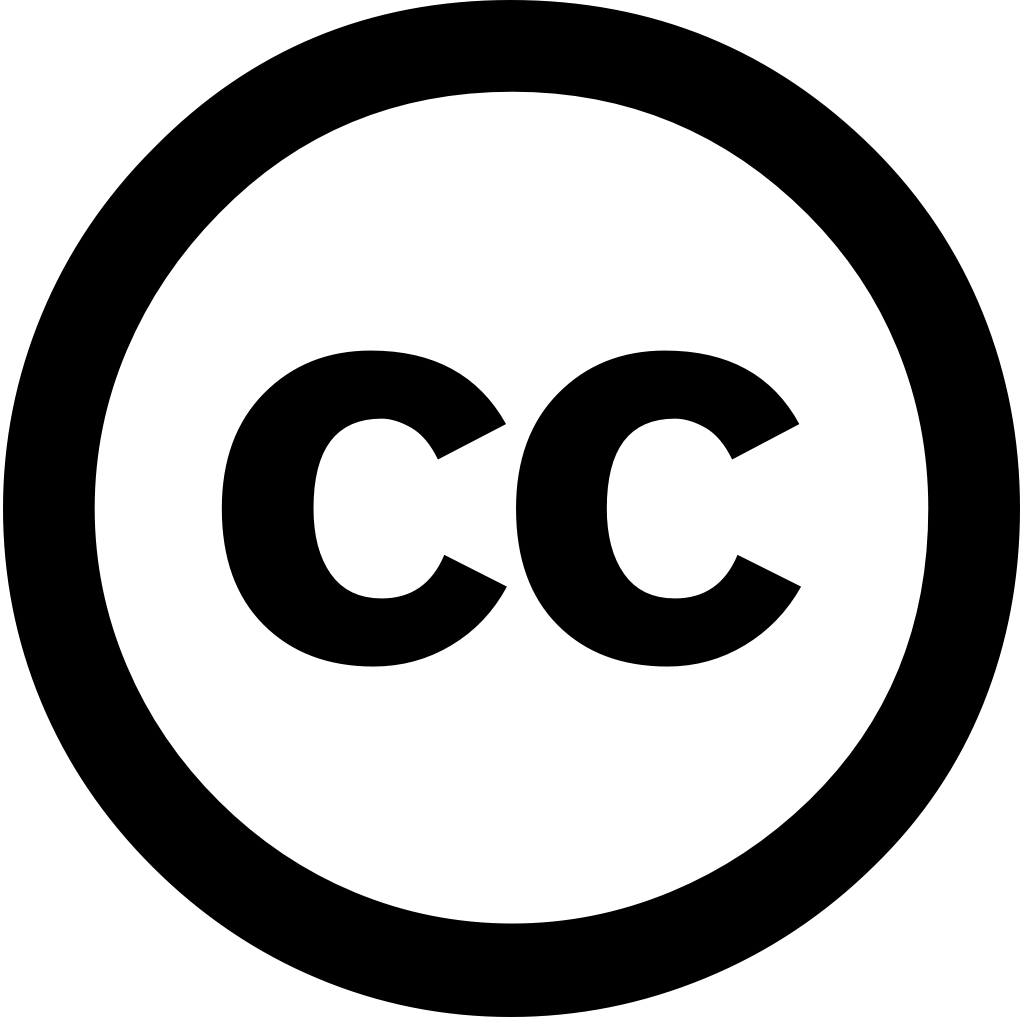
Heliyon, Journal Year: 2024, Volume and Issue: 10(20), P. e39205 - e39205
Published: Oct. 1, 2024
Language: Английский
Heliyon, Journal Year: 2024, Volume and Issue: 10(20), P. e39205 - e39205
Published: Oct. 1, 2024
Language: Английский
Sensors, Journal Year: 2025, Volume and Issue: 25(4), P. 1166 - 1166
Published: Feb. 14, 2025
Polycystic ovary syndrome (PCOS) is a medical condition that impacts millions of women worldwide; however, due to lack public awareness, as well the expensive testing involved in identification PCOS, 70% cases go undiagnosed. Therefore, primary objective this study design an expert machine learning (ML) model for early diagnosis PCOS based on initial symptoms and health indicators; two datasets were amalgamated preprocessed accomplish goal, resulting new symptomatic dataset with 12 attributes. An ensemble (EL) model, seven base classifiers, deep (DL) meta-level classifier, are proposed. The hyperparameters EL optimized through nature-inspired walrus optimization (WaO), cuckoo search (CSO), random (RSO) algorithms, leading WaOEL, CSOEL, RSOEL models, respectively. results obtained prove supremacy designed WaOEL over other prediction accuracy 92.8% area under receiver operating characteristic curve (AUC) 0.93; moreover, feature importance analysis, presented forest (RF) Shapley additive values (SHAP) positive predictions, highlights crucial clinical insights need intervention. Our findings suggest patients features related obesity high cholesterol more likely be diagnosed positive. Most importantly, it inferred from without tests possible proposed which helps clinicians make better informed decisions, identify comorbidities, reduce harmful long-term effects PCOS.
Language: Английский
Citations
0Engineering Reports, Journal Year: 2025, Volume and Issue: 7(2)
Published: Feb. 1, 2025
ABSTRACT PCOS (polycystic ovary syndrome) is a common hormonal disorder that affects many women during their reproductive years. It marked by imbalances, leading to ovarian cysts, and can result in health issues such as infertility, diabetes, even heart problems. Diagnosing accurately early be challenging, it requires specific medical expertise. However, spotting promptly allows individuals follow recommendations, which lead healthier lifestyles. In this study, we