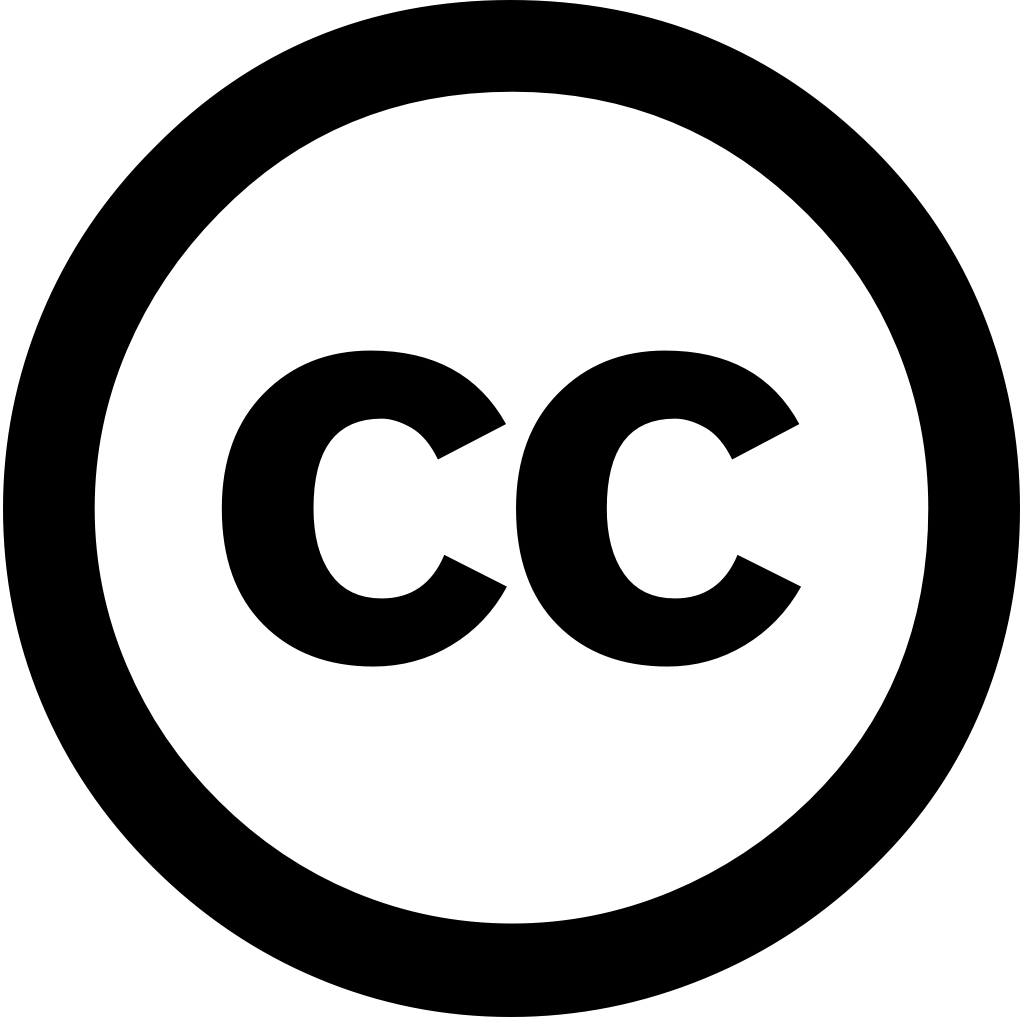
Geosciences, Journal Year: 2025, Volume and Issue: 15(5), P. 183 - 183
Published: May 19, 2025
Proper geologic reservoir characterization is crucial for energy generation and climate change mitigation efforts. While conventional techniques like core analysis well logs provide limited spatial information, seismic data can offer valuable 3D insights into fluid rock properties away from the well. This research focuses on identifying important structural stratigraphic variations at Mississippi Canyon Block 118 (MC-118) field, located northern slope of Gulf Mexico, which significantly influenced by complex salt tectonics failure. Due to a lack direct subsurface cores, this area poses challenges in delineating potential reservoirs carbon storage. The study leveraged multi-attribute machine learning 3-D improve characterization, could inform field development strategies hydrogen or Different combinations geometric, instantaneous, amplitude-based, spectral frequency, textural attributes were tested using Self-Organizing Maps (SOM) identify distinct facies. SOM Models 1 2, combined spectral, amplitude-based attributes, shown delineate storage reservoirs, gas hydrates, structures, associated radial faults, areas with poor quality due presence structures more than 3 4. results presented evidence validated matching sands log information identified facies SOM. By automating integration property prediction, proposed workflow leads cost-effective faster understanding traditional interpretation methods. Additionally, approach may apply other locations sparse interest.
Language: Английский