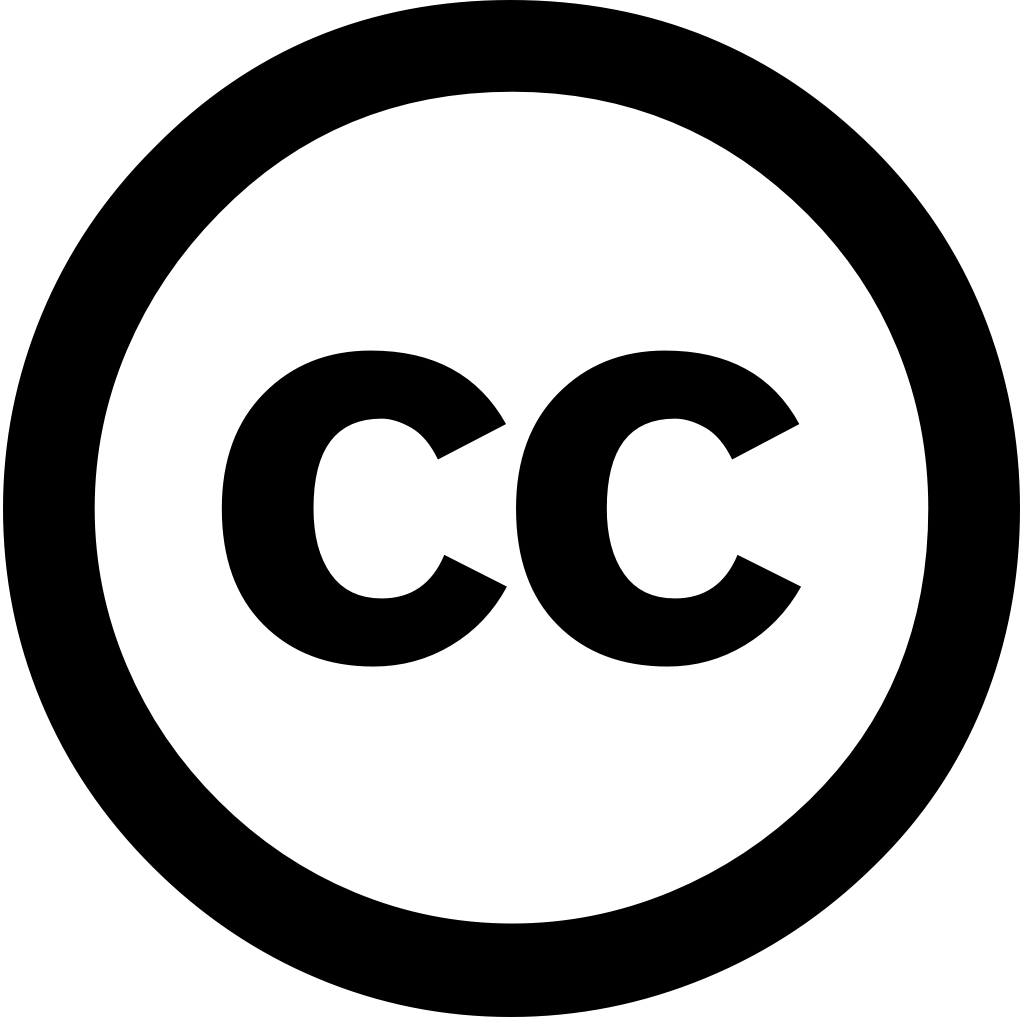
Scientific Reports, Journal Year: 2025, Volume and Issue: 15(1)
Published: March 4, 2025
Transformers are important equipment in the power system and their reliable safe operation is an guarantee for high-efficiency of system. In order to achieve prognostics health management transformer, a novel intelligent fault diagnosis transformer based on multi-source data fusion correlation analysis proposed. Firstly, multiple components dissolved gases performed by improved entropy weighting method. Then, combination bidirectional long short-term memory network, attention mechanism, convolution neural network employed predict load rate, upper oil temperature, winding temperature data, indices gas transformer. Furthermore, Apriori rate layer, support confidence levels predictive assessment state. Finally, validity algorithm verified applying actual from monitoring platform. The results show that vicinity sample point 88, gas, not within normal range intervals, it presumed arc discharge phenomenon. average correct 100 diagnoses model proposed this paper 0.917, mean square error 0.018. can prediction accident early warning, prevent further expansion accident.
Language: Английский