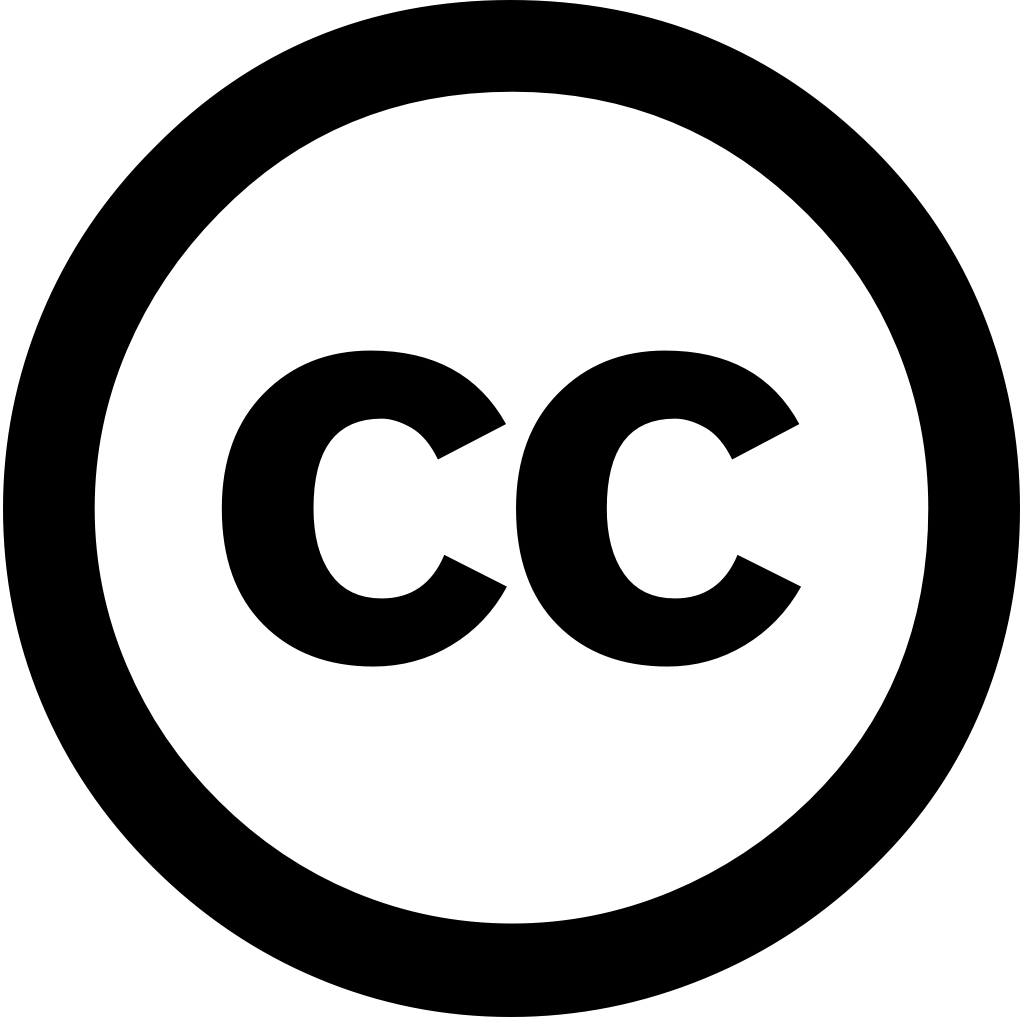
Sustainable Energy Grids and Networks, Journal Year: 2024, Volume and Issue: unknown, P. 101604 - 101604
Published: Dec. 1, 2024
Language: Английский
Sustainable Energy Grids and Networks, Journal Year: 2024, Volume and Issue: unknown, P. 101604 - 101604
Published: Dec. 1, 2024
Language: Английский
International Journal of Electrical Power & Energy Systems, Journal Year: 2025, Volume and Issue: 165, P. 110490 - 110490
Published: Feb. 3, 2025
Language: Английский
Citations
1Journal of King Saud University - Computer and Information Sciences, Journal Year: 2025, Volume and Issue: 37(3)
Published: April 18, 2025
Language: Английский
Citations
0Electronics, Journal Year: 2024, Volume and Issue: 13(24), P. 4988 - 4988
Published: Dec. 18, 2024
The strong development of distributed energy sources has become one the most important measures for low-carbon worldwide. With a significant quantity photovoltaic (PV) power generation being integrated to grid, accurate and efficient prediction PV is an essential guarantee security stability electricity grid. Due shortage data from stations influence weather, it difficult obtain satisfactory performance prediction. In this regard, we present forecasting model based on Fourier graph neural network (FourierGNN). Firstly, hypervariable constructed by considering weather neighbouring plants as nodes, respectively. hypervariance then transformed in space capture spatio-temporal dependence among nodes via discrete transform. multilayer operator (FGO) can be further exploited information. Experiments carried out at six show that presented approach enables optimal obtained adequately exploiting
Language: Английский
Citations
1Published: Jan. 1, 2024
Language: Английский
Citations
0Energies, Journal Year: 2024, Volume and Issue: 17(23), P. 5848 - 5848
Published: Nov. 22, 2024
With the rapid development of renewable energy, accurately forecasting wind power is crucial for stable operation systems and effective energy management. This paper proposes a short-term method based on Orthogonalized Maximal Information Coefficient (OMNIC) combined with an Adaptive fractional Generalized Pareto motion (fGPm) model. The quantifies influence meteorological factors prediction identifies optimal set number influencing factors. model accounts long-range dependence (LRD) in time series data constructs uncertainty using properties parameters generalized distribution (GPD), significantly improving accuracy under nonlinear conditions. proposed approach was validated real dataset from farm northwest China compared other models such as Convolutional Neural Network-Long Short-Term Memory (CNN-LSTM) Network-Gated Recurrent Unit (CNN-GRU). Results show that adaptive fGPm reduces RMSE by 0.448 MW 0.466 MW, MAPE 6.936% 9.702%, achieves average R2 0.9826 to CNN-GRU CNN-LSTM. improvement due dynamic adjustment trends use LRD features. provides practical value addressing grid integration regulation challenges.
Language: Английский
Citations
0Sustainable Energy Grids and Networks, Journal Year: 2024, Volume and Issue: unknown, P. 101604 - 101604
Published: Dec. 1, 2024
Language: Английский
Citations
0