examined dataset consisting of 541 patient records enhance the detection using advanced machine learning techniques. We established data preprocessing pipeline rigorously addressed missing values identified outliers, while also normalizing ensure was ready for input. For feature selection, applied techniques SelectKBest, Chi‐Square, XGBoost. These methods helped us pinpoint most predictive attributes, improved interpretability efficiency our models. Hyperparameter tuning carefully performed through grid search cross‐validation, ensuring each model optimized best prediction accuracy. Importantly, research highlights how effective predicting PCOS. The logistic regression support vector stood out with its remarkable accuracy 99.7753%. Furthermore, created user‐friendly web application facilitate smooth deployment real‐time analysis. This provides healthcare professionals handy tool identifying risks related features an intuitive interface where users easily input clinical information receive immediate risk assessments.
Language: Английский
Citations
0Scientific Reports, Journal Year: 2025, Volume and Issue: 15(1)
Published: Feb. 18, 2025
A complex biological process involves physical changes and hormonal fluctuation in the menstrual cycle. The traditional nutrition recommendation models often offer general guidelines but fail to address specific requirements of women during various cycle stages. This paper proposes a novel Optimization Hybrid Deep Learning (OdriHDL) model provide personalized health for their It pre-processing data through Missing Value Imputation, Z-score Normalization, One-hot encoding. Next, feature extraction is accomplished using Layered Sparse Autoencoder Network. Then, extracted features are utilized by Attention-based Bidirectional Convolutional Greylag Goose Gated Recurrent Network (HABi-ConGRNet) nutrient recommendation. hyper-parameter tuning HABi-ConGRNet carried out Algorithm enhance performance. Python platform used simulation collected data, several performance metrics employed analyze OdriHDL demonstrates superior performance, achieving maximum accuracy 97.52% enhanced precision rate contrast existing methods, like RNN, CNN-LSTM, attention GRU. findings suggest that captures patterns between nutritional needs symptoms provides robust solutions unique physiological experienced women.
