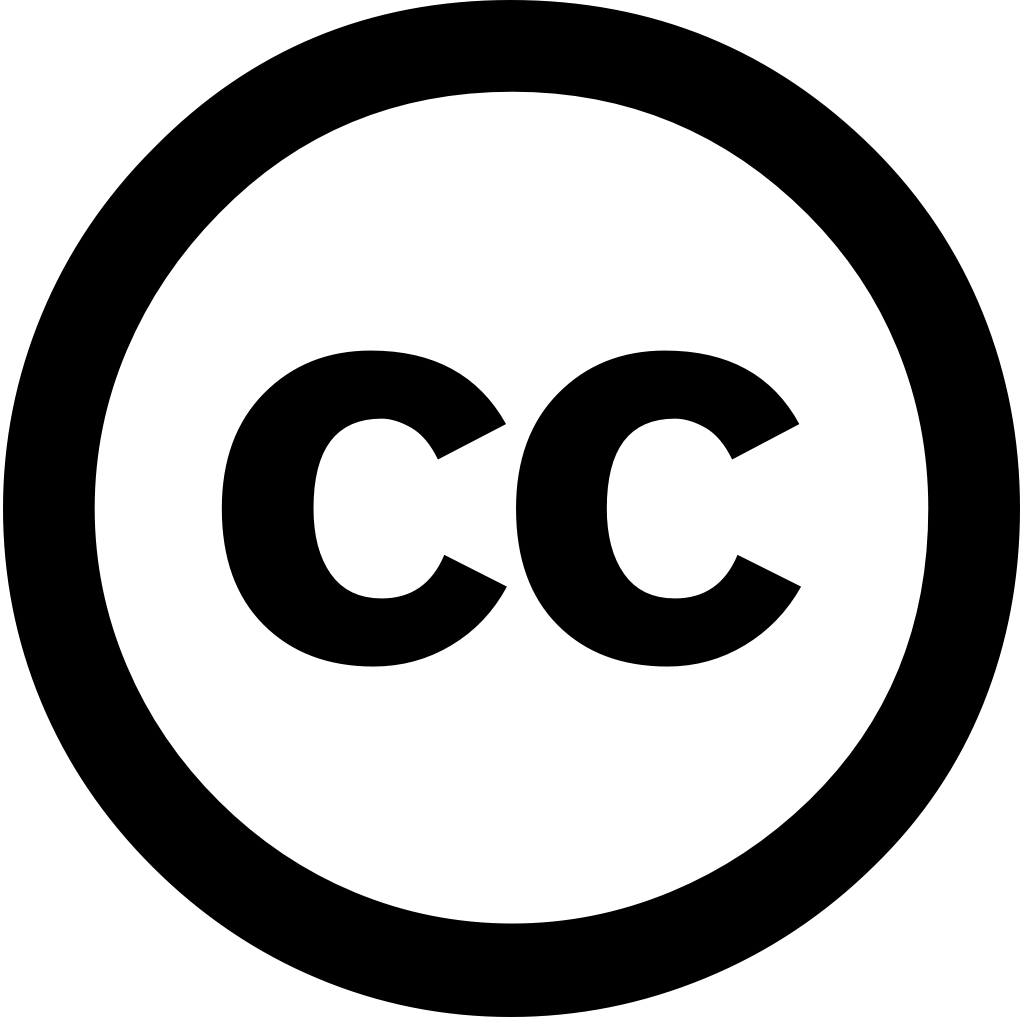
Scientific Reports, Journal Year: 2024, Volume and Issue: 14(1)
Published: Sept. 4, 2024
Language: Английский
Scientific Reports, Journal Year: 2024, Volume and Issue: 14(1)
Published: Sept. 4, 2024
Language: Английский
Neural Computing and Applications, Journal Year: 2024, Volume and Issue: 36(18), P. 10613 - 10635
Published: March 27, 2024
Abstract This article proposes the use of a leader white shark optimizer (LWSO) with aim improving exploitation conventional (WSO) and solving economic operation-based load dispatch (ELD) problem. The ELD problem is crucial aspect power system operation, involving allocation generation resources to meet demand while minimizing operational costs. proposed approach aims enhance performance efficiency WSO by introducing leadership mechanism within optimization process, which aids in more effectively navigating complex solution space. LWSO achieves increased utilizing leader-based mutation selection throughout each sharks. efficacy algorithm tested on 13 engineer benchmarks non-convex problems from CEC 2020 compared recent metaheuristic algorithms such as dung beetle (DBO), WSO, fox (FOX), moth-flame (MFO) algorithms. also used address different case studies (6 units, 10 11 40 units), 20 separate runs using other competitive being statistically assessed demonstrate its effectiveness. results show that outperforms algorithms, achieving best for minimum fuel cost Additionally, statistical tests are conducted validate competitiveness algorithm.
Language: Английский
Citations
14Scientific Reports, Journal Year: 2024, Volume and Issue: 14(1)
Published: April 1, 2024
Abstract This study presents an advanced metaheuristic approach termed the Enhanced Gorilla Troops Optimizer (EGTO), which builds upon Marine Predators Algorithm (MPA) to enhance search capabilities of (GTO). Like numerous other algorithms, GTO encounters difficulties in preserving convergence accuracy and stability, notably when tackling intricate adaptable optimization problems, especially compared more techniques. Addressing these challenges aiming for improved performance, this paper proposes EGTO, integrating high low-velocity ratios inspired by MPA. The EGTO technique effectively balances exploration exploitation phases, achieving impressive results utilizing fewer parameters operations. Evaluation on a diverse array benchmark functions, comprising 23 established functions ten complex ones from CEC2019 benchmark, highlights its performance. Comparative analysis against techniques reveals EGTO's superiority, consistently outperforming counterparts such as tuna swarm optimization, grey wolf optimizer, gradient based artificial rabbits algorithm, pelican Runge Kutta algorithm (RUN), original algorithms across various test functions. Furthermore, efficacy extends addressing seven challenging engineering design encompassing three-bar truss design, compression spring pressure vessel cantilever beam welded speed reducer gear train design. showcase robust rate, adeptness locating local/global optima, supremacy over alternative methodologies explored.
Language: Английский
Citations
6Computers & Electrical Engineering, Journal Year: 2024, Volume and Issue: 117, P. 109279 - 109279
Published: May 9, 2024
Language: Английский
Citations
5International Journal of Electrical Power & Energy Systems, Journal Year: 2024, Volume and Issue: 161, P. 110200 - 110200
Published: Aug. 28, 2024
Language: Английский
Citations
4Energy, Journal Year: 2025, Volume and Issue: 316, P. 134483 - 134483
Published: Jan. 13, 2025
Language: Английский
Citations
0Electric Power Systems Research, Journal Year: 2025, Volume and Issue: 242, P. 111454 - 111454
Published: Jan. 31, 2025
Language: Английский
Citations
0Computers & Electrical Engineering, Journal Year: 2025, Volume and Issue: 123, P. 110149 - 110149
Published: Feb. 21, 2025
Language: Английский
Citations
0Energy Informatics, Journal Year: 2025, Volume and Issue: 8(1)
Published: March 18, 2025
Language: Английский
Citations
0Applied Soft Computing, Journal Year: 2025, Volume and Issue: unknown, P. 113039 - 113039
Published: March 1, 2025
Language: Английский
Citations
0Mathematics, Journal Year: 2025, Volume and Issue: 13(7), P. 1042 - 1042
Published: March 23, 2025
The Static Economic Load Dispatch (SELD) problem is a paramount optimization challenge in power engineering that seeks to optimize the allocation of between generating units meet imposed constraints while minimizing energy requirements. Recently, researchers have employed numerous meta-heuristic approaches tackle this challenging, non-convex problem. This work introduces an innovative algorithm, named “Attaining and Refining Knowledge-based Optimization (ARKO)”, which uses ability humans learn from their surroundings by leveraging collective knowledge population. ARKO algorithm consists two distinct phases: attaining refining. In phase, gathers population’s top candidates, refining phase enhances performance other selected candidates. way learning improving with help candidates provides robust exploration exploitation capability for algorithm. To validate efficacy ARKO, we conduct comprehensive evaluation against eleven established algorithms using diverse set 41 test functions CEC-2017 CEC-2022 suites, then, three real-life applications also verify its practical ability. Subsequently, implement SELD considering several instances. examination numerical statistical results confirms remarkable efficiency potential complex tasks.
Language: Английский
Citations
0