Language: Английский
Citations
0PeerJ Computer Science, Journal Year: 2025, Volume and Issue: 11, P. e2702 - e2702
Published: Feb. 28, 2025
In the modern era of digitalization, integration with blockchain and machine learning (ML) technologies is most important for improving applications in healthcare management secure prediction analysis health data. This research aims to develop a novel methodology securely storing patient medical data analyzing it PCOS prediction. The main goals are leverage Hyperledger Fabric immutable, private integrate Explainable Artificial Intelligence (XAI) techniques enhance transparency decision-making. innovation this study unique technology ML XAI, solving critical issues security model interpretability healthcare. With Caliper tool, blockchain’s performance evaluated enhanced. suggested AI-based system Polycystic Ovary Syndrome detection (EAIBS-PCOS) demonstrates outstanding records 98% accuracy, 100% precision, 98.04% recall, resultant F1-score 99.01%. Such quantitative measures ensure success proposed delivering dependable intelligible predictions diagnosis, therefore making great addition literature while serving as solid solution near future.
Language: Английский
Citations
0Intelligence-Based Medicine, Journal Year: 2025, Volume and Issue: unknown, P. 100253 - 100253
Published: April 1, 2025
Language: Английский
Citations
0ENVIRONMENTAL SYSTEMS RESEARCH, Journal Year: 2024, Volume and Issue: 13(1)
Published: Oct. 9, 2024
Air is one of the most significant elements environment. The increasing global air pollution crisis poses an unavoidable threat to human health, environmental sustainability, ecosystems, and earth's climate. has been referred as a silent killer due its insidious nature. Its indirect impact on health further underscores dangerous effects. Early detection quality can potentially save millions lives globally. A unique transformative approach harness power machine learning combat pollution. This research presents manual web-based automatic prediction system that provides real-time alerts status help prevent premature deaths, chronic diseases, other problems. pollutants, including carbon monoxide (CO), ozone (O3), nitrogen dioxide (NO2), particulate matter (PM 2.5), are used in this study for feature analysis extraction. utilizes publicly available data from 23,463 different cities worldwide. Data preprocessing was performed before feeding into models correlation evaluation. proposed uses various predict quality, Random Forest (100%), Logistic Regression (79%), Decision Tree Support Vector Machine (93%), Linear SVC (98%), K-Nearest Neighbor (99%), Multinomial Naïve Bayes (52%). user-friendly Django-based web interface offers accessible platform users monitor real-time, based two best-performing models: techniques.
Language: Английский
Citations
3Deleted Journal, Journal Year: 2024, Volume and Issue: unknown
Published: June 25, 2024
Cancer remains the leading cause of death worldwide, significantly impacting individuals and healthcare systems alike. In recent decades, skin cancer has surged in prevalence compared to other major types. Various factors such as texture, color, morphological characteristics, structure are employed categorizing different forms cancer. However, traditional methods identification often prove time-consuming costly. Skin classification predominantly relies on machine learning, with primary method being convolutional neural networks (CNNs). Our 'SkinMultiNet' framework, presented this study based transfer learning principles, integrates InceptionV3 Xception CNN models for predicting using image data. While ResNet50, NasNet, MobileNet were explored, framework demonstrated most promising outcomes. Utilizing a publicly available dataset comprising 6086 images, we trained, tested, evaluated our extensively. Proposed system train generator feed data into deep models, followed by implementing rate reducer datasets within model. Through rigorous testing validation procedures, successfully processed substantial volume contrast conventional approaches, proposed architecture offers potential more reliable diagnoses, achieving an optimal accuracy 94% prediction. This advancement holds promise early detection improved patient outcomes following therapy.
Language: Английский
Citations
2International Journal of Information Technology, Journal Year: 2024, Volume and Issue: unknown
Published: Sept. 4, 2024
Language: Английский
Citations
2Crop Design, Journal Year: 2024, Volume and Issue: 3(4), P. 100079 - 100079
Published: Sept. 10, 2024
Language: Английский
Citations
0Journal of The Institution of Engineers (India) Series B, Journal Year: 2024, Volume and Issue: unknown
Published: Oct. 9, 2024
Language: Английский
Citations